But the unfortunate reality is that 85% of AI and ML projects cannot be fully delivered, and only 53% of projects can go from prototype to production. Still, U.S. spending on artificial intelligence will grow to $120 billion by 2025, an increase of 20% or more, according to recent IDC spending guidance.
Therefore, it’s important to avoid five common mistakes that often cause AI and ML projects to fail.
1. Understand the resources required to train ML algorithms, especially data resources
While it sounds great to say that AI and ML are being used to revolutionize company processes, the reality is that 80% of companies Finding these items is harder than expected.
In order for these projects to be successful, a clear understanding of what is needed in terms of resources and people is needed. One of the most common mistakes is not understanding how to obtain the right training data - not only is this critical to the success of such a program, but it also requires a lot of effort and expertise to complete successfully. Most companies looking to adopt AI/ML projects do not have access to the amount or diversity of data needed to ensure high-quality, unbiased results.
However, failure to do this often creates huge obstacles to success, causing project costs to soar and project confidence to plummet.
There is no shortage of training data for companies to purchase, and many third-party data companies can provide services. The problem is that just because a company can easily buy large amounts of data cheaply doesn't mean it's high-quality training data, which is what successful AI and ML projects require. Rather than simply buying one-size-fits-all data, companies need data that is project-specific.
Therefore, in order to reduce bias, it is important to ensure that the data is representative of a broad and diverse audience. Data also needs to be accurately annotated for your algorithm, and data should always be checked for compliance with data standards, data privacy laws, and security measures.
2. Don’t expect that the development of artificial intelligence will be smooth sailing
The training of ML algorithms is not a strange process. Once training begins and the data model is better understood, changes must continue to be made to the data collected. It's not easy to know what data you actually need before the algorithm training process begins. For example, you might realize there's a problem with the training set or the way the data was collected.
Like traditional software development, artificial intelligence is essentially composed of software and requires continuous and stable investment to gradually generate benefits. And in this process, never take it lightly.
3. Always Integrate Quality Assurance (QA) Testing
Often, QA testing is considered an add-on or form of ensuring that the product works correctly, rather than being viewed as optimizing the product across all iterations essential tool. In fact, QA testing is an important part of successful AI development. Results validation should be integrated into every stage of the AI development process to reduce costs, accelerate development timelines, and ensure efficient allocation of resources.
4. Schedule frequent application feedback
While it may be daunting to imagine, the reality is that AI projects are never truly finished. Even if a project exceeds accuracy and performance expectations, you still have room for improvement and improvement. Additionally, algorithms make decisions based on constantly changing things (opinions, conversations, images, etc.). For an AI experience to be successful now and in the future, it must be retrained on a rolling basis to adapt to new social circumstances, technological developments, and other changes that impact data.
In fact, companies that see the most positive impacts from AI adoption follow core and AI best practices and invest in AI more efficiently and effectively than their peers. This includes testing the performance of AI models before deployment, tracking performance to see if results improve over time, and developing good protocols to ensure data quality.
By developing a robust approach to developing AI programs, companies can avoid these common mistakes and ensure the long-term success of their AI and ML initiatives.
The above is the detailed content of Four common obstacles in AI/ML projects. For more information, please follow other related articles on the PHP Chinese website!
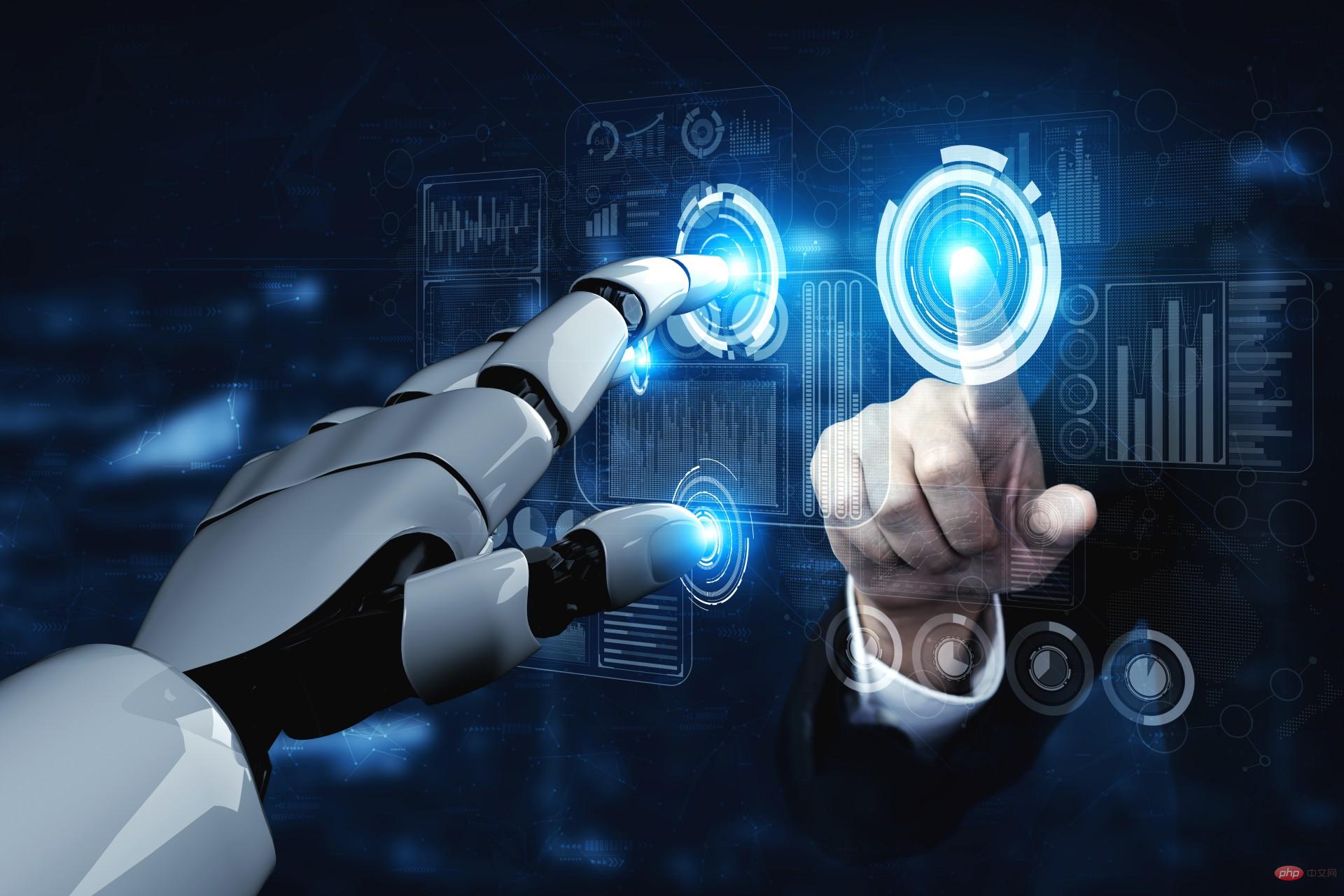
机器学习是一个不断发展的学科,一直在创造新的想法和技术。本文罗列了2023年机器学习的十大概念和技术。 本文罗列了2023年机器学习的十大概念和技术。2023年机器学习的十大概念和技术是一个教计算机从数据中学习的过程,无需明确的编程。机器学习是一个不断发展的学科,一直在创造新的想法和技术。为了保持领先,数据科学家应该关注其中一些网站,以跟上最新的发展。这将有助于了解机器学习中的技术如何在实践中使用,并为自己的业务或工作领域中的可能应用提供想法。2023年机器学习的十大概念和技术:1. 深度神经网
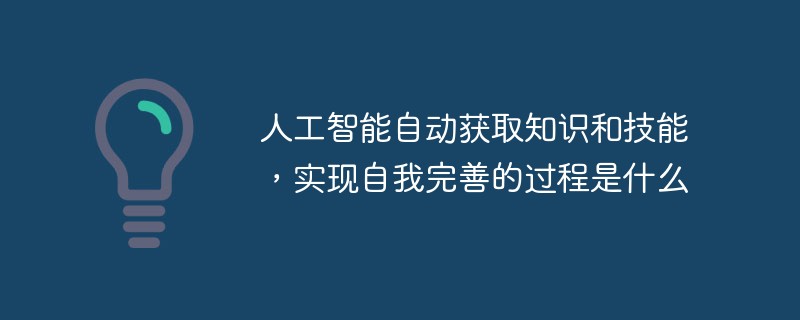
实现自我完善的过程是“机器学习”。机器学习是人工智能核心,是使计算机具有智能的根本途径;它使计算机能模拟人的学习行为,自动地通过学习来获取知识和技能,不断改善性能,实现自我完善。机器学习主要研究三方面问题:1、学习机理,人类获取知识、技能和抽象概念的天赋能力;2、学习方法,对生物学习机理进行简化的基础上,用计算的方法进行再现;3、学习系统,能够在一定程度上实现机器学习的系统。

本文将详细介绍用来提高机器学习效果的最常见的超参数优化方法。 译者 | 朱先忠审校 | 孙淑娟简介通常,在尝试改进机器学习模型时,人们首先想到的解决方案是添加更多的训练数据。额外的数据通常是有帮助(在某些情况下除外)的,但生成高质量的数据可能非常昂贵。通过使用现有数据获得最佳模型性能,超参数优化可以节省我们的时间和资源。顾名思义,超参数优化是为机器学习模型确定最佳超参数组合以满足优化函数(即,给定研究中的数据集,最大化模型的性能)的过程。换句话说,每个模型都会提供多个有关选项的调整“按钮
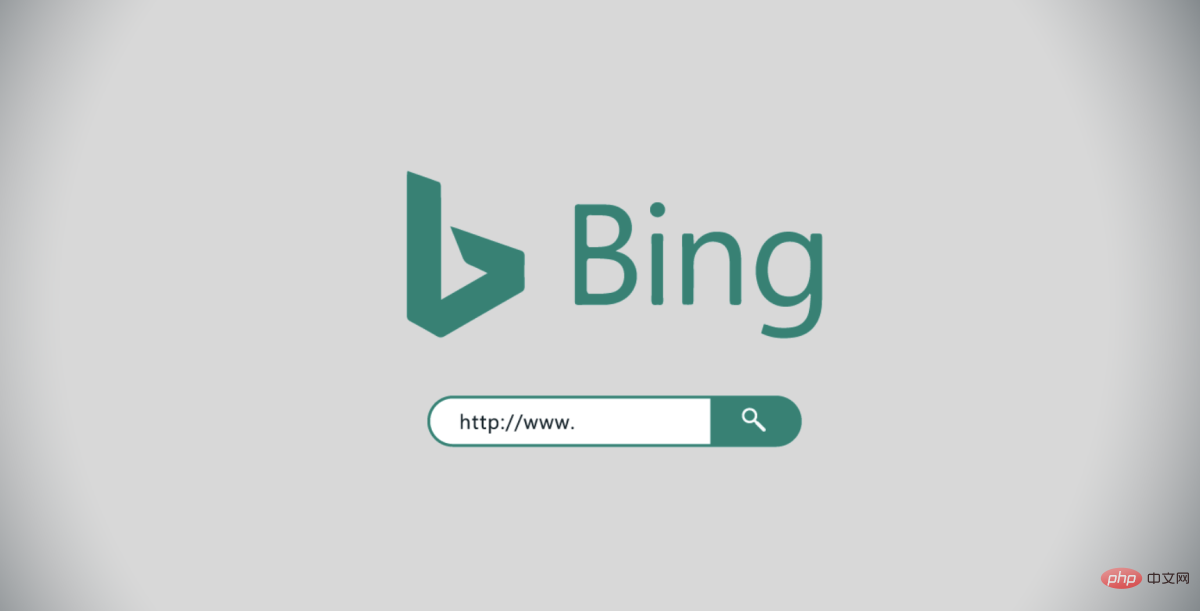
截至3月20日的数据显示,自微软2月7日推出其人工智能版本以来,必应搜索引擎的页面访问量增加了15.8%,而Alphabet旗下的谷歌搜索引擎则下降了近1%。 3月23日消息,外媒报道称,分析公司Similarweb的数据显示,在整合了OpenAI的技术后,微软旗下的必应在页面访问量方面实现了更多的增长。截至3月20日的数据显示,自微软2月7日推出其人工智能版本以来,必应搜索引擎的页面访问量增加了15.8%,而Alphabet旗下的谷歌搜索引擎则下降了近1%。这些数据是微软在与谷歌争夺生
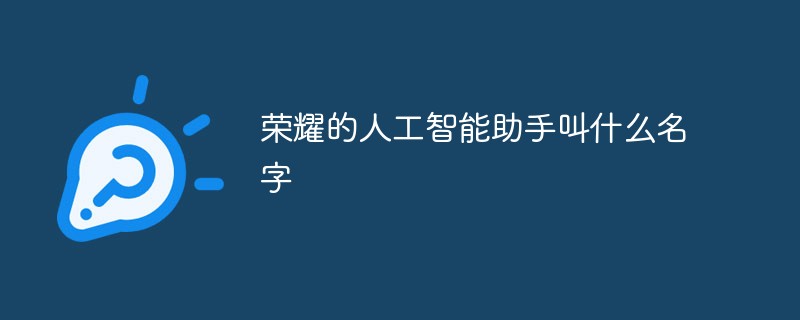
荣耀的人工智能助手叫“YOYO”,也即悠悠;YOYO除了能够实现语音操控等基本功能之外,还拥有智慧视觉、智慧识屏、情景智能、智慧搜索等功能,可以在系统设置页面中的智慧助手里进行相关的设置。
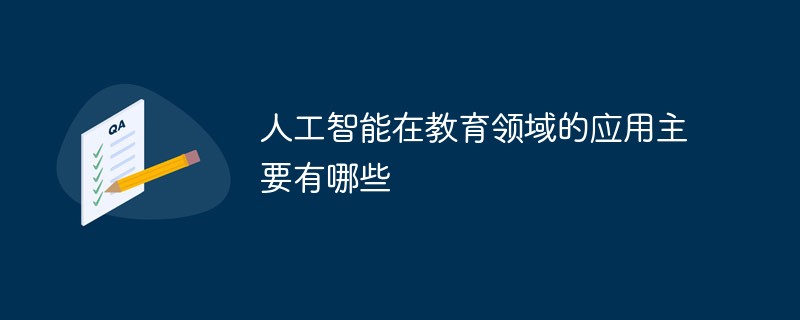
人工智能在教育领域的应用主要有个性化学习、虚拟导师、教育机器人和场景式教育。人工智能在教育领域的应用目前还处于早期探索阶段,但是潜力却是巨大的。
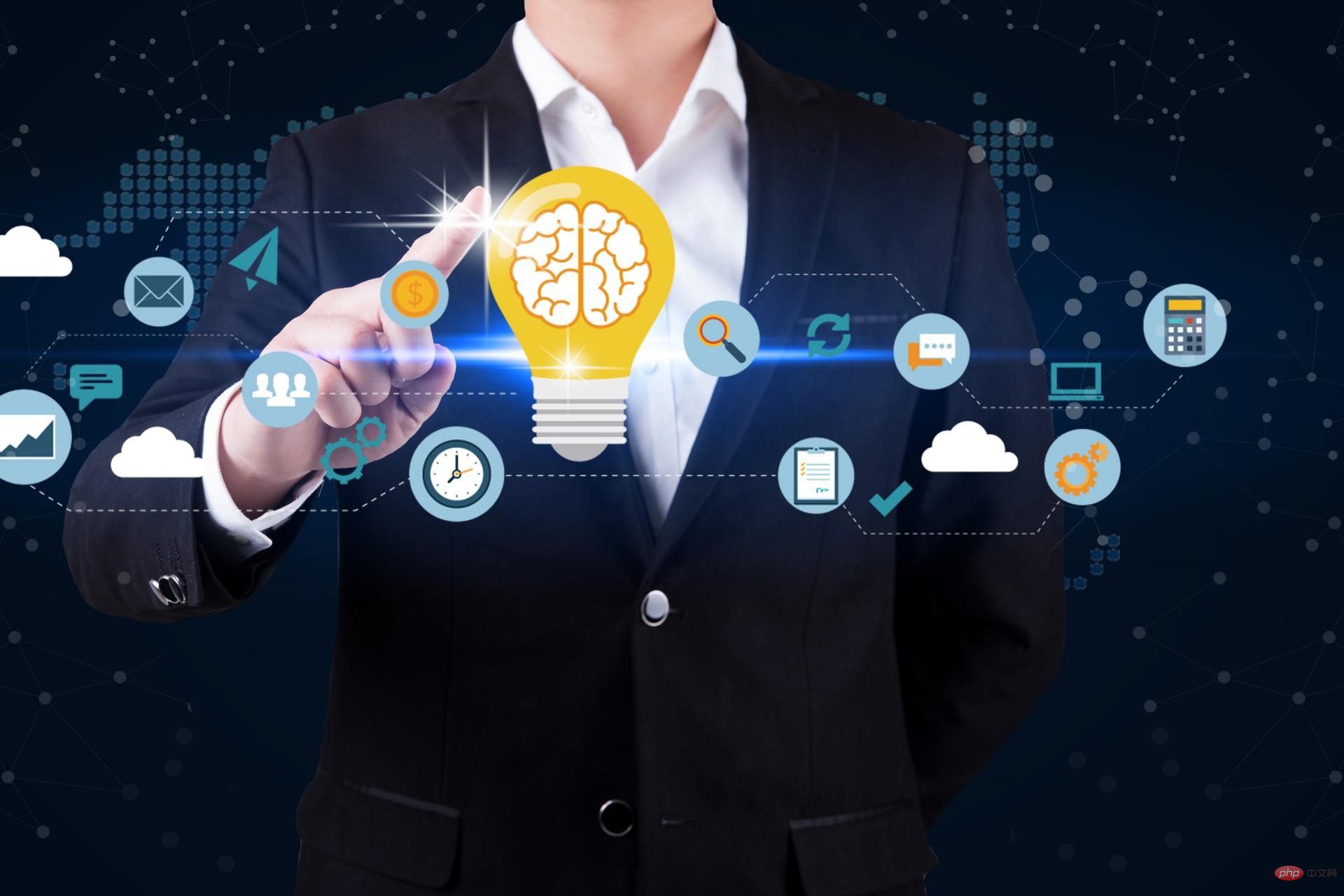
阅读论文可以说是我们的日常工作之一,论文的数量太多,我们如何快速阅读归纳呢?自从ChatGPT出现以后,有很多阅读论文的服务可以使用。其实使用ChatGPT API非常简单,我们只用30行python代码就可以在本地搭建一个自己的应用。 阅读论文可以说是我们的日常工作之一,论文的数量太多,我们如何快速阅读归纳呢?自从ChatGPT出现以后,有很多阅读论文的服务可以使用。其实使用ChatGPT API非常简单,我们只用30行python代码就可以在本地搭建一个自己的应用。使用 Python 和 C
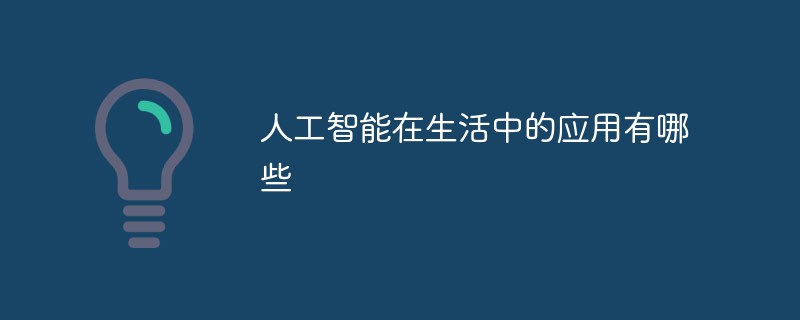
人工智能在生活中的应用有:1、虚拟个人助理,使用者可通过声控、文字输入的方式,来完成一些日常生活的小事;2、语音评测,利用云计算技术,将自动口语评测服务放在云端,并开放API接口供客户远程使用;3、无人汽车,主要依靠车内的以计算机系统为主的智能驾驶仪来实现无人驾驶的目标;4、天气预测,通过手机GPRS系统,定位到用户所处的位置,在利用算法,对覆盖全国的雷达图进行数据分析并预测。


Hot AI Tools

Undresser.AI Undress
AI-powered app for creating realistic nude photos

AI Clothes Remover
Online AI tool for removing clothes from photos.

Undress AI Tool
Undress images for free

Clothoff.io
AI clothes remover

AI Hentai Generator
Generate AI Hentai for free.

Hot Article

Hot Tools

SublimeText3 Chinese version
Chinese version, very easy to use
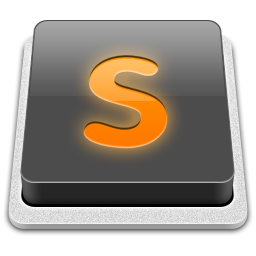
SublimeText3 Mac version
God-level code editing software (SublimeText3)

MantisBT
Mantis is an easy-to-deploy web-based defect tracking tool designed to aid in product defect tracking. It requires PHP, MySQL and a web server. Check out our demo and hosting services.
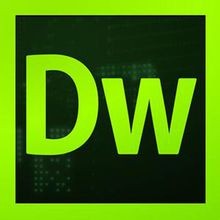
Dreamweaver CS6
Visual web development tools

DVWA
Damn Vulnerable Web App (DVWA) is a PHP/MySQL web application that is very vulnerable. Its main goals are to be an aid for security professionals to test their skills and tools in a legal environment, to help web developers better understand the process of securing web applications, and to help teachers/students teach/learn in a classroom environment Web application security. The goal of DVWA is to practice some of the most common web vulnerabilities through a simple and straightforward interface, with varying degrees of difficulty. Please note that this software
