


We all know that 90% of the human brain is self-supervised learning, and organisms will constantly make predictions about what will happen next. Self-supervised learning means that decisions can be made without external intervention. There are only a few cases where we will accept external feedback, such as the teacher saying: "You made a mistake." Now some scholars have discovered that the self-supervised learning mechanism of large language models is very much like our brains. Quanta Magazine, a well-known popular science media, recently reported that more and more studies have found that self-supervised learning models, especially the self-learning methods of large-scale language models, are very similar to the learning model of our brains.
Common AI systems in the past used a large amount of labeled data for training. For example, images might be labeled "tabby cat" or "tiger cat" to train an artificial neural network to correctly distinguish between tabby and tiger.
## This kind of "self-supervised" training requires manual and laborious work Label data, and neural networks often take shortcuts and learn to associate labels with minimal, and sometimes superficial, information. For example, a neural network might use the presence of grass to identify photos of cows, since cows are often photographed in fields. Alexei Efros, a computer scientist at the University of California, Berkeley, said: The algorithms we are training are like It's undergraduates who haven't been to class for an entire semester, and although they didn't study the material systematically, they did well on the exam.
Furthermore, for researchers interested in the intersection of animal intelligence and machine intelligence, this kind of "supervised learning" may be limited to it Revealed about the biological brain. Many animals, including humans, do not use labeled data sets to learn. For the most part, they explore their environment on their own, and in doing so, they gain a rich and deep understanding of the world.
#Now some computational neuroscientists have begun exploring neural networks trained with little or no human-labeled data. Recent findings suggest that computational models of animal visual and auditory systems built using self-supervised learning models more closely approximate brain function than supervised learning models.
#To some neuroscientists, artificial neural networks seem to be beginning to reveal ways to use the brain as an analogy for machine learning. Flawed Supervision
About 10 years ago, brain models inspired by artificial neural networks began to emerge, and at the same time a project called AlexNet Neural networks have revolutionized the task of classifying unknown images.
This result was published in the paper "ImageNet Classification with Deep Convolutional Neural Networks" by Alex Krizhevsky, Ilya Sutskever, and Geoffrey E. Hinton.
Paper address: https://dl.acm.org/doi/10.1145/3065386 Like all neural networks, this network consists of multiple layers Composed of artificial neurons, in which the weights of connections between different neurons are different.
If the neural network fails to correctly classify an image, the learning algorithm updates the weights of the connections between neurons to reduce the likelihood of misclassification in the next round of training sex. The algorithm repeats this process multiple times, adjusting the weights, until the network's error rate is acceptably low. Later, neuroscientists used AlexNet to develop the first computational model of the primate visual system.
When monkeys and artificial neural networks are shown the same image , the activity of real neurons and artificial neurons showed similar responses. Similar results were obtained on artificial models of hearing and odor detection. But as the field developed, researchers realized the limitations of self-supervised training. In 2017, computer scientist Leon Gatys of the University of Tübingen in Germany and his colleagues took a photo of a Ford Model T and then covered the photo with a leopard skin pattern.
And the artificial intelligence neural network correctly classified the original image as Model T, but see the modified image as a leopard. The reason is that it only focuses on the image texture and does not understand the shape of the car (or leopard). Self-supervised learning models are designed to avoid such problems. Friedemann Zenke, a computational neuroscientist at the Friedrich Michel Institute for Biomedical Research in Basel, Switzerland, said,
In this approach, humans do not label the data; instead, the labels come from the data itself. Self-supervised algorithms essentially create gaps in the data and ask the neural network to fill them.
# For example, in a so-called large language model, a training algorithm will show a neural network the first few words of a sentence and ask it to predict the next word. When trained using large amounts of text collected from the Internet, the model appeared to learn the syntactic structure of the language, demonstrating impressive linguistic prowess—all without external labels or supervision. Similar efforts are underway in computer vision. At the end of 2021, He Kaiming and his colleagues demonstrated the famous masked auto-encoder research "Masked Auto-Encoder" (MAE).
##Paper address: https://arxiv.org/ abs/2111.06377 MAE converts the unmasked portion into a latent representation—a compressed mathematical description that contains important information about the object. In the case of images, the underlying representation might be a mathematical description that includes the shape of the objects in the image. The decoder then converts these representations back into full images.
In such a system, some neuroscientists believe that , our brains are actually self-supervised learning. "I think there's no question that 90 percent of what the brain does is self-supervised learning," says Blake Richards, a computational neuroscientist at McGill University and Quebec's Institute of Artificial Intelligence (Mila). The biological brain is Think of it as constantly predicting, for example, the future position of an object as it moves, or the next word in a sentence, much like a self-supervised learning algorithm trying to predict gaps in an image or a piece of text.
## Computational neuroscientist Blake Richards created an AI system that mimics a living body Visual Networks in the Brain Richards and his team created a self-supervised model that hints at an answer. They trained an artificial intelligence that combined two different neural networks.
The first, called the ResNet architecture, is designed for processing images; the second, called the Recurrent Network, can keep track of previous input sequences , predict the next expected input. To train the joint AI, the team starts with a sequence of videos, say 10 frames, and lets ResNet process them one by one.
#Then, the recurrent network predicts the latent representation of frame 11 instead of simply matching the previous 10 frames. Self-supervised learning algorithms compare predicted values to actual values and instruct the neural network to update its weights to make better predictions.
#To test it further, the researchers showed the AI a set of videos that researchers at the Allen Institute for Brain Science in Seattle had previously shown to mice. video. Like primates, mice have brain regions dedicated to static images and movement. Allen researchers recorded neural activity in the visual cortex of mice while the mice watched videos.
#Richards’ team found similarities in the way AI and living brains respond to videos. During training, one pathway in the artificial neural network became more similar to a ventral, object-detection area of the mouse brain, while another pathway became similar to a dorsal area focused on movement.
These results suggest that our visual system has two specialized pathways as they help predict the future of vision; a single pathway is not good enough . Models of the human auditory system tell a similar story. In June, a team led by Jean-Rémi King, a research scientist at Meta AI, trained an artificial intelligence called Wav2Vec 2.0, which uses a neural network to convert audio into latent representations. The researchers masked some of these representations and then fed them into another component of the neural network called a converter.
#During the training process, the converter predicts blocked information. In the process, the AI as a whole learns to transform sounds into underlying representations, again, without the need for labels. The team used approximately 600 hours of speech data to train the network. "That's about what a kid gets in the first two years of experience," King said.
## Meta AI’s Jean-Remy King helped train developed an artificial intelligence that processes audio in a way that mimics the brain—in part by predicting what should happen next. Once the system was trained, the researchers played it audiobook portions in English, French, and Mandarin, and then fed the AI The performance was compared with data from 412 people, all native speakers of the three languages, who listened to the same length of audio while MRI scans were imaging their brains.
The results show that although the fMRI images are noisy and have low resolution, the AI neural network and the human brain "not only correlate with each other, but also function in a systematic way." way to relate”. Activity in the early layers of AI is consistent with activity in the primary auditory cortex, while activity in the deepest layers of AI is consistent with activity in higher levels of the brain, such as the prefrontal cortex. "
This is beautiful data, not conclusive, but compelling evidence of how we learn language. A lot of it is predicting what will be said next." Someone disagrees: simulated brain? The models and algorithms are far behind
Of course, not everyone agrees with this statement. Josh McDermott, a computational neuroscientist at MIT, has used supervised and self-supervised learning to study models of vision and hearing. His lab engineered synthetic audio and visual signals that, to humans, would be just elusive noise. #However, to artificial neural networks, these signals appear to be indistinguishable from real language and images. This shows that the representations formed deep in neural networks, even with self-supervised learning, are not the same as those in our brains. McDermott said: "These self-supervised learning methods are an improvement because you can learn representations that can support a lot of recognition behaviors without needing all the labels. But there are still many characteristics of supervised models." The algorithm itself also needs more improvements. For example, in Meta AI's Wav2Vec 2.0 model, the AI only predicts the potential representation of a sound for tens of milliseconds, which is shorter than the time it takes for a person to utter a noise syllable, let alone predict a word. #To truly make AI models similar to the human brain, we still have a lot to do, Jin said. If the similarities between the brain and self-supervised learning models discovered so far hold true in other sensory tasks, it would be an even stronger indication that whatever miraculous abilities our brains have, some form of self-supervised learning is required.
The above is the detailed content of 90% of the human brain is self-supervised learning. How far is a large AI model from simulating the brain?. For more information, please follow other related articles on the PHP Chinese website!
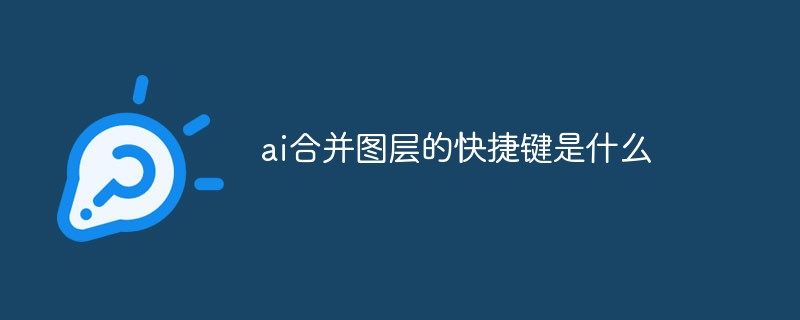
ai合并图层的快捷键是“Ctrl+Shift+E”,它的作用是把目前所有处在显示状态的图层合并,在隐藏状态的图层则不作变动。也可以选中要合并的图层,在菜单栏中依次点击“窗口”-“路径查找器”,点击“合并”按钮。
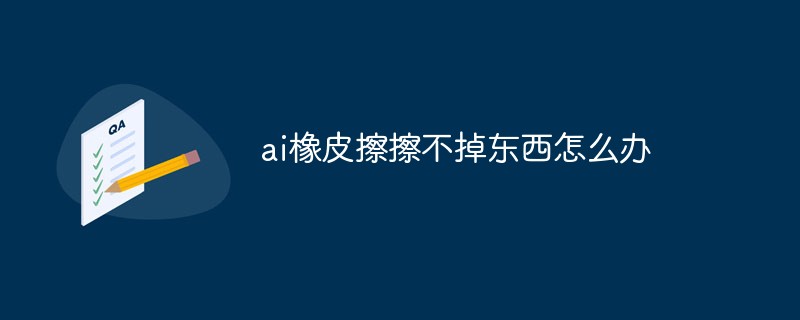
ai橡皮擦擦不掉东西是因为AI是矢量图软件,用橡皮擦不能擦位图的,其解决办法就是用蒙板工具以及钢笔勾好路径再建立蒙板即可实现擦掉东西。
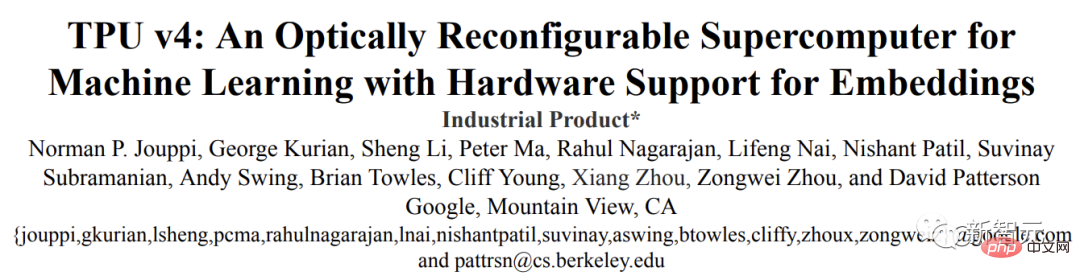
虽然谷歌早在2020年,就在自家的数据中心上部署了当时最强的AI芯片——TPU v4。但直到今年的4月4日,谷歌才首次公布了这台AI超算的技术细节。论文地址:https://arxiv.org/abs/2304.01433相比于TPU v3,TPU v4的性能要高出2.1倍,而在整合4096个芯片之后,超算的性能更是提升了10倍。另外,谷歌还声称,自家芯片要比英伟达A100更快、更节能。与A100对打,速度快1.7倍论文中,谷歌表示,对于规模相当的系统,TPU v4可以提供比英伟达A100强1.
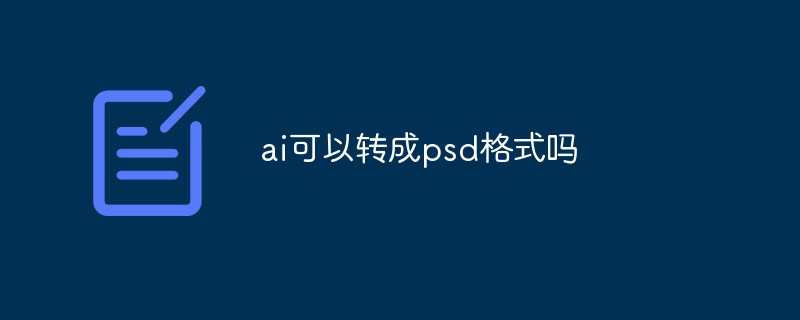
ai可以转成psd格式。转换方法:1、打开Adobe Illustrator软件,依次点击顶部菜单栏的“文件”-“打开”,选择所需的ai文件;2、点击右侧功能面板中的“图层”,点击三杠图标,在弹出的选项中选择“释放到图层(顺序)”;3、依次点击顶部菜单栏的“文件”-“导出”-“导出为”;4、在弹出的“导出”对话框中,将“保存类型”设置为“PSD格式”,点击“导出”即可;
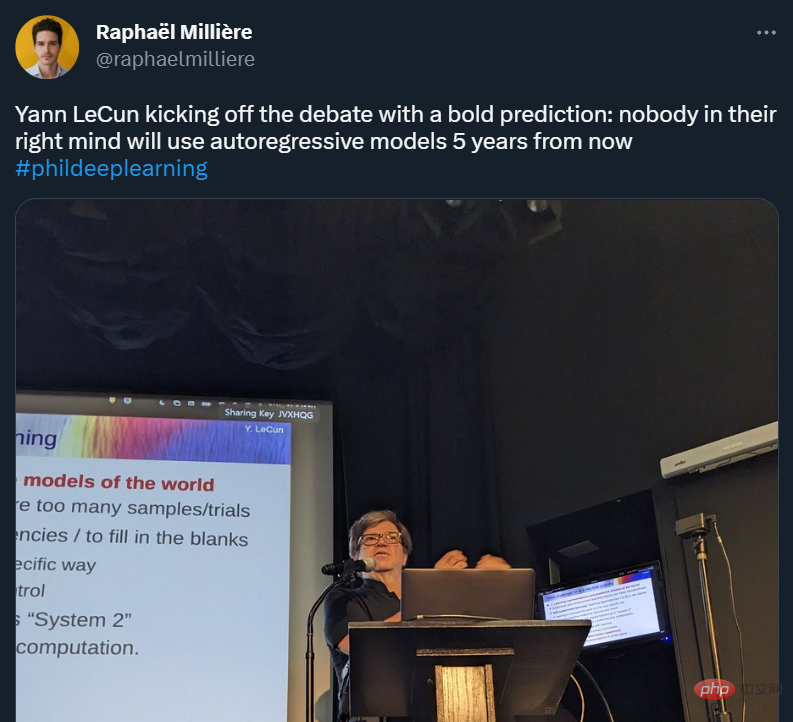
Yann LeCun 这个观点的确有些大胆。 「从现在起 5 年内,没有哪个头脑正常的人会使用自回归模型。」最近,图灵奖得主 Yann LeCun 给一场辩论做了个特别的开场。而他口中的自回归,正是当前爆红的 GPT 家族模型所依赖的学习范式。当然,被 Yann LeCun 指出问题的不只是自回归模型。在他看来,当前整个的机器学习领域都面临巨大挑战。这场辩论的主题为「Do large language models need sensory grounding for meaning and u
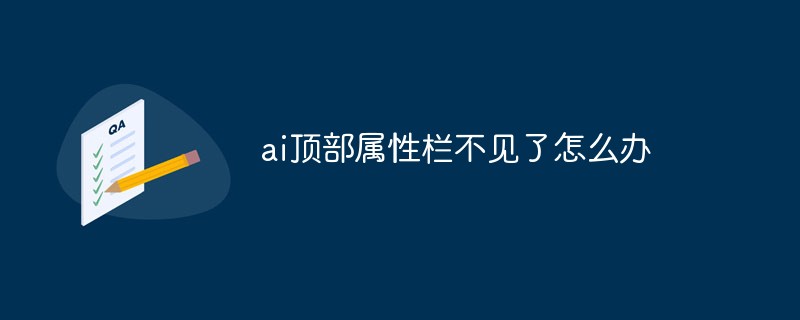
ai顶部属性栏不见了的解决办法:1、开启Ai新建画布,进入绘图页面;2、在Ai顶部菜单栏中点击“窗口”;3、在系统弹出的窗口菜单页面中点击“控制”,然后开启“控制”窗口即可显示出属性栏。
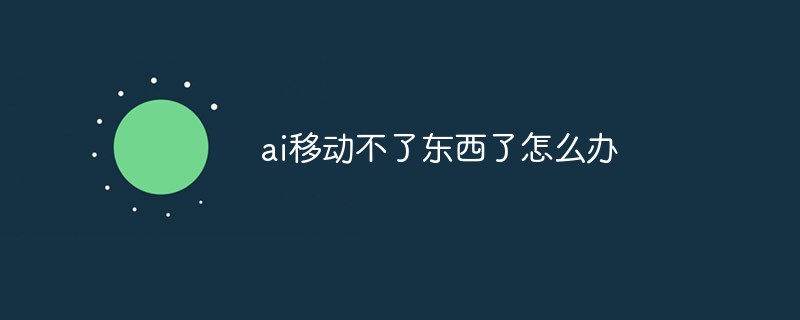
ai移动不了东西的解决办法:1、打开ai软件,打开空白文档;2、选择矩形工具,在文档中绘制矩形;3、点击选择工具,移动文档中的矩形;4、点击图层按钮,弹出图层面板对话框,解锁图层;5、点击选择工具,移动矩形即可。
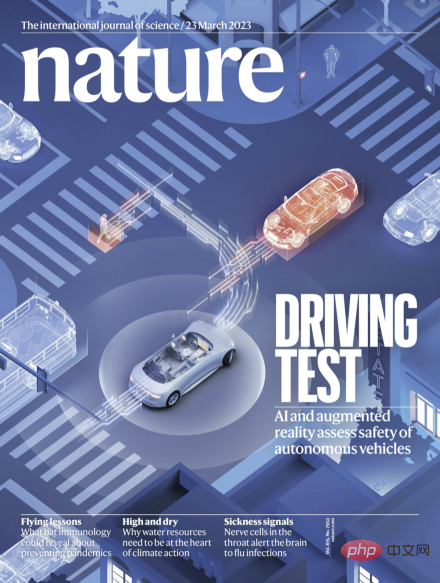
引入密集强化学习,用 AI 验证 AI。 自动驾驶汽车 (AV) 技术的快速发展,使得我们正处于交通革命的风口浪尖,其规模是自一个世纪前汽车问世以来从未见过的。自动驾驶技术具有显着提高交通安全性、机动性和可持续性的潜力,因此引起了工业界、政府机构、专业组织和学术机构的共同关注。过去 20 年里,自动驾驶汽车的发展取得了长足的进步,尤其是随着深度学习的出现更是如此。到 2015 年,开始有公司宣布他们将在 2020 之前量产 AV。不过到目前为止,并且没有 level 4 级别的 AV 可以在市场


Hot AI Tools

Undresser.AI Undress
AI-powered app for creating realistic nude photos

AI Clothes Remover
Online AI tool for removing clothes from photos.

Undress AI Tool
Undress images for free

Clothoff.io
AI clothes remover

AI Hentai Generator
Generate AI Hentai for free.

Hot Article

Hot Tools

SublimeText3 Linux new version
SublimeText3 Linux latest version

MinGW - Minimalist GNU for Windows
This project is in the process of being migrated to osdn.net/projects/mingw, you can continue to follow us there. MinGW: A native Windows port of the GNU Compiler Collection (GCC), freely distributable import libraries and header files for building native Windows applications; includes extensions to the MSVC runtime to support C99 functionality. All MinGW software can run on 64-bit Windows platforms.

SAP NetWeaver Server Adapter for Eclipse
Integrate Eclipse with SAP NetWeaver application server.
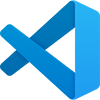
VSCode Windows 64-bit Download
A free and powerful IDE editor launched by Microsoft

Notepad++7.3.1
Easy-to-use and free code editor
