What are the four essential common sense for getting started with big data?
Four essential common sense for getting started with big data
A very important job for big data engineers is to pass Analyze data to find characteristics of past events. For example, Tencent's data team is building a data warehouse to sort out the large and irregular data information on all the company's network platforms and summarize the characteristics that can be queried to support the data needs of the company's various businesses, including advertising. placement, game development, social networking, etc.
1. Five basic aspects of big data analysis
1. Visual analysis
Users of big data analysis include big data analysis experts. There are also ordinary users, but their most basic requirement for big data analysis is visual analysis, because visual analysis can intuitively present the characteristics of big data and can be easily accepted by readers. It is as simple and clear as looking at pictures and talking. .
2. Data mining algorithm
The theoretical core of big data analysis is the data mining algorithm. Various data mining algorithms are based on different data types and formats to more scientifically present the data itself. It is precisely because of these various statistical methods (which can be called truth) recognized by statisticians around the world that they can go deep into the data and unearth recognized values. Another aspect is that these data mining algorithms can process big data more quickly. If an algorithm takes several years to reach a conclusion, then the value of big data will be impossible to say.
3. Predictive analysis capabilities
One of the final application fields of big data analysis is predictive analysis. It is to mine characteristics from big data and scientifically establish models, and then you can Bring in new data through the model to predict future data.
4. Semantic engine
Big data analysis is widely used in network data mining. It can analyze and judge user needs from the user's search keywords, tag keywords, or other input semantics, so as to Achieve better user experience and advertising matching.
5. Data quality and data management
Big data analysis is inseparable from data quality and data management. High-quality data and effective data management, whether in academic research or commercial applications field, can ensure that the analysis results are true and valuable. The basis of big data analysis is the above five aspects. Of course, if you go deeper into big data analysis, there are many more distinctive, in-depth, and professional big data analysis methods.
2. How to choose suitable data analysis tools
To understand what data to analyze, there are four main types of data to be analyzed in big data:
TRANSACTION DATA
The big data platform can obtain structured transaction data with a larger time span and a larger amount, so that a wider range of transaction data types can be analyzed, not only POS or E-commerce shopping data also includes behavioral transaction data, such as Internet click stream data logs recorded by web servers.
HUMAN-GENERATED DATA
Unstructured data widely exists in emails, documents, pictures, audios, videos, as well as data generated through blogs, wikis, especially social media flow. This data provides a rich source of data for analysis using text analytics capabilities.
MOBILE DATA
Smartphones and tablets that can access the Internet are becoming more and more common. Apps on these mobile devices are capable of tracking and communicating countless events, from in-app transaction data (such as recording a search for a product) to profile or status reporting events (such as a location change reporting a new geocode).
MACHINE AND SENSOR DATA
This includes data created or generated by functional devices, such as smart meters, smart temperature controllers, factory machines and internet-connected home appliances. These devices can be configured to communicate with other nodes in the internetwork and can also automatically transmit data to a central server so the data can be analyzed. Machine and sensor data are prime examples arising from the emerging Internet of Things (IoT). Data from the IoT can be used to build analytical models, continuously monitor predictive behavior (such as identifying when sensor values indicate a problem), and provide prescribed instructions (such as alerting technicians to inspect equipment before an actual problem occurs).
Related recommendations: "FAQ"
3. How to distinguish three popular professions of big data - data scientist, data engineer, data analyst
As big data becomes more and more popular, careers related to big data have also become popular, bringing many opportunities for talent development. Data scientists, data engineers, and data analysts have become the most popular positions in the big data industry. How are they defined? What exactly does it do? What skills are required? Let’s take a look.
How are these three professions positioned?
What kind of existence is a data scientist
Data scientists refer to engineers or engineers who can use scientific methods and data mining tools to digitally reproduce and understand complex and large amounts of numbers, symbols, text, URLs, audio or video information, and can find new data insights. Expert (different from statistician or analyst).
How data engineers are defined
Data engineers are generally defined as “star software engineers who have a deep understanding of statistics.” If you're struggling with a business problem, you need a data engineer. Their core value lies in their ability to create data pipelines from clean data. Fully understanding file systems, distributed computing and databases are necessary skills to become an excellent data engineer.
Data engineers have a fairly good understanding of algorithms. Therefore, data engineers should be able to run basic data models. The high-end business needs have given rise to the need for highly complex calculations. Many times, these needs exceed the scope of the data engineer's knowledge. At this time, you need to call a data scientist for help.
How to understand data analysts
Data analysts refer to people in different industries who specialize in collecting, sorting, and analyzing industry data, and making industry decisions based on the data. Professionals who research, evaluate and forecast. They know how to ask the right questions and are very good at data analysis, data visualization and data presentation.
What are the specific responsibilities of these three professions
The job responsibilities of data scientists
Data scientists tend to look at the surrounding world by exploring data. world. To turn a large amount of scattered data into structured data that can be analyzed, it is also necessary to find rich data sources, integrate other possibly incomplete data sources, and clean up the resulting data set. In the new competitive environment, challenges are constantly changing and new data are constantly flowing in. Data scientists need to help decision makers shuttle through various analyses, from temporary data analysis to continuous data interaction analysis. When they make discoveries, they communicate their findings and suggest new business directions. They present visual information creatively and make the patterns they find clear and convincing. Suggest the patterns contained in the data to the Boss to influence products, processes and decisions.
Job responsibilities of data engineers
Analyzing history, predicting the future, and optimizing choices are the three most important tasks of big data engineers when "playing with data". Through these three lines of work, they help companies make better business decisions.
A very important job of big data engineers is to find out the characteristics of past events by analyzing data. For example, Tencent's data team is building a data warehouse to sort out the large and irregular data information on all the company's network platforms and summarize the characteristics that can be queried to support the data needs of the company's various businesses, including advertising. placement, game development, social networking, etc.
The biggest role of finding out the characteristics of past events is to help companies better understand consumers. By analyzing the user's past behavior trajectory, you can understand this person and predict his behavior.
By introducing key factors, big data engineers can predict future consumer trends. On Alimama’s marketing platform, engineers are trying to help Taobao sellers do business by introducing weather data. For example, if this summer is not hot, it is very likely that certain products will not sell as well as last year. In addition to air conditioners and fans, vests, swimsuits, etc. may also be affected. Then we will establish the relationship between weather data and sales data, find related categories, and alert sellers in advance to turn over inventory.
Depending on the business nature of different enterprises, big data engineers can achieve different purposes through data analysis. For Tencent, the simplest and most direct example that reflects the work of big data engineers is option testing (AB Test), which helps product managers choose between alternatives A and B. In the past, decision makers could only make judgments based on experience, but now big data engineers can conduct real-time tests on a large scale—for example, in the example of social network products, let half of the users see interface A and the other half use interface B to observe Statistics of click-through rates and conversion rates over a period of time will help the marketing department make the final choice.
Job Responsibilities of Data Analysts
The Internet itself has digital and interactive characteristics. This attribute has brought revolution to data collection, organization, and research. breakthrough. In the past, data analysts in the "atomic world" had to spend higher costs (funds, resources and time) to obtain data to support research and analysis. The richness, comprehensiveness, continuity and timeliness of data were much worse than in the Internet era.
Compared with traditional data analysts, what data analysts in the Internet era face is not a lack of data, but a surplus of data. Therefore, data analysts in the Internet era must learn to use technical means to perform efficient data processing. More importantly, data analysts in the Internet era must continue to innovate and make breakthroughs in data research methodologies.
As far as the industry is concerned, the value of data analysts is similar to this. As far as the news publishing industry is concerned, no matter in any era, whether media operators can accurately, detailedly and timely understand the audience situation and changing trends is the key to the success or failure of the media.
In addition, for content industries such as news and publishing, it is even more critical that data analysts can perform the function of content consumer data analysis, which is a key function that supports news and publishing organizations in improving customer service.
What skills do you need to master to engage in these 3 careers?
A. Skills that data scientists need to master
1, Computer Science
Generally speaking, most data scientists are required to have a professional background related to programming and computer science. Simply put, it is the skills related to large-scale parallel processing technologies such as Hadoop and Mahout and machine learning that are necessary for processing big data.
2. Mathematics, statistics, data mining, etc.
In addition to the literacy in mathematics and statistics, you also need to have the skills to use mainstream statistical analysis software such as SPSS and SAS. Among them, the open source programming language and its operating environment "R" for statistical analysis have attracted much attention recently. The strength of R is not only that it contains a rich statistical analysis library, but also has high-quality chart generation capabilities for visualizing results, which can be run through simple commands. In addition, it also has a package extension mechanism called CRAN (The Comprehensive R Archive Network). By importing the extension package, you can use functions and data sets that are not supported in the standard state.
3. Data visualization (Visualization)
The quality of information depends largely on its expression. It is very important for data scientists to analyze the meaning contained in the data composed of numerical lists, develop Web prototypes, and use external APIs to unify charts, maps, Dashboards and other services to visualize the analysis results. One of the skills.
B. Skills that data engineers need to master
1, Mathematics and statistics related background
The requirements for big data engineers are that they hope to have a background in statistics and mathematics Master's or doctoral degree. Data workers who lack theoretical background are more likely to enter a technical danger zone (Danger Zone) - a bunch of numbers can always produce some results according to different data models and algorithms, but if you don't know what they mean , it is not a truly meaningful result, and such a result can easily mislead you. Only with certain theoretical knowledge can we understand models, reuse models and even innovate models to solve practical problems.
2. Computer coding ability
Actual development capabilities and large-scale data processing capabilities are some necessary elements for a big data engineer. Because the value of much data comes from the process of mining, you have to do it yourself to discover the value of gold. For example, many records generated by people on social networks are now unstructured data. How to extract meaningful information from these clueless texts, voices, images and even videos requires big data engineers to dig out it themselves. Even in some teams, big data engineers' responsibilities are mainly business analysis, but they must also be familiar with the way computers process big data.
3. Knowledge of specific application fields or industries
A very important point in the role of big data engineer is that it cannot be separated from the market, because big data can only be generated when combined with applications in specific fields value. Therefore, experience in one or more vertical industries can help candidates accumulate knowledge of the industry, which will be of great help in becoming a big data engineer in the future. Therefore, this is also a more convincing bonus when applying for this position.
C. Skills that data analysts need to master
1. Understand business. The prerequisite for engaging in data analysis work is to understand the business, that is, be familiar with industry knowledge, company business and processes, and it is best to have your own unique insights. If you are divorced from industry knowledge and company business background, the results of the analysis will only be off-line. Kites don't have much use value.
2. Understand management. On the one hand, it is the requirement to build a data analysis framework. For example, to determine the analysis ideas, you need to use marketing, management and other theoretical knowledge to guide you. If you are not familiar with management theory, it will be difficult to build a data analysis framework, and subsequent data analysis will also be difficult to carry out. . On the other hand, the role is to provide instructive analysis suggestions based on data analysis conclusions.
3. Understand analysis. It refers to mastering the basic principles of data analysis and some effective data analysis methods, and being able to flexibly apply them to practical work in order to effectively carry out data analysis. Basic analysis methods include: comparative analysis, group analysis, cross analysis, structural analysis, funnel chart analysis, comprehensive evaluation analysis, factor analysis, matrix correlation analysis, etc. Advanced analysis methods include: correlation analysis, regression analysis, cluster analysis, discriminant analysis, principal component analysis, factor analysis, correspondence analysis, time series, etc.
4. Understand the tools. Refers to mastering common tools related to data analysis. Data analysis methods are theories, and data analysis tools are tools to implement the theory of data analysis methods. Facing increasingly large amounts of data, we cannot rely on calculators for analysis. We must rely on powerful data analysis tools to help us complete the data analysis work.
5. Understand design. Understanding design means using charts to effectively express the data analyst’s analytical views so that the analysis results are clear at a glance. The design of charts is a major subject, such as the selection of graphics, layout design, color matching, etc., all of which require mastering certain design principles.
4. A 9-step development plan for becoming a data scientist from a rookie
First of all, each company has different definitions of data scientists, and there is currently no unified definition. . But in general, a data scientist combines the skills of a software engineer with a statistician, and has significant industry knowledge invested in the area in which he or she wishes to work.
About 90% of data scientists have at least a college education, and even a Ph.D. and a Ph.D. degree, of course, in a very wide range of fields. Some recruiters even find that humanities majors have the needed creativity to teach others critical skills.
So, excluding a data science degree program (which is popping up like mushrooms at prestigious universities around the world), what steps do you need to take to become a data scientist?
Review You Mathematical and Statistical Skills
A good data scientist must be able to understand what the data is telling you, and to do this you must have a solid understanding of basic linear algebra, an understanding of algorithms, and statistical skills. Advanced math may be required in some specific situations, but this is a good place to start.
Understand the concept of machine learning
Machine learning is the next emerging word, but it is inextricably linked to big data. Machine learning uses artificial intelligence algorithms to transform data into value without explicit programming.
Learn to Code
A data scientist must know how to tweak the code to tell the computer how to analyze the data. Start with an open source language like Python.
Understand databases, data pools and distributed storage
Data is stored in databases, data pools or entire distributed networks. And how to build a repository of this data depends on how you access, use, and analyze this data. If you don’t have an overall architecture or advance planning when building your data storage, the consequences for you will be profound.
Learn data modification and data cleaning techniques
Data modification is the process of converting raw data into another format that is easier to access and analyze. Data cleansing helps eliminate duplicate and "bad" data. Both are essential tools in a data scientist’s toolbox.
Understand the basics of good data visualization and reporting
You don’t have to be a graphic designer, but you do need to have a solid understanding of how to create data reports that are easy for the layman to understand People like your manager or CEO can understand.
Add more tools to your toolbox
Once you have mastered the above skills, it is time to expand your data science toolbox to include Hadoop, R Language and Spark. Experience and knowledge of using these tools will put you above the large pool of data science job seekers.
Practice
How do you practice being a data scientist before you have a job in a new field? Use open source code to develop a project you love, enter competitions, Become a web job data scientist, attend a bootcamp, volunteer, or intern. The best data scientists will have experience and intuition in the field of data and be able to demonstrate their work to become candidates.
Become a member of the community
Follow thought leaders in your industry, read industry blogs and websites, participate, ask questions, and stay informed about current news and theory.
The above is the detailed content of What are the four essential common sense for getting started with big data?. For more information, please follow other related articles on the PHP Chinese website!

Hot AI Tools

Undresser.AI Undress
AI-powered app for creating realistic nude photos

AI Clothes Remover
Online AI tool for removing clothes from photos.

Undress AI Tool
Undress images for free

Clothoff.io
AI clothes remover

Video Face Swap
Swap faces in any video effortlessly with our completely free AI face swap tool!

Hot Article

Hot Tools

SAP NetWeaver Server Adapter for Eclipse
Integrate Eclipse with SAP NetWeaver application server.

Atom editor mac version download
The most popular open source editor
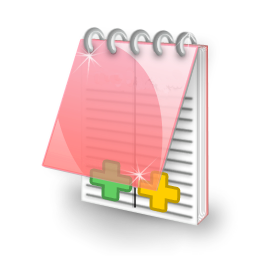
EditPlus Chinese cracked version
Small size, syntax highlighting, does not support code prompt function

SublimeText3 English version
Recommended: Win version, supports code prompts!

MantisBT
Mantis is an easy-to-deploy web-based defect tracking tool designed to aid in product defect tracking. It requires PHP, MySQL and a web server. Check out our demo and hosting services.
