


Comparison of FastAPI, Flask and Streamlit for Web Development
Python has become one of the most popular programming languages for web development, thanks to its simplicity, versatility, and vast collection of libraries and frameworks. Developers have a variety of options when it comes to building web applications in Python, from full-stack frameworks like Django and Pyramid, to lightweight microframeworks like Flask and FastAPI, to specialized tools like Streamlit for data science applications.
In this article, we will compare three of the most popular Python web frameworks - FastAPI, Flask, and Streamlit - to help you design Choose the right tool. We’ll explore the features, strengths, and weaknesses of each framework and highlight the use cases they excel at.
FastAPI
FastAPI is a modern, fast (hence the name) web framework for building APIs using Python 3.6 based on standard Python type hints. It leverages the asynchronous programming capabilities of Python's asyncio library and the performance of the Pydantic library for data validation and serialization. FastAPI provides automatic API documentation compliant with OpenAPI standards, including support for interactive documentation using Swagger UI and ReDoc. It also has built-in support for OAuth2 authentication and FastAPI dependencies for managing shared data and resources.
FastAPI’s strengths are its speed, ease of use, and support for asynchronous programming. It can easily handle high concurrency and throughput, making it ideal for building high-performance APIs and microservices. Its built-in support for data validation and serialization reduces the amount of code you program and improves code quality. FastAPI's documentation feature makes it easy to document the API and generate client libraries.
Flask
Flask is a lightweight, scalable and flexible Python web framework that follows a microservice architecture. It provides a simple and intuitive API for building web applications with minimal coding. Flask is built on the Werkzeug WSGI toolkit and Jinja2 templating engine. It also supports third-party extensions for adding functionality, such as SQLAlchemy for database access, WTForms for form validation, and Flask-RESTful for building RESTful APIs.
Flask’s strengths are its simplicity, flexibility, and scalability. It provides a low-level API that allows developers to build applications with full control over the code. Flask's lightweight nature makes it easy to learn and use, especially for small and medium-sized projects. Its extensibility allows developers to add third-party libraries and plug-ins to enhance the functionality of their applications.
Streamlit
Streamlit is a Python library for building data science web applications with minimal coding. It provides a simple and intuitive API for creating interactive and responsive data visualizations, dashboards, and machine learning models. Streamlit is built on Python’s data science stack, including NumPy, Pandas, Matplotlib, and Scikit-learn. It also supports third-party extensions for adding functionality, such as Streamlit-Altair for advanced data visualization and Streamlit-Geopandas for processing geospatial data.
Streamlit’s strengths are its simplicity, interactivity, and focus on data science. It provides a high-level API that allows developers to create web applications with minimal coding, especially for data science use cases. Streamlit's interactivity allows users to interact with data visualizations and machine learning models in real time, making it an ideal tool for data exploration and experimentation. It focuses on data science, allowingdevelopers to leverage Python's powerful data science library to build web applications.
Feature ComparisonTo compare FastAPI, Flask and Streamlit we will use the following criteria:
- Speed and performance: How fast and efficient is the framework?
- Ease of use: How easy is it to learn and use the framework?
- Documentation: How well documented is the framework?
- Extensibility: How easy is it to add third-party libraries and plugins?
- Data Science Capabilities: How well does the framework support data science use cases?
FastAPI is known for its speed and performance, thanks to its support for asynchronous programming and for data validation and sequencing ized Pydantic library. According to benchmarks, FastAPI can handle up to 70,000 requests per second, making it one of the fastest Python web frameworks available.
Flask is also known for its speed and performance, although it is not as fast as FastAPI. Flask can handle up to 5,000 requests per second, which is still impressive for a microframework.
Streamlit is not designed for high-performance web applications, but for interactive data visualization and data science experiments. It can handle moderate traffic, but is not optimized for handling large amounts of data or high concurrency.
Ease of use
FastAPI provides a modern and intuitive API that is easy to use, especially suitable for developers familiar with Python's type hints and asynchronous programming. FastAPI's automatic API documentation and built-in support for data validation and serialization reduce the amount of code that needs to be written and improve code quality.
Flask’s API is simple and easy to learn, even for beginners. Flask's minimalist design makes it easy to use for small to medium-sized projects.
Streamlit provides an easy-to-use high-level API, especially suitable for data science use cases. Streamlit's focus on interactivity and responsiveness makes it easy to create interactive data visualizations and machine learning models with minimal coding.
Documentation
FastAPI’s documentation is well organized and comprehensive, with detailed examples and tutorials. FastAPI's automatic API documentation is generated using OpenAPI standards and includes support for Swagger UI and ReDoc.
Flask’s documentation is also well organized and comprehensive, with plenty of third-party extensions and tutorials. Flask's documentation is not as comprehensive as FastAPI's, but it still provides ample resources for learning and using the framework.
Streamlit’s documentation focuses on data science use cases, providing detailed examples and tutorials for creating interactive data visualizations and machine learning models. Streamlit's documentation is not as comprehensive as FastAPI or Flask's, but still provides ample resources for learning and using the framework.
Extensibility
FastAPI supports third-party libraries and plugins to add functionality such as databases, authentication, and testing frameworks. FastAPI's built-in support for dependencies makes it easy to manage shared data and resources.
Flask’s extensibility is one of its strengths, with a large number of third-party extensions for adding functionality such as database access, form validation, and RESTful API development. Flask's minimalist design allows developers to add only the functionality they need.
Streamlit also supports third-party extensions for adding functionality, such as advanced data visualization and geospatial data support. Streamlit's focus on data science use cases limits the number of third-party extensions available, but there are still ample resources available for creating interactive data visualizations and machine learning models.
Data Science Features
FastAPI provides support for asynchronous programming and data validation and serialization using the Pydantic library. While FastAPI can be used in data science applications, it is not specifically designed for this use case.
Flask support for data science applications comes from third-party extensions such as SQLAlchemy for database access and Flask-RESTful for building RESTful APIs. Flask is not specifically designed for data science use cases.
Streamlit is designed for data science use cases, with built-in support for Python’s data science stack, including NumPy, Pandas, Matplotlib, and Scikit-learn. Streamlit’s API is designed to create interactive data visualizations and machine learning models with minimal coding.
Conclusion
FastAPI, Flask, and Streamlit are all excellent Python frameworks for web development and data science. Each framework has its advantages and disadvantages depending on the use case and project requirements.
FastAPI is best suited for high-performance web applications that need to support asynchronous programming as well as data validation and serialization. FastAPI's speed and performance make it an excellent choice for large web applications.
Flask is best suited for small to medium-sized web applications that require minimalist design and scalability. Flask's simplicity and ease of use make it a great choice for beginners and developers who want to build web applications quickly.
Streamlit is best suited for data science use cases that require interactive data visualization and machine learning models. Streamlit's high-level API and focus on interactivity and responsiveness make it an excellent choice for data scientists and developers who want to create interactive data visualizations and machine learning models with minimal code.
Ultimately, the choice of framework depends on project requirements and developer preference. FastAPI, Flask, and Streamlit are all great choices for Python web development and data science.
The above is the detailed content of Comparison of FastAPI, Flask and Streamlit for Web Development. For more information, please follow other related articles on the PHP Chinese website!
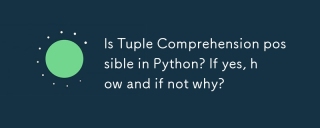
Article discusses impossibility of tuple comprehension in Python due to syntax ambiguity. Alternatives like using tuple() with generator expressions are suggested for creating tuples efficiently.(159 characters)
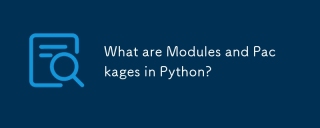
The article explains modules and packages in Python, their differences, and usage. Modules are single files, while packages are directories with an __init__.py file, organizing related modules hierarchically.
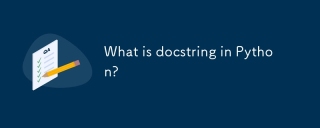
Article discusses docstrings in Python, their usage, and benefits. Main issue: importance of docstrings for code documentation and accessibility.
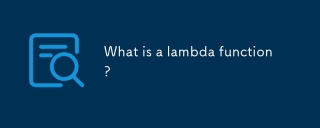
Article discusses lambda functions, their differences from regular functions, and their utility in programming scenarios. Not all languages support them.
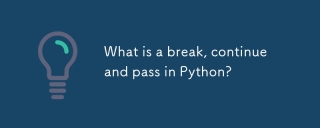
Article discusses break, continue, and pass in Python, explaining their roles in controlling loop execution and program flow.
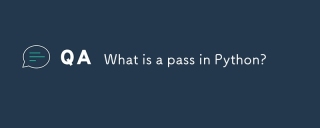
The article discusses the 'pass' statement in Python, a null operation used as a placeholder in code structures like functions and classes, allowing for future implementation without syntax errors.
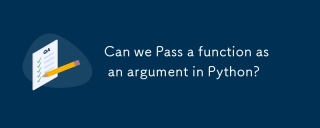
Article discusses passing functions as arguments in Python, highlighting benefits like modularity and use cases such as sorting and decorators.
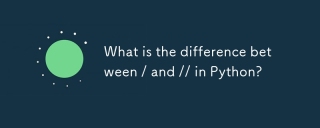
Article discusses / and // operators in Python: / for true division, // for floor division. Main issue is understanding their differences and use cases.Character count: 158


Hot AI Tools

Undresser.AI Undress
AI-powered app for creating realistic nude photos

AI Clothes Remover
Online AI tool for removing clothes from photos.

Undress AI Tool
Undress images for free

Clothoff.io
AI clothes remover

Video Face Swap
Swap faces in any video effortlessly with our completely free AI face swap tool!

Hot Article

Hot Tools

MantisBT
Mantis is an easy-to-deploy web-based defect tracking tool designed to aid in product defect tracking. It requires PHP, MySQL and a web server. Check out our demo and hosting services.
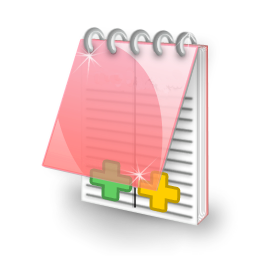
EditPlus Chinese cracked version
Small size, syntax highlighting, does not support code prompt function

SublimeText3 Chinese version
Chinese version, very easy to use
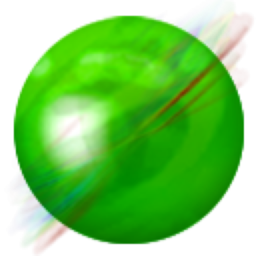
ZendStudio 13.5.1 Mac
Powerful PHP integrated development environment

SecLists
SecLists is the ultimate security tester's companion. It is a collection of various types of lists that are frequently used during security assessments, all in one place. SecLists helps make security testing more efficient and productive by conveniently providing all the lists a security tester might need. List types include usernames, passwords, URLs, fuzzing payloads, sensitive data patterns, web shells, and more. The tester can simply pull this repository onto a new test machine and he will have access to every type of list he needs.
