


In recent years, reinforcement learning in self-game has achieved superhuman performance in a series of games such as Go and chess. Furthermore, the idealized version of self-play also converges to a Nash equilibrium. Nash equilibrium is very famous in game theory. This theory was proposed by John Nash, the founder of game theory and Nobel Prize winner. That is, in a game process, regardless of the other party's strategy choice, one party will choose a certain strategy. strategy, the strategy is called a dominant strategy. If any player chooses the optimal strategy when the strategies of all other players are determined, then this combination is defined as a Nash equilibrium.
Previous research has shown that the seemingly effective continuous control strategies in self-game can also be exploited by countermeasures, suggesting that self-game may not be as powerful as previously thought. This leads to a question: Is confrontational strategy a way to overcome self-game, or is self-game strategy itself insufficient?
In order to answer this question, researchers from MIT, UC Berkeley and other institutions conducted some research. They chose a field that they are good at in self-games, namely Go. Specifically, they conducted an attack on KataGo, the strongest publicly available Go AI system. For a fixed network (freezing KataGo), they trained an end-to-end adversarial strategy. Using only 0.3% of the calculations when training KataGo, they obtained an adversarial strategy and used this strategy to attack KataGo. In this case, their strategy achieved a 99% winning rate against KataGo, which is comparable to the top 100 European Go players. And when KataGo used enough searches to approach superhuman levels, their win rate reached 50%. Crucially, the attacker (in this paper referring to the strategy learned in this study) cannot win by learning a general Go strategy.
Here we need to talk about KataGo. As this article says, when they wrote this article, KataGo was still the most powerful public Go AI system. With the support of search, it can be said that KataGo is very powerful, defeating ELF OpenGo and Leela Zero, which are superhuman themselves. Now the attacker in this study has defeated KataGo, which can be said to be very powerful.
Figure 1: Adversarial strategy defeats KataGo victim.
- Paper address: https://arxiv.org/pdf/2211.00241.pdf
- Research homepage: https://goattack.alignmentfund.org/adversarial-policy-katago?row=0#no_search-board
Interestingly, the adversarial strategy proposed in this study cannot defeat human players, and even amateur players can significantly outperform the proposed model.
Attack method
Previous methods such as KataGo and AlphaZero usually train the agent to play games by itself, and the game opponent is the agent itself. In this research by MIT, UC Berkeley and other institutions, a game is played between the attacker (adversary) and fixed victim (victim) agents, and the attacker is trained in this way. The research hopes to train attackers to exploit game interactions with victim agents rather than just imitating game opponents. This process is called "victim-play".
In conventional self-game, the agent models the opponent's actions by sampling from its own policy network. This approach does work. In the game of self. But in victim-play, modeling the victim from the attacker's policy network is the wrong approach. To solve this problem, this study proposes two types of adversarial MCTS (A-MCTS), including:
- A-MCTS-S: In A-MCTS-S, the researcher sets the attacker's search process as follows: when the victim moves the chess piece, sample from the victim's strategy network; When it is the attacker's turn to move a piece, samples are taken from the attacker's policy network.
- A-MCTS-R: Since A-MCTS-S underestimates the victim's ability, the study proposes A-MCTS-R, in the A-MCTS-R tree Run MCTS for the victim on each victim node. However, this change increases the computational complexity of training and inference for the attacker.
During training, the study trained adversarial strategies against games against frozen KataGo victims. Without search, the attacker can achieve >99% win rate against KataGo victims, which is comparable to the top 100 European Go players. Furthermore, the trained attacker achieved a win rate of over 80% in 64 rounds played against the victim agent, which the researchers estimate was comparable to the best human Go players.
It is worth noting that these games show that the countermeasures proposed in this study are not entirely gaming, but rather deceiving KataGo into positioning in a position favorable to the attacker. End the game early. In fact, while the attacker was able to exploit gaming strategies comparable to those of the best human Go players, it was easily defeated by human amateurs.
In order to test the attacker's ability to play against humans, the study asked Tony Tong Wang, the first author of the paper, to actually play against the attacker model. Wang had never learned the game of Go before this research project, but he still beat the attacker model by a huge margin. This shows that while the adversarial strategy proposed in this study can defeat an AI model that can defeat top human players, it cannot defeat human players. This may indicate that some AI Go models have bugs.
Evaluation results
Attack Victim Policy Network
First, the researchers evaluated themselves The attack method was evaluated on the performance of KataGo (Wu, 2019), and it was found that the A-MCTS-S algorithm achieved a winning rate of more than 99% against the search-free Latest (KataGo's latest network).
As shown in Figure 3 below, the researcher evaluated the performance of the self-confrontation strategy on the Initial and Latest policy networks. They found that during most of the training, the self-attacker achieved a high winning rate (above 90%) against both victims. However, as time goes by, the attacker overfits Latest, and the winning rate against Initial drops to about 20%.
The researchers also evaluated the best counter-strategy checkpoints against Latest, achieving a win rate of over 99%. Moreover, such a high win rate is achieved while the adversarial strategy is trained for only 3.4 × 10^7 time steps, which is 0.3% of the victim time steps.
Migrate to victims with searches
Researchers will The adversarial strategy was successfully transferred to the low search mechanism, and the adversarial strategy trained in the previous section was evaluated for its ability to search Latest. As shown in Figure 4a below, they found that A-MCTS-S’s win rate against victims dropped to 80% at 32 victim rounds. But here, the victim does not search during training and inference.
In addition, the researchers also tested A-MCTS-R and found that it performed better, with 32 victim rounds. Latest achieved a win rate of over 99%, but at round 128 the win rate dropped below 10%.
In Figure 4b, the researchers show that when the attacker comes to 4096 rounds, A-MCTS-S achieves a maximum winning rate of 54% against Latest. This is very similar to the performance of A-MCTS-R at 200 epochs, which achieved a 49% win rate.
Other evaluation
As shown in Figure 9 below, the researchers found that although Latest is a more powerful intelligence body, but the attacker trained against Latest performs better against Latest than Initial.
Finally, the researchers discussed the attack principle, including victim value prediction and hard-coded defense evaluation. As shown in Figure 5 below, all baseline attacks perform significantly worse than the adversarial strategies they were trained on.
Please refer to the original paper for more technical details.
The above is the detailed content of Use magic to defeat magic! A Go AI that rivaled top human players lost to its peers. For more information, please follow other related articles on the PHP Chinese website!
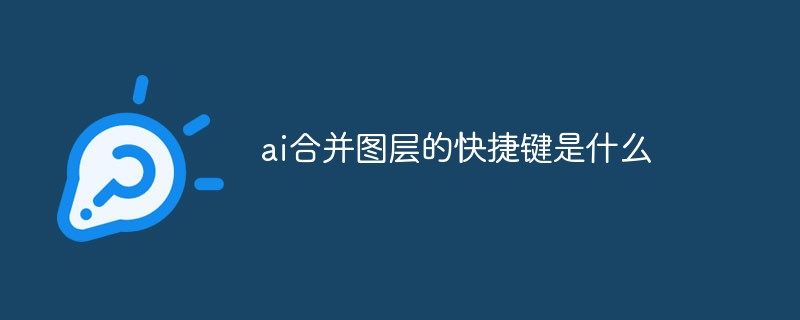
ai合并图层的快捷键是“Ctrl+Shift+E”,它的作用是把目前所有处在显示状态的图层合并,在隐藏状态的图层则不作变动。也可以选中要合并的图层,在菜单栏中依次点击“窗口”-“路径查找器”,点击“合并”按钮。
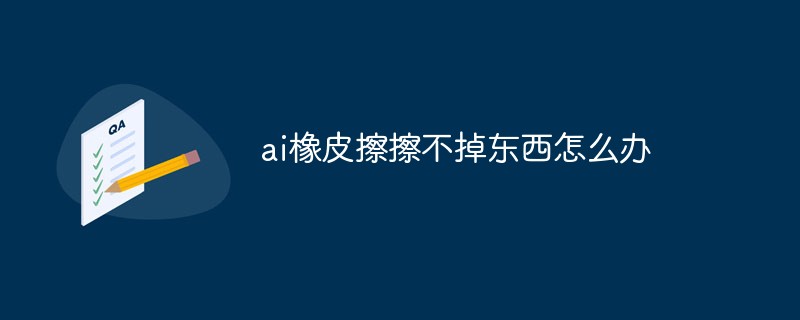
ai橡皮擦擦不掉东西是因为AI是矢量图软件,用橡皮擦不能擦位图的,其解决办法就是用蒙板工具以及钢笔勾好路径再建立蒙板即可实现擦掉东西。
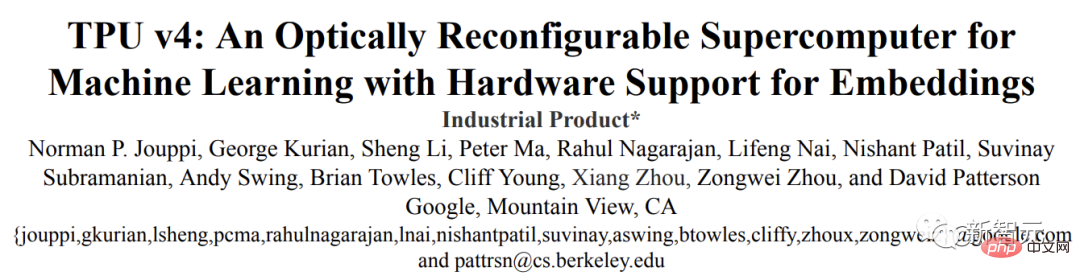
虽然谷歌早在2020年,就在自家的数据中心上部署了当时最强的AI芯片——TPU v4。但直到今年的4月4日,谷歌才首次公布了这台AI超算的技术细节。论文地址:https://arxiv.org/abs/2304.01433相比于TPU v3,TPU v4的性能要高出2.1倍,而在整合4096个芯片之后,超算的性能更是提升了10倍。另外,谷歌还声称,自家芯片要比英伟达A100更快、更节能。与A100对打,速度快1.7倍论文中,谷歌表示,对于规模相当的系统,TPU v4可以提供比英伟达A100强1.
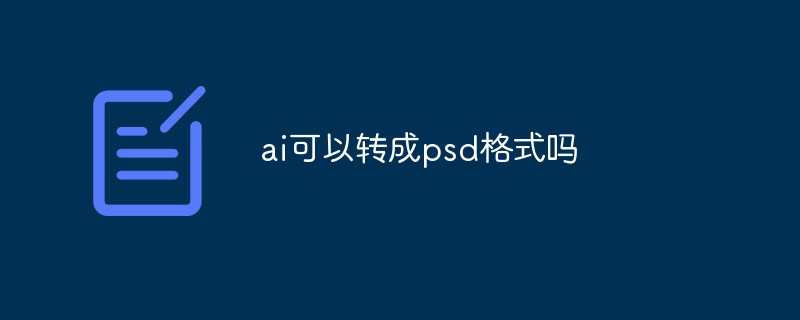
ai可以转成psd格式。转换方法:1、打开Adobe Illustrator软件,依次点击顶部菜单栏的“文件”-“打开”,选择所需的ai文件;2、点击右侧功能面板中的“图层”,点击三杠图标,在弹出的选项中选择“释放到图层(顺序)”;3、依次点击顶部菜单栏的“文件”-“导出”-“导出为”;4、在弹出的“导出”对话框中,将“保存类型”设置为“PSD格式”,点击“导出”即可;
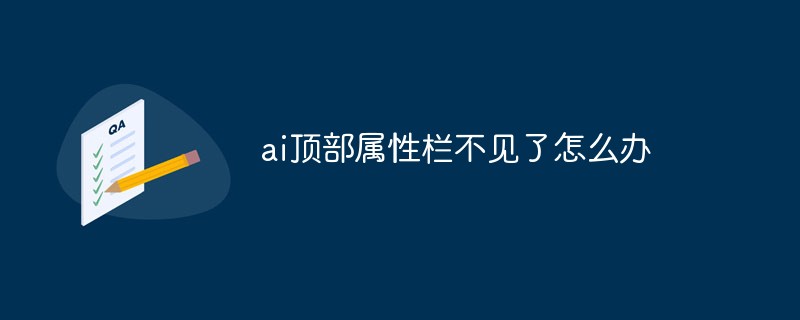
ai顶部属性栏不见了的解决办法:1、开启Ai新建画布,进入绘图页面;2、在Ai顶部菜单栏中点击“窗口”;3、在系统弹出的窗口菜单页面中点击“控制”,然后开启“控制”窗口即可显示出属性栏。
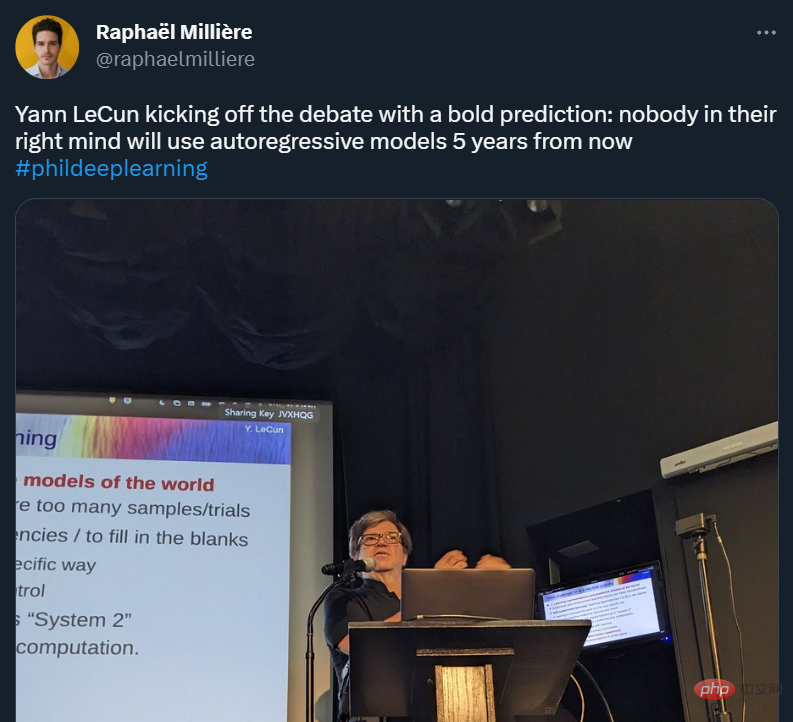
Yann LeCun 这个观点的确有些大胆。 「从现在起 5 年内,没有哪个头脑正常的人会使用自回归模型。」最近,图灵奖得主 Yann LeCun 给一场辩论做了个特别的开场。而他口中的自回归,正是当前爆红的 GPT 家族模型所依赖的学习范式。当然,被 Yann LeCun 指出问题的不只是自回归模型。在他看来,当前整个的机器学习领域都面临巨大挑战。这场辩论的主题为「Do large language models need sensory grounding for meaning and u
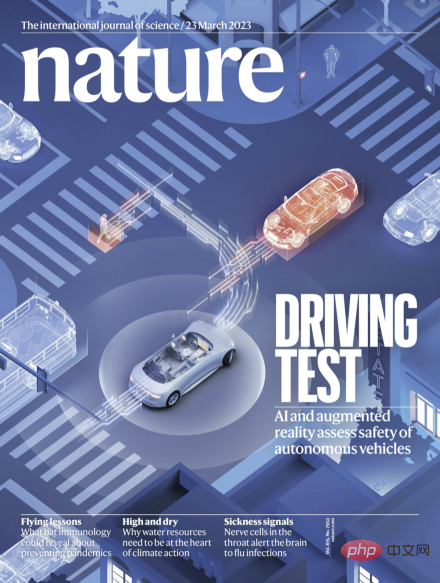
引入密集强化学习,用 AI 验证 AI。 自动驾驶汽车 (AV) 技术的快速发展,使得我们正处于交通革命的风口浪尖,其规模是自一个世纪前汽车问世以来从未见过的。自动驾驶技术具有显着提高交通安全性、机动性和可持续性的潜力,因此引起了工业界、政府机构、专业组织和学术机构的共同关注。过去 20 年里,自动驾驶汽车的发展取得了长足的进步,尤其是随着深度学习的出现更是如此。到 2015 年,开始有公司宣布他们将在 2020 之前量产 AV。不过到目前为止,并且没有 level 4 级别的 AV 可以在市场
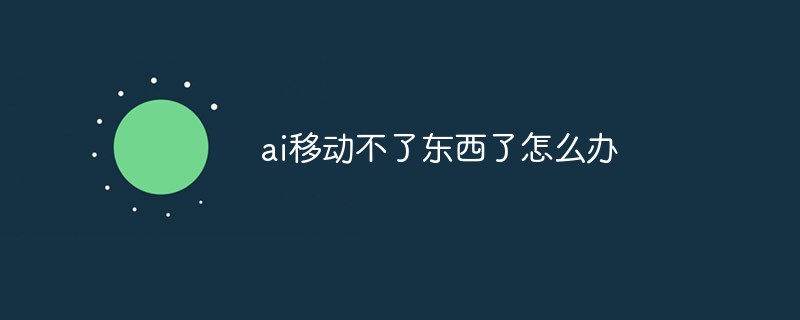
ai移动不了东西的解决办法:1、打开ai软件,打开空白文档;2、选择矩形工具,在文档中绘制矩形;3、点击选择工具,移动文档中的矩形;4、点击图层按钮,弹出图层面板对话框,解锁图层;5、点击选择工具,移动矩形即可。


Hot AI Tools

Undresser.AI Undress
AI-powered app for creating realistic nude photos

AI Clothes Remover
Online AI tool for removing clothes from photos.

Undress AI Tool
Undress images for free

Clothoff.io
AI clothes remover

AI Hentai Generator
Generate AI Hentai for free.

Hot Article

Hot Tools

SublimeText3 Chinese version
Chinese version, very easy to use

mPDF
mPDF is a PHP library that can generate PDF files from UTF-8 encoded HTML. The original author, Ian Back, wrote mPDF to output PDF files "on the fly" from his website and handle different languages. It is slower than original scripts like HTML2FPDF and produces larger files when using Unicode fonts, but supports CSS styles etc. and has a lot of enhancements. Supports almost all languages, including RTL (Arabic and Hebrew) and CJK (Chinese, Japanese and Korean). Supports nested block-level elements (such as P, DIV),

Notepad++7.3.1
Easy-to-use and free code editor

DVWA
Damn Vulnerable Web App (DVWA) is a PHP/MySQL web application that is very vulnerable. Its main goals are to be an aid for security professionals to test their skills and tools in a legal environment, to help web developers better understand the process of securing web applications, and to help teachers/students teach/learn in a classroom environment Web application security. The goal of DVWA is to practice some of the most common web vulnerabilities through a simple and straightforward interface, with varying degrees of difficulty. Please note that this software

SecLists
SecLists is the ultimate security tester's companion. It is a collection of various types of lists that are frequently used during security assessments, all in one place. SecLists helps make security testing more efficient and productive by conveniently providing all the lists a security tester might need. List types include usernames, passwords, URLs, fuzzing payloads, sensitive data patterns, web shells, and more. The tester can simply pull this repository onto a new test machine and he will have access to every type of list he needs.
