


A brief discussion on tensorflow1.0 pooling layer (pooling) and fully connected layer (dense)
This article mainly introduces a brief discussion of the pooling layer (pooling) and fully connected layer (dense) of tensorflow 1.0. Now I will share it with you and give you a reference. Let’s take a look together
The pooling layer is defined in tensorflow/python/layers/pooling.py.
There are maximum pooling and mean pooling.
1. tf.layers.max_pooling2d
max_pooling2d( inputs, pool_size, strides, padding='valid', data_format='channels_last', name=None )
inputs: Pooled data.
pool_size: pooled core size (pool_height, pool_width), such as [3, 3]. If the length and width are equal, it can also be set directly to a number, such as pool_size=3.
strides: The sliding stride of pooling. It can be set to two integers like [1,1]. It can also be set directly to a number, such as strides=2
padding: edge padding, 'same' and 'valid' Choose one. The default is valid
data_format: Input data format, the default is channels_last, which is (batch, height, width, channels), it can also be set to channels_first corresponding to (batch, channels, height, width ).
name: The name of the layer.
Example:
pool1=tf.layers.max_pooling2d(inputs=x, pool_size=[2, 2], strides=2)
is usually placed after the convolutional layer, such as:
conv=tf.layers.conv2d( inputs=x, filters=32, kernel_size=[5, 5], padding="same", activation=tf.nn.relu) pool=tf.layers.max_pooling2d(inputs=conv, pool_size=[2, 2], strides=2)
2.tf.layers.average_pooling2d
average_pooling2d( inputs, pool_size, strides, padding='valid', data_format='channels_last', name=None )
The parameters are the same as the previous maximum pooling.
The fully connected dense layer is defined in tensorflow/python/layers/core.py.
3, tf.layers.dense
dense( inputs, units, activation=None, use_bias=True, kernel_initializer=None, bias_initializer=tf.zeros_initializer(), kernel_regularizer=None, bias_regularizer=None, activity_regularizer=None, trainable=True, name=None, reuse=None )
inputs: Input data, 2-dimensional tensor.
units: The number of neural unit nodes in this layer.
activation: activation function.
use_bias: Boolean type, whether to use the bias term.
kernel_initializer: The initializer of the convolution kernel.
bias_initializer: The initializer of the bias term, the default initialization is 0.
kernel_regularizer : Regularization of convolution kernel, optional.
bias_regularizer: Regularization of bias term, optional.
activity_regularizer: Output regularization function.
trainable: Boolean type, indicating whether the parameters of this layer participate in training. If true, the variable is added to the graph collection GraphKeys.TRAINABLE_VARIABLES (see tf.Variable).
name: The name of the layer.
reuse: Boolean type, whether to reuse parameters.
Fully connected layer execution operation outputs = activation(inputs.kernel bias)
If the execution result does not want to be activated, Then set activation=None.
Example:
#全连接层 dense1 = tf.layers.dense(inputs=pool3, units=1024, activation=tf.nn.relu) dense2= tf.layers.dense(inputs=dense1, units=512, activation=tf.nn.relu) logits= tf.layers.dense(inputs=dense2, units=10, activation=None)
You can also regularize the parameters of the fully connected layer:
Copy code The code is as follows:
dense1 = tf.layers.dense(inputs=pool3, units=1024, activation=tf.nn.relu,kernel_regularizer=tf.contrib.layers.l2_regularizer(0.003))
Related recommendations:
A brief discussion on saving and restoring the Tensorflow model
Detailed explanation of the three ways to load data into tensorflow
The above is the detailed content of A brief discussion on tensorflow1.0 pooling layer (pooling) and fully connected layer (dense). For more information, please follow other related articles on the PHP Chinese website!
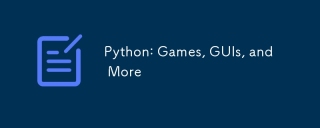
Python excels in gaming and GUI development. 1) Game development uses Pygame, providing drawing, audio and other functions, which are suitable for creating 2D games. 2) GUI development can choose Tkinter or PyQt. Tkinter is simple and easy to use, PyQt has rich functions and is suitable for professional development.
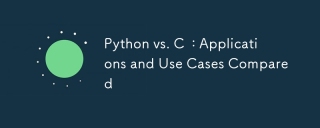
Python is suitable for data science, web development and automation tasks, while C is suitable for system programming, game development and embedded systems. Python is known for its simplicity and powerful ecosystem, while C is known for its high performance and underlying control capabilities.
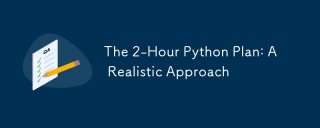
You can learn basic programming concepts and skills of Python within 2 hours. 1. Learn variables and data types, 2. Master control flow (conditional statements and loops), 3. Understand the definition and use of functions, 4. Quickly get started with Python programming through simple examples and code snippets.
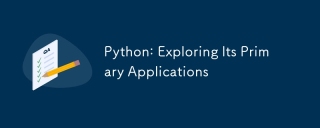
Python is widely used in the fields of web development, data science, machine learning, automation and scripting. 1) In web development, Django and Flask frameworks simplify the development process. 2) In the fields of data science and machine learning, NumPy, Pandas, Scikit-learn and TensorFlow libraries provide strong support. 3) In terms of automation and scripting, Python is suitable for tasks such as automated testing and system management.
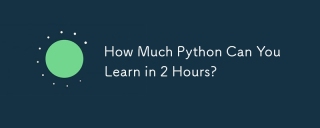
You can learn the basics of Python within two hours. 1. Learn variables and data types, 2. Master control structures such as if statements and loops, 3. Understand the definition and use of functions. These will help you start writing simple Python programs.
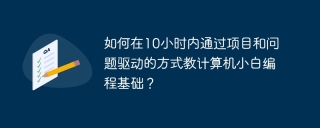
How to teach computer novice programming basics within 10 hours? If you only have 10 hours to teach computer novice some programming knowledge, what would you choose to teach...
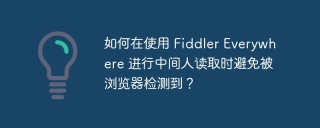
How to avoid being detected when using FiddlerEverywhere for man-in-the-middle readings When you use FiddlerEverywhere...
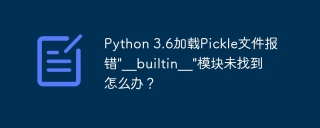
Error loading Pickle file in Python 3.6 environment: ModuleNotFoundError:Nomodulenamed...


Hot AI Tools

Undresser.AI Undress
AI-powered app for creating realistic nude photos

AI Clothes Remover
Online AI tool for removing clothes from photos.

Undress AI Tool
Undress images for free

Clothoff.io
AI clothes remover

AI Hentai Generator
Generate AI Hentai for free.

Hot Article

Hot Tools

SublimeText3 Chinese version
Chinese version, very easy to use

mPDF
mPDF is a PHP library that can generate PDF files from UTF-8 encoded HTML. The original author, Ian Back, wrote mPDF to output PDF files "on the fly" from his website and handle different languages. It is slower than original scripts like HTML2FPDF and produces larger files when using Unicode fonts, but supports CSS styles etc. and has a lot of enhancements. Supports almost all languages, including RTL (Arabic and Hebrew) and CJK (Chinese, Japanese and Korean). Supports nested block-level elements (such as P, DIV),

DVWA
Damn Vulnerable Web App (DVWA) is a PHP/MySQL web application that is very vulnerable. Its main goals are to be an aid for security professionals to test their skills and tools in a legal environment, to help web developers better understand the process of securing web applications, and to help teachers/students teach/learn in a classroom environment Web application security. The goal of DVWA is to practice some of the most common web vulnerabilities through a simple and straightforward interface, with varying degrees of difficulty. Please note that this software
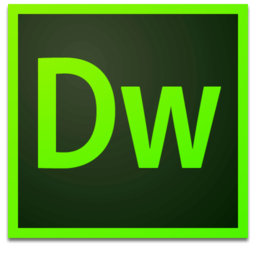
Dreamweaver Mac version
Visual web development tools

SecLists
SecLists is the ultimate security tester's companion. It is a collection of various types of lists that are frequently used during security assessments, all in one place. SecLists helps make security testing more efficient and productive by conveniently providing all the lists a security tester might need. List types include usernames, passwords, URLs, fuzzing payloads, sensitive data patterns, web shells, and more. The tester can simply pull this repository onto a new test machine and he will have access to every type of list he needs.