


New method of 3D model segmentation frees your hands! No manual labeling is required, only one training is required, and unlabeled categories can also be recognized | HKU & Byte
No manual annotation is required, and only one training is required to allow the 3D model to understand the language and identify unlabeled categories.
3D model segmentation is now hands-free!
The University of Hong Kong and ByteDream have collaborated and come up with a new method:
No manual annotation is required, and only one training is needed to allow the 3D model to understand the language and identify unknown objects. labeled categories.
For example, look at the following example, unannotated blackboard and monitor. After the 3D model is trained by this method, it can quickly "grasp" the target for segmentation.
For another example, if you enter synonyms such as sofa and cough to make things difficult for it, it can be easily won.
# Even abstract categories such as bathrooms can be solved.
This new method is called PLA (Point-Language Assocation), which is a method that combines point clouds (massive point collections of target surface properties) and natural language. .
Currently, this paper has been accepted by CVPR 2023.
But having said that, there is no need for manual annotation, only one training is performed, and synonym abstract classification can also be recognized... This is a superposition of multiple buffs.
You must know that the 3D data and natural language used by the general method cannot be obtained directly from the Internet for free, and often require expensive manual annotation, and the general method cannot be based on the semantic relationship between words. Identify new categories.
So how does PLA do it? Let’s take a look~
Specific principles
In fact, to put it bluntly, the most important step to successfully achieve 3D model division is to make the 3D data understand natural language.
Professionally speaking, it is to introduce natural language descriptions to 3D point clouds.
How to introduce it?
In view of the fact that there are currently relatively successful methods for dividing 2D images, the research team decided to start with 2D images.
First, convert the 3D point cloud into the corresponding 2D image, and then use it as the input of the 2D multi-modal large model, and extract the language description of the image from it.
Next, using the projection relationship between the image and the point cloud, the language description of the image can naturally be associated with the 3D point cloud data.
Moreover, in order to be compatible with 3D objects of different granularities, PLA also proposes a multi-granularity 3D point cloud-natural language association method.
For the entire 3D scene, PLA summarizes the language descriptions extracted from all images corresponding to the scene, and uses this summarized language to associate the entire 3D scene.
For the part of the 3D scene corresponding to each image view, PLA directly uses the image as a bridge to associate the corresponding 3D point cloud and language.
For more fine-grained 3D objects, PLA provides a more detailed method by comparing the intersection and union between corresponding point clouds of different images, as well as the intersection and union of the language description part. Granular 3D-language correlation approach.
In this way, the research team can obtain pairs of 3D point clouds-natural language, which directly solves the problem of manual annotation.
PLA uses the obtained "3D point cloud-natural language" pair and existing data set supervision to allow the 3D model to understand the detection and segmentation problem definition.
Specifically, contrastive learning is used to shorten the distance between each pair of 3D point clouds and natural language in the feature space, and to push away the mismatched 3D point clouds and natural language descriptions. .
Having talked about so many principles, how does PLA perform in specific segmentation tasks?
The semantic segmentation task exceeded the baseline by 65%
The researchers tested the performance of the 3D open world model in unlabeled categories as the main measurement criterion.
First, in the semantic segmentation tasks of ScanNet and S3DIS, PLA exceeded the previous baseline method by 35% to 65%.
In the instance segmentation task, PLA has also been improved. Compared with the previous method, the PLA improvement ranges from 15% to 50%.
Research Team
The research team for this project comes from the CVMI Lab of the University of Hong Kong and ByteDance.
CVMI Lab is an artificial intelligence laboratory of the University of Hong Kong. The laboratory was established on February 1, 2020.
The research scope covers computer vision and pattern recognition, machine learning/deep learning, image/video content analysis and industrial big data analysis based on machine intelligence.
Paper address:https://arxiv.org/pdf/2211.16312.pdf
Project home page:https: //github.com/CVMI-Lab/PLA
The above is the detailed content of New method of 3D model segmentation frees your hands! No manual labeling is required, only one training is required, and unlabeled categories can also be recognized | HKU & Byte. For more information, please follow other related articles on the PHP Chinese website!
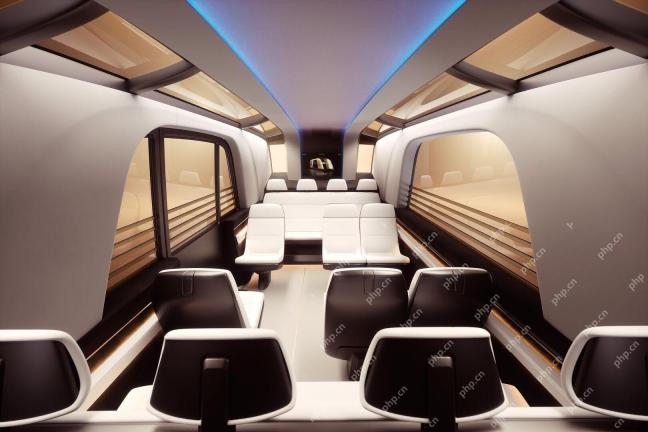
Since 2008, I've championed the shared-ride van—initially dubbed the "robotjitney," later the "vansit"—as the future of urban transportation. I foresee these vehicles as the 21st century's next-generation transit solution, surpas
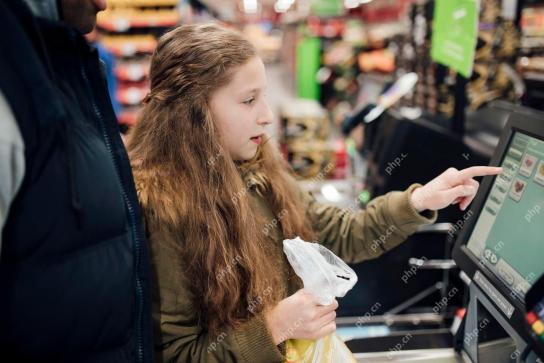
Revolutionizing the Checkout Experience Sam's Club's innovative "Just Go" system builds on its existing AI-powered "Scan & Go" technology, allowing members to scan purchases via the Sam's Club app during their shopping trip.
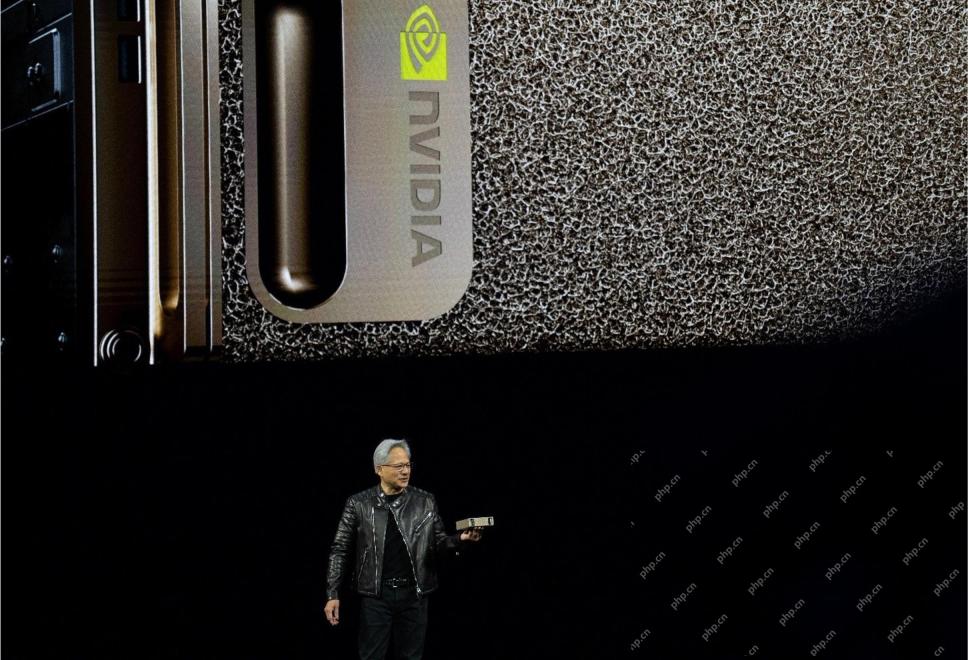
Nvidia's Enhanced Predictability and New Product Lineup at GTC 2025 Nvidia, a key player in AI infrastructure, is focusing on increased predictability for its clients. This involves consistent product delivery, meeting performance expectations, and
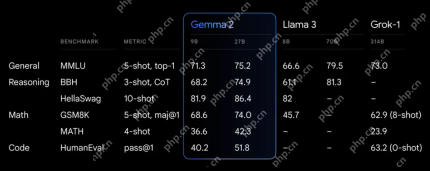
Google's Gemma 2: A Powerful, Efficient Language Model Google's Gemma family of language models, celebrated for efficiency and performance, has expanded with the arrival of Gemma 2. This latest release comprises two models: a 27-billion parameter ver
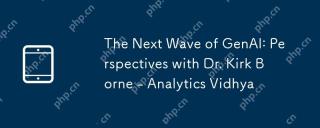
This Leading with Data episode features Dr. Kirk Borne, a leading data scientist, astrophysicist, and TEDx speaker. A renowned expert in big data, AI, and machine learning, Dr. Borne offers invaluable insights into the current state and future traje
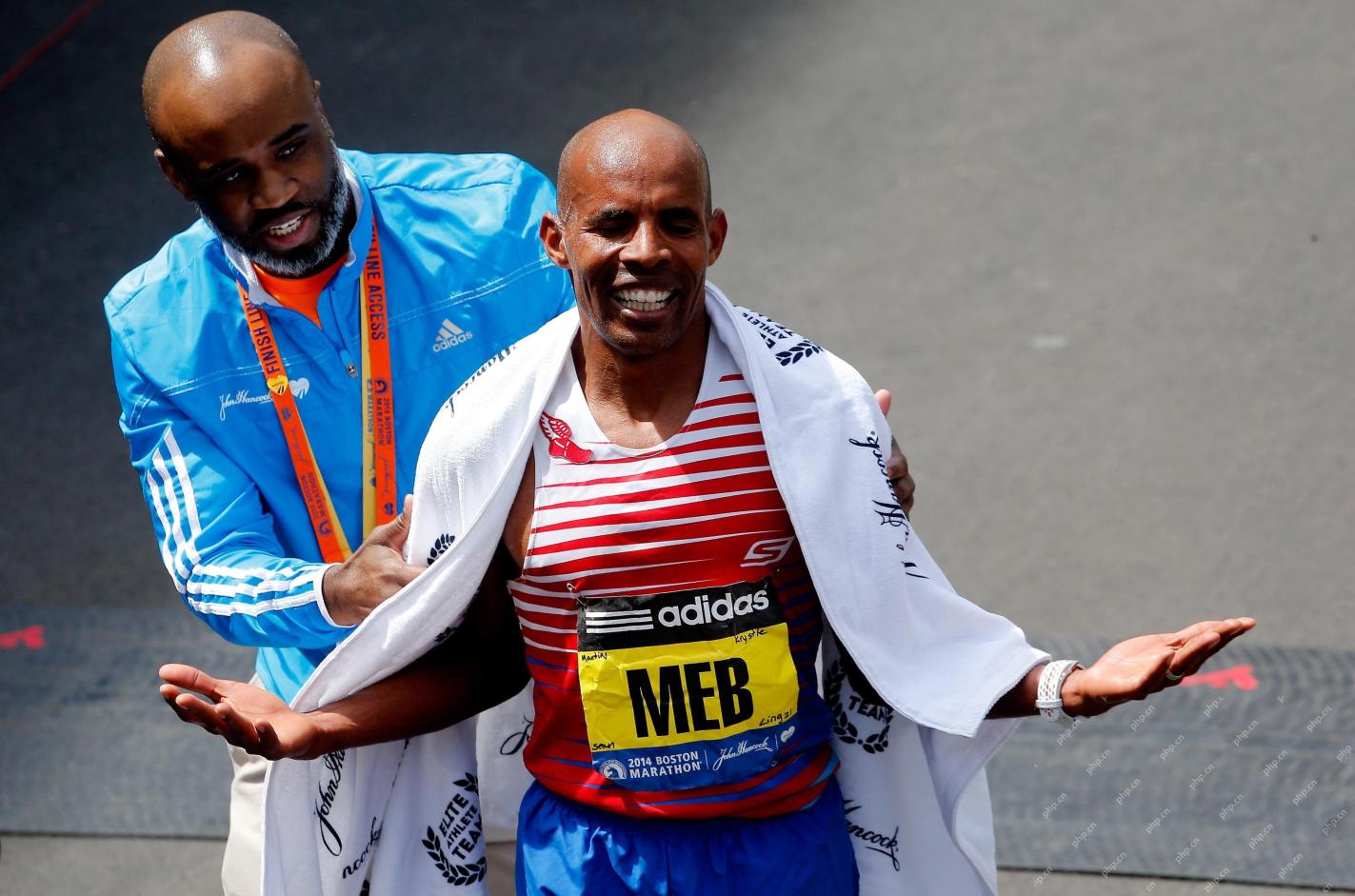
There were some very insightful perspectives in this speech—background information about engineering that showed us why artificial intelligence is so good at supporting people’s physical exercise. I will outline a core idea from each contributor’s perspective to demonstrate three design aspects that are an important part of our exploration of the application of artificial intelligence in sports. Edge devices and raw personal data This idea about artificial intelligence actually contains two components—one related to where we place large language models and the other is related to the differences between our human language and the language that our vital signs “express” when measured in real time. Alexander Amini knows a lot about running and tennis, but he still
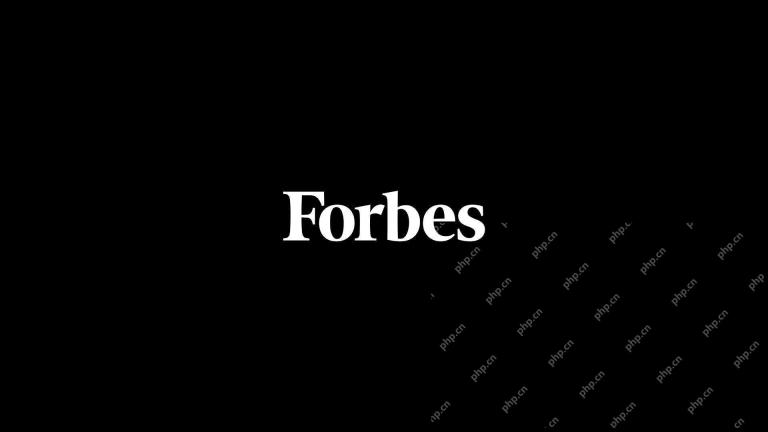
Caterpillar's Chief Information Officer and Senior Vice President of IT, Jamie Engstrom, leads a global team of over 2,200 IT professionals across 28 countries. With 26 years at Caterpillar, including four and a half years in her current role, Engst
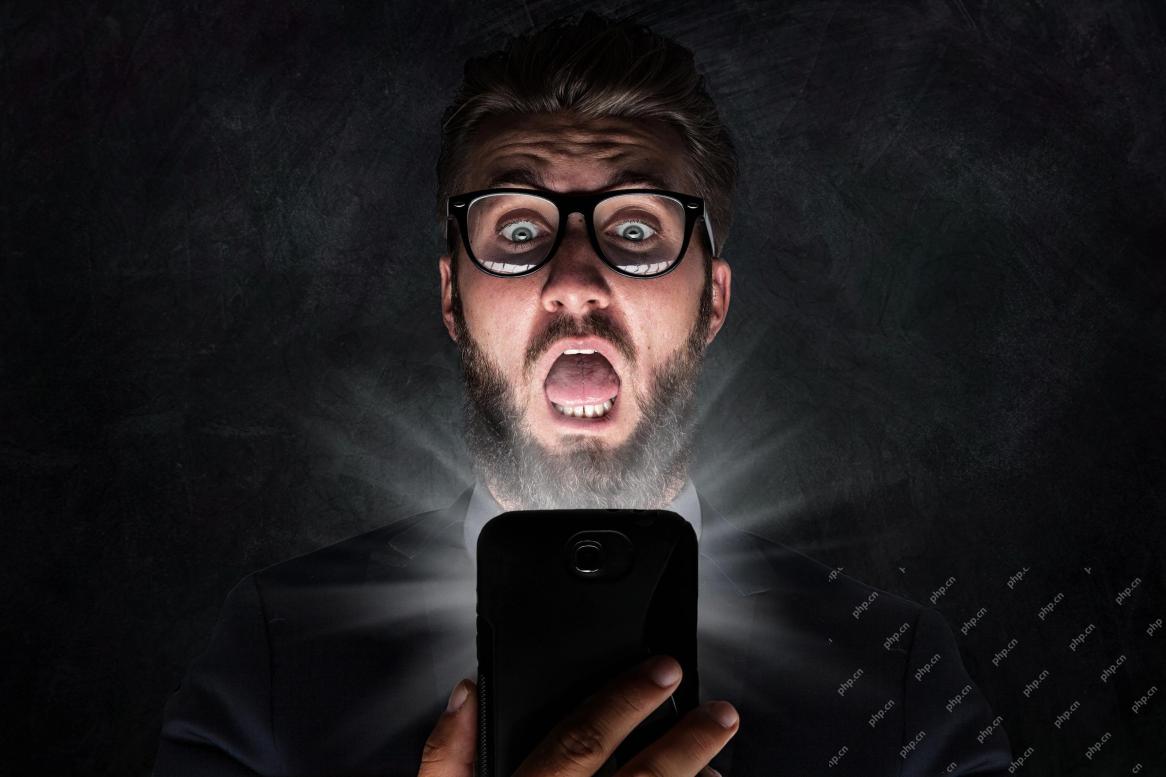
Google Photos' New Ultra HDR Tool: A Quick Guide Enhance your photos with Google Photos' new Ultra HDR tool, transforming standard images into vibrant, high-dynamic-range masterpieces. Ideal for social media, this tool boosts the impact of any photo,


Hot AI Tools

Undresser.AI Undress
AI-powered app for creating realistic nude photos

AI Clothes Remover
Online AI tool for removing clothes from photos.

Undress AI Tool
Undress images for free

Clothoff.io
AI clothes remover

Video Face Swap
Swap faces in any video effortlessly with our completely free AI face swap tool!

Hot Article

Hot Tools

Atom editor mac version download
The most popular open source editor

SublimeText3 Linux new version
SublimeText3 Linux latest version

mPDF
mPDF is a PHP library that can generate PDF files from UTF-8 encoded HTML. The original author, Ian Back, wrote mPDF to output PDF files "on the fly" from his website and handle different languages. It is slower than original scripts like HTML2FPDF and produces larger files when using Unicode fonts, but supports CSS styles etc. and has a lot of enhancements. Supports almost all languages, including RTL (Arabic and Hebrew) and CJK (Chinese, Japanese and Korean). Supports nested block-level elements (such as P, DIV),

Zend Studio 13.0.1
Powerful PHP integrated development environment

SecLists
SecLists is the ultimate security tester's companion. It is a collection of various types of lists that are frequently used during security assessments, all in one place. SecLists helps make security testing more efficient and productive by conveniently providing all the lists a security tester might need. List types include usernames, passwords, URLs, fuzzing payloads, sensitive data patterns, web shells, and more. The tester can simply pull this repository onto a new test machine and he will have access to every type of list he needs.