


The most important difference between ChatGPT and GPT-4 is that building on GPT-4 predicts the next character with higher accuracy. The better a neural network can predict the next word in a text, the better it can understand the text.
produced by Big Data Digest
Author: Caleb
What kind of sparks will Nvidia create when it encounters OpenAI?
Just now, Nvidia founder and CEO Huang Jensen had an in-depth exchange with OpenAI co-founder Ilya Sutskever during a GTC fireside chat.
Video link:
https://www.nvidia.cn/gtc-global/session-catalog/?tab.catalogallsessinotallow=16566177511100015Kus #/session/1669748941314001t6Nv
Two days ago, OpenAI launched the most powerful artificial intelligence model to date, GPT-4. OpenAI calls GPT-4 "OpenAI's most advanced system" on its official website and "can produce safer and more useful responses."
Sutskever also said during the talk that GPT-4 marks "considerable improvements" in many aspects compared to ChatGPT, noting that the new model can read images and text. "In some future version, [users] may get a chart" in response to questions and inquiries, he said.
There is no doubt that with the popularity of ChatGPT and GPT-4 on a global scale, this has also become the focus of this conversation. In addition to GPT-4 and its predecessors including ChatGPT related topics, Huang Renxun and Sutskever also talked about the capabilities, limitations and internal operations of deep neural networks, as well as predictions for future AI development.
Let’s take a closer look at this conversation with Digest Fungus~
Start when no one cares about network scale and computing scale
There may be many people When I hear Sutskever's name, the first thing that comes to mind is OpenAI and its related AI products, but you must know that Sutskever's resume can be traced back to Andrew Ng's postdoc, Google Brain research scientist, and co-developer of the Seq2Seq model.
It can be said that from the beginning, deep learning has been bound to Sutskever.
When talking about his understanding of deep learning, Sutskever said that from now on, deep learning has indeed changed the world. However, his personal starting point lies more in his intuition about the huge impact potential of AI, his strong interest in consciousness and human experience, and his belief that the development of AI will help answer these questions.
During 2002-03, people generally believed that learning was something that only humans could do, and that computers could not learn. And if computers can be given the ability to learn, it will be a major breakthrough in the field of AI.
This also became an opportunity for Sutskever to officially enter the AI field.
So Sutskever found Jeff Hinton from the same university. In his view, the neural network Hinton is working on is the breakthrough, because the characteristics of neural networks lie in parallel computers that can learn and be programmed automatically.
At that time, no one cared about the importance of network scale and calculation scale. People trained only 50 or 100 neural networks, and hundreds of them were already considered large, with one million parameters. Also considered huge.
In addition, they can only run programs on unoptimized CPU code, because no one understands BLAS. They use optimized Matlab to do some experiments, such as what kind of questions to use to ask and compare. good.
But the problem is that these are very scattered experiments and cannot really promote technological progress.
Building a neural network for computer vision
At that time, Sutskever realized that supervised learning was the way forward in the future.
This is not only an intuition, but also an undisputed fact. If the neural network is deep enough and large enough, it will have the ability to solve some difficult tasks. But people have not yet focused on deep and large neural networks, or even focused on neural networks at all.
In order to find a good solution, a suitably large data set and a lot of calculations are needed.
ImageNet is that data. At that time, ImageNet was a very difficult data set, but to train a large convolutional neural network, you must have matching computing power.
Next it’s time for the GPU to appear. Under the suggestion of Jeff Hinton, they found that with the emergence of the ImageNet data set, the convolutional neural network is a very suitable model for GPU, so it can be made very fast and the scale is getting larger and larger.
Subsequently, it directly and significantly broke the record of computer vision. This is not based on the continuation of previous methods. The key lies in the difficulty and scope of the data set itself.
OpenAI: From 100 People to ChatGPT
In the early days of OpenAI, Sutskever admitted that they were not entirely sure how to promote the project.
At the beginning of 2016, neural networks were not as developed and there were many fewer researchers than there are now. Sutskever recalled that there were only 100 people in the company at the time, and most of them were still working at Google or deepmind.
But they had two big ideas at the time.
One of them is unsupervised learning through compression. In 2016, unsupervised learning was an unsolved problem in machine learning, and no one knew how to implement it. Compression has not been a topic that people usually talk about recently, but suddenly everyone realized that GPT actually compresses the training data.
Mathematically speaking, training these autoregressive generative models compresses the data, and intuitively you can see why it works. If the data is compressed well enough, you can extract all the hidden information present in it. This also directly led to OpenAI’s related research on emotional neurons.
At the same time, when they tuned the same LSTM to predict the next character of an Amazon review, they found that if you predict the next character well enough, there will be a neuron within the LSTM that corresponds to its sentiment. This is a good demonstration of the effect of unsupervised learning and also verifies the idea of next character prediction.
But where do we get the data for unsupervised learning? The hard part about unsupervised learning, Sutskever said, is less about the data and more about why you're doing it, and realizing that training a neural network to predict the next character is worth pursuing and exploring. From there it learns an understandable representation.
Another big idea is reinforcement learning. Sutskever has always believed that bigger is better. At OpenAI, one of their goals is to figure out the right way to scale.
The first really big project OpenAI completed was the implementation of the strategy game Dota 2. At that time, OpenAI trained a reinforcement learning agent to fight against itself. The goal was to reach a certain level and be able to play games with human players.
The transformation from Dota's reinforcement learning to the reinforcement learning of human feedback combined with the GPT output technology base has become today's ChatGPT.
How OpenAI trains a large neural network
When training a large neural network to accurately predict the next word in different texts on the Internet, what OpenAI does is learn a world Model.
This looks like we are only learning statistical correlations in text, but in fact, learning these statistical correlations can compress this knowledge very well. What the neural network learns are some expressions in the process of generating text. This text is actually a map of the world, so the neural network can learn more and more perspectives to view humans and society. These are what the neural network really learns in the task of accurately predicting the next word.
At the same time, the more accurate the prediction of the next word, the higher the degree of restoration, and the higher the resolution of the world obtained in this process. This is the role of the pre-training phase, but it does not make the neural network behave the way we want it to behave.
What a language model is really trying to do is, if I had some random text on the internet, starting with some prefix or hint, what would it complete.
Of course it can also find text to fill in on the Internet, but this is not what was originally conceived, so additional training is required, which is fine-tuning, reinforcement learning from human teachers, and other forms of Where AI assistance can help.
But this is not about teaching new knowledge, but about communicating with it and conveying to it what we want it to be, which also includes boundaries. The better this process is done, the more useful and reliable the neural network will be, and the higher the fidelity of the boundaries will be.
Let’s talk about GPT-4 again
Not long after ChatGPT became the application with the fastest growing users, GPT-4 was officially released.
When talking about the differences between the two, Sutskever said that GPT-4 has achieved considerable improvements in many dimensions compared to ChatGPT.
The most important difference between ChatGPT and GPT-4 is that building on GPT-4 predicts the next character with higher accuracy. The better a neural network can predict the next word in a text, the better it can understand the text.
For example, you read a detective novel. The plot is very complex, with many plots and characters interspersed, and many mysterious clues buried. In the last chapter of the book, the detective collected all the clues, called everyone together, and said that now he will reveal who the culprit is, and that person is...
This is what GPT-4 can predict.
People say that deep learning cannot reason logically. But whether it is this example or some of the things that GPT can do, it shows a certain degree of reasoning ability.
Sutskever responded that when we are defining logical reasoning, if you can think about it in a certain way when making the next decision, you may be able to get a better answer. It remains to be seen how far neural networks can go, and OpenAI has not yet fully tapped its potential.
Some neural networks actually already have this kind of ability, but most of them are not reliable enough. Reliability is the biggest obstacle to making these models useful, and it is also a major bottleneck of current models. It’s not about whether the model has a specific capability, but how much capability it has.
Sutskever also said that GPT-4 did not have a built-in search function when it was released. It is just a good tool that can predict the next word, but it can be said that it fully has this ability and will make search more efficient. good.
Another significant improvement in GPT-4 is the response and processing of images. Multimodal learning plays an important role in it. Sutskever said that multimodality has two dimensions. The first is that multimodality is useful for neural networks, especially vision; the second is that in addition to text learning In addition, knowledge about the world can also be learned from images.
The future of artificial intelligence
When it comes to using AI to train AI, Sutskever said this part of the data should not be ignored.
It is difficult to predict the development of language models in the future, but in Sutskever’s view, there is good reason to believe that this field will continue to progress, and AI will continue to shock mankind with its strength at the boundaries of its capabilities. The reliability of AI is determined by whether it can be trusted, and it will definitely reach a point where it can be completely trusted in the future.
If it doesn’t fully understand, it will also ask questions to figure it out, or tell you that it doesn’t know. These are the areas where AI usability has the greatest impact and will see the greatest progress in the future.
Now we are faced with such a challenge, you want a neural network to summarize a long document or obtain a summary, how to ensure that important details have not been overlooked? If a point is obviously important enough that every reader will agree on it, then the content summarized by the neural network can be accepted as reliable.
The same applies to whether the neural network clearly follows the user's intent.
We will see more and more of this technology in the next two years, making this technology more and more reliable.
Related reports:https://blogs.nvidia.com/blog/2023/03/22/sutskever-openai-gtc/
The above is the detailed content of What improvement does GPT-4 have over ChatGPT? Jen-Hsun Huang held a 'fireside chat' with OpenAI co-founder. For more information, please follow other related articles on the PHP Chinese website!
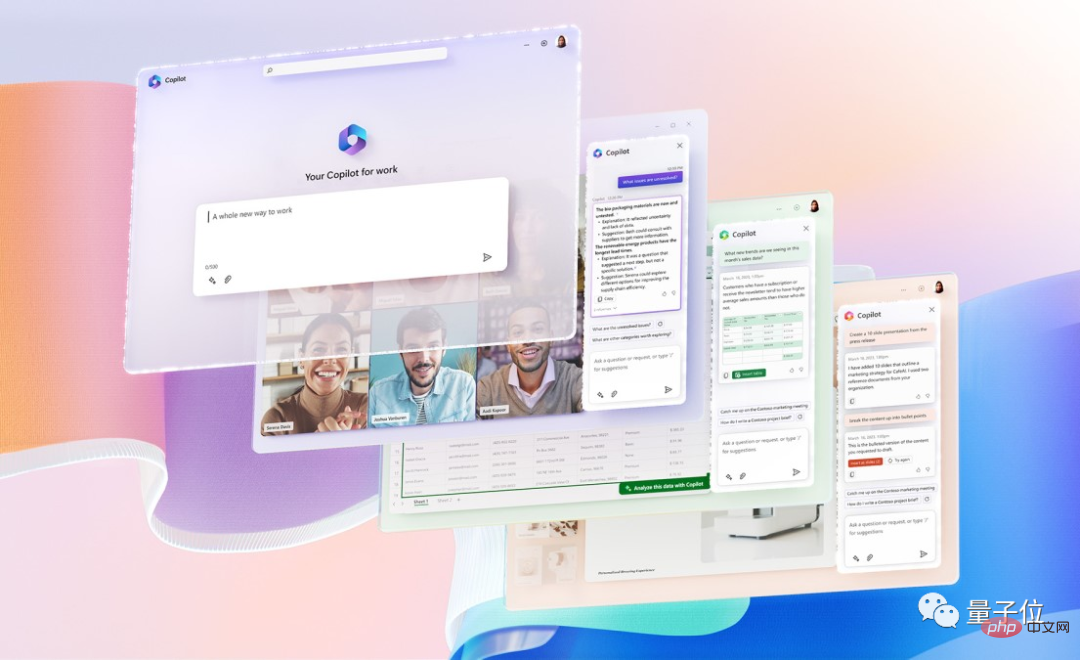
一觉醒来,工作的方式被彻底改变。微软把AI神器GPT-4全面接入Office,这下ChatPPT、ChatWord、ChatExcel一家整整齐齐。CEO纳德拉在发布会上直接放话:今天,进入人机交互的新时代,重新发明生产力。新功能名叫Microsoft 365 Copilot(副驾驶),与改变了程序员的代码助手GitHub Copilot成为一个系列,继续改变更多人。现在AI不光能自动做PPT,而且能根据Word文档的内容一键做出精美排版。甚至连上台时对着每一页PPT应该讲什么话,都给一起安排

集成GPT-4的Github Copilot X还在小范围内测中,而集成GPT-4的Cursor已公开发行。Cursor是一个集成GPT-4的IDE,可以用自然语言编写代码,让编写代码和聊天一样简单。 GPT-4和GPT-3.5在处理和编写代码的能力上差别还是很大的。官网的一份测试报告。前两个是GPT-4,一个采用文本输入,一个采用图像输入;第三个是GPT3.5,可以看出GPT-4的代码能力相较于GPT-3.5有较大能力的提升。集成GPT-4的Github Copilot X还在小范围内测中,而
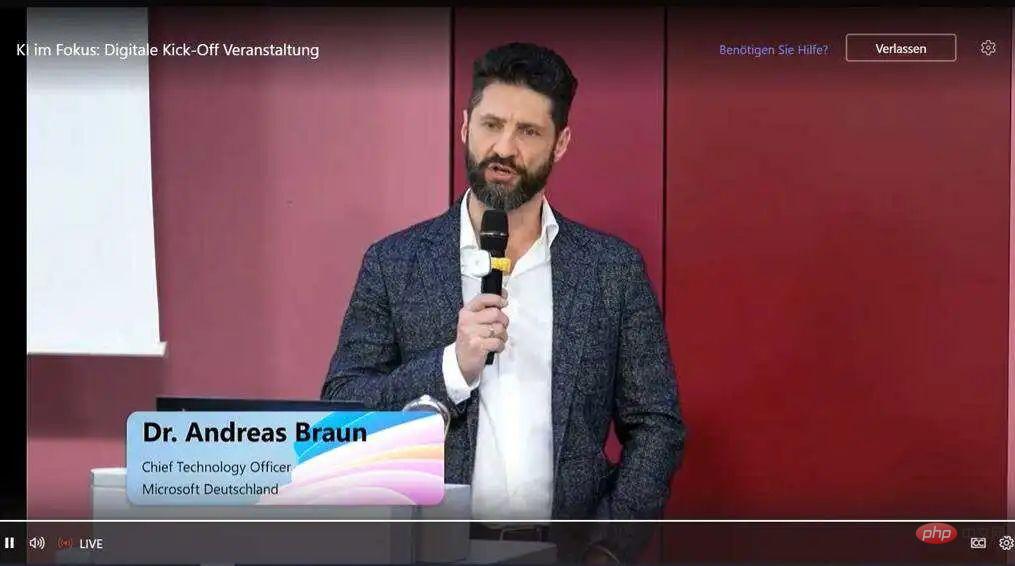
作者 | 云昭3月9日,微软德国CTO Andreas Braun在AI kickoff会议上带来了一个期待已久的消息:“我们将于下周推出GPT-4,届时我们将推出多模式模式,提供完全不同的可能性——例如视频。”言语之中,他将大型语言模型(LLM)比作“游戏改变者”,因为他们教机器理解自然语言,然后机器以统计的方式理解以前只能由人类阅读和理解的东西。与此同时,这项技术已经发展到“适用于所有语言”:你可以用德语提问,也可以用意大利语回答。借助多模态,微软(-OpenAI)将“使模型变得全面”。那
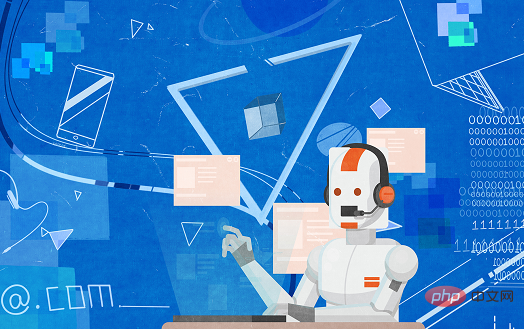
近段时间,人工智能聊天机器人ChatGPT刷爆网络,网友们争先恐后去领略它的超高情商和巨大威力。参加高考、修改代码、构思小说……它在广大网友的“鞭策”下不断突破自我,甚至可以用一整段程序,为你拼接出一只小狗。而这些技能只是基于GPT-3.5开发而来,在3月15日,AI世界再次更新,最新版本的GPT-4也被OpenAI发布了出来。与之前相比,GPT-4不仅展现了更加强大的语言理解能力,还能够处理图像内容,在考试中的得分甚至能超越90%的人类。那么,如此“逆天”的GPT-4还具有哪些能力?它又是如何
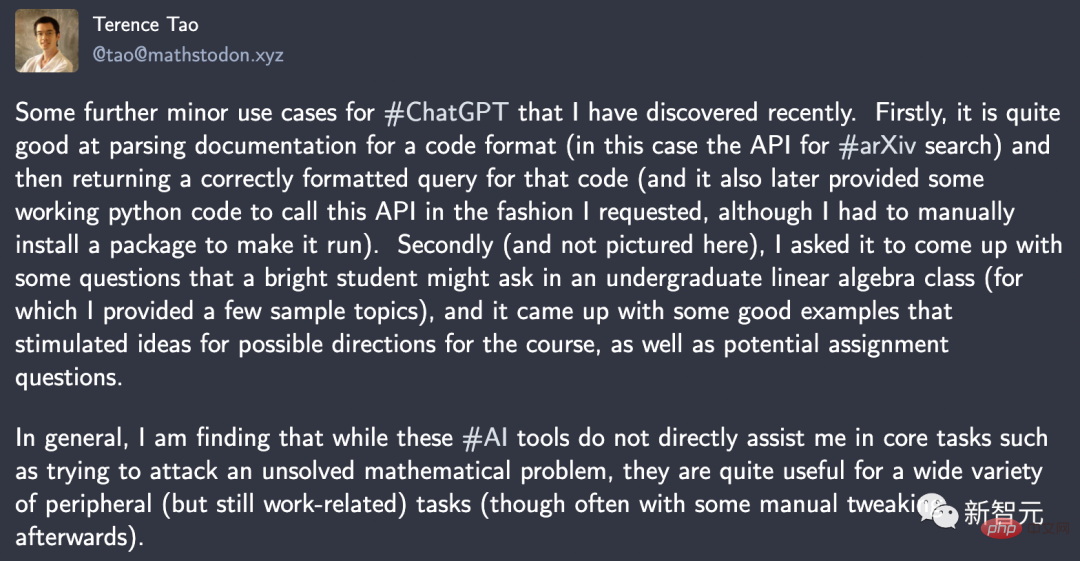
当红炸子鸡ChatGPT,也成为数学天才陶哲轩的研究工具了。近日,他在网上称自己发现了一些ChatGPT的小用例。首先,它很擅长解析代码格式的文档(在这种情况下是#arXiv搜索的API),然后返回一个正确格式的代码查询(后来它还提供了一些工作的python代码,以我要求的方式调用这个API,尽管我不得不手动安装一个包来使它运行)。其次,我让它想出一些,聪明的学生在本科线性代数课上可能会问的问题(为此我提供了一些样本题目),它给出了一些很好的例子,让我对课程可能方向,以及潜在的作业问题有所启发。
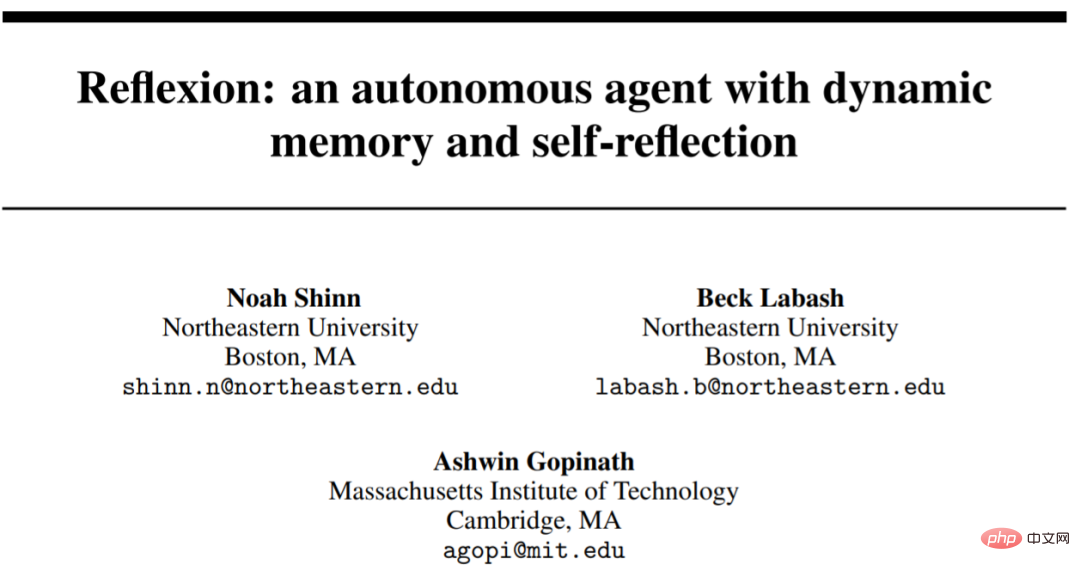
GPT-4 的思考方式,越来越像人了。 人类在做错事时,会反思自己的行为,避免再次出错,如果让 GPT-4 这类大型语言模型也具备反思能力,性能不知道要提高多少了。众所周知,大型语言模型 (LLM) 在各种任务上已经表现出前所未有的性能。然而,这些 SOTA 方法通常需要对已定义的状态空间进行模型微调、策略优化等操作。由于缺乏高质量的训练数据、定义良好的状态空间,优化模型实现起来还是比较难的。此外,模型还不具备人类决策过程所固有的某些品质,特别是从错误中学习的能力。不过现在好了,在最近的一篇论文
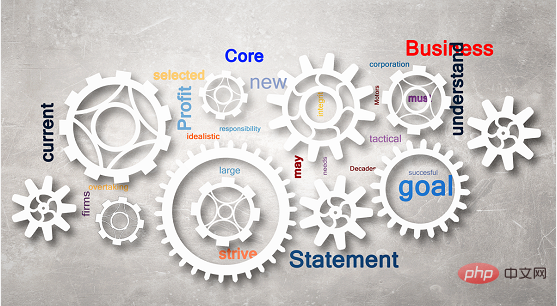
3 月 15 日消息,今天 OpenAI 发布了全新的 GPT-4 大型语言模型,随后微软官方宣布,Bing Chat 此前已经升级使用 OpenAI 的 GPT-4 技术。微软公司副总裁兼消费者首席营销官 Yusuf Mehdi 确认 Bing Chat 聊天机器人 AI 已经在 GPT-4 上运行,ChatGPT 基于最新版本 GPT-4,由 OpenAI 开发 。微软 Bing 博客网站上的一篇帖子进一步证实了这一消息。微软表示,如果用户在过去五周内的任何时间使用过新的 Bing 预览版,
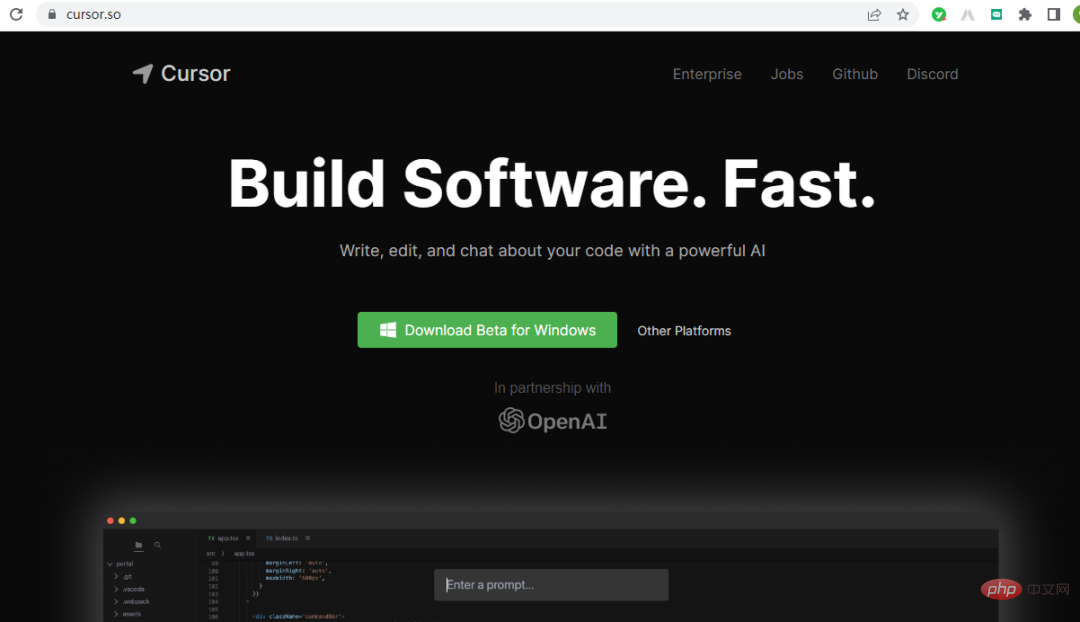
目前 Cursor 已经开源在 GitHub 上,已斩获了 9000+ GitHub Star,并成功登上 GitHub Trending。 最近一款名为Cursor的代码编辑器已经传遍了圈内,受到众多编程爱好者的追捧。它主打的亮点就是,通过 GPT-4 来辅助你编程,完成 AI 智能生成代码、修改 Bug、生成测试等操作。确实很吸引人,而且貌似也能大大节省人为的重复工作,让广大码农把有限的时间放在无限的需求构思上!目前 Cursor 已经开源在 GitHub 上,已斩获了 9000+ GitH


Hot AI Tools

Undresser.AI Undress
AI-powered app for creating realistic nude photos

AI Clothes Remover
Online AI tool for removing clothes from photos.

Undress AI Tool
Undress images for free

Clothoff.io
AI clothes remover

AI Hentai Generator
Generate AI Hentai for free.

Hot Article

Hot Tools

SAP NetWeaver Server Adapter for Eclipse
Integrate Eclipse with SAP NetWeaver application server.
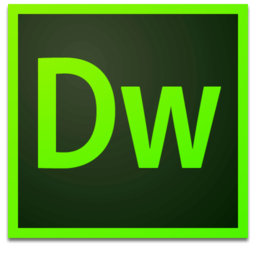
Dreamweaver Mac version
Visual web development tools
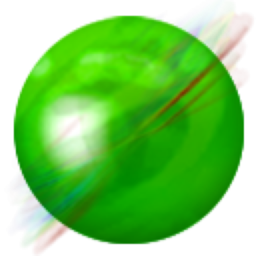
ZendStudio 13.5.1 Mac
Powerful PHP integrated development environment

Atom editor mac version download
The most popular open source editor

SublimeText3 Linux new version
SublimeText3 Linux latest version
