


Clustering is a kind of unsupervised learning. Putting similar objects into the same cluster is a bit like fully automatic classification. The more similar the objects in the cluster are, the greater the difference between objects between clusters, and the better the clustering effect will be. good. This article mainly introduces the implementation of kMeans algorithm in python in detail, which has certain reference value. Interested friends can refer to it and hope it can help everyone.
1. k-means clustering algorithm
k-means clustering divides the data into k clusters, and each cluster passes its centroid, that is, the center of the cluster Describe the center of all points. First, k initial points are randomly determined as centroids, and then the data set is assigned to the closest cluster. The centroid of each cluster is then updated to be the average of all data sets. Then divide the data set a second time until the clustering results no longer change.
The pseudo code is
Randomly create k cluster centroids
When the cluster assignment of any point changes:
For each point in the data set Data points:
For each centroid:
Calculate the distance from the data set to the centroid
Allocate the data set to the cluster corresponding to the nearest centroid
For each cluster, calculate the mean of all points in the cluster And use the mean as the center of mass
python implementation
##
import numpy as np import matplotlib.pyplot as plt def loadDataSet(fileName): dataMat = [] with open(fileName) as f: for line in f.readlines(): line = line.strip().split('\t') dataMat.append(line) dataMat = np.array(dataMat).astype(np.float32) return dataMat def distEclud(vecA,vecB): return np.sqrt(np.sum(np.power((vecA-vecB),2))) def randCent(dataSet,k): m = np.shape(dataSet)[1] center = np.mat(np.ones((k,m))) for i in range(m): centmin = min(dataSet[:,i]) centmax = max(dataSet[:,i]) center[:,i] = centmin + (centmax - centmin) * np.random.rand(k,1) return center def kMeans(dataSet,k,distMeans = distEclud,createCent = randCent): m = np.shape(dataSet)[0] clusterAssment = np.mat(np.zeros((m,2))) centroids = createCent(dataSet,k) clusterChanged = True while clusterChanged: clusterChanged = False for i in range(m): minDist = np.inf minIndex = -1 for j in range(k): distJI = distMeans(dataSet[i,:],centroids[j,:]) if distJI < minDist: minDist = distJI minIndex = j if clusterAssment[i,0] != minIndex: clusterChanged = True clusterAssment[i,:] = minIndex,minDist**2 for cent in range(k): ptsInClust = dataSet[np.nonzero(clusterAssment[:,0].A == cent)[0]] centroids[cent,:] = np.mean(ptsInClust,axis = 0) return centroids,clusterAssment data = loadDataSet('testSet.txt') muCentroids, clusterAssing = kMeans(data,4) fig = plt.figure(0) ax = fig.add_subplot(111) ax.scatter(data[:,0],data[:,1],c = clusterAssing[:,0].A) plt.show() print(clusterAssing)
2. Bisection k-means algorithm
First treat all points as a cluster, then divide the cluster into two, and then select the cluster among all clusters that can minimize the SSE value until the specified number of clusters is met.
Consider all points as a clusterCalculate SSE
while When the number of clusters is less than k:
for each cluster :
being being being done to be –
” having Perform division operation
Python implementation
import numpy as np import matplotlib.pyplot as plt def loadDataSet(fileName): dataMat = [] with open(fileName) as f: for line in f.readlines(): line = line.strip().split('\t') dataMat.append(line) dataMat = np.array(dataMat).astype(np.float32) return dataMat def distEclud(vecA,vecB): return np.sqrt(np.sum(np.power((vecA-vecB),2))) def randCent(dataSet,k): m = np.shape(dataSet)[1] center = np.mat(np.ones((k,m))) for i in range(m): centmin = min(dataSet[:,i]) centmax = max(dataSet[:,i]) center[:,i] = centmin + (centmax - centmin) * np.random.rand(k,1) return center def kMeans(dataSet,k,distMeans = distEclud,createCent = randCent): m = np.shape(dataSet)[0] clusterAssment = np.mat(np.zeros((m,2))) centroids = createCent(dataSet,k) clusterChanged = True while clusterChanged: clusterChanged = False for i in range(m): minDist = np.inf minIndex = -1 for j in range(k): distJI = distMeans(dataSet[i,:],centroids[j,:]) if distJI < minDist: minDist = distJI minIndex = j if clusterAssment[i,0] != minIndex: clusterChanged = True clusterAssment[i,:] = minIndex,minDist**2 for cent in range(k): ptsInClust = dataSet[np.nonzero(clusterAssment[:,0].A == cent)[0]] centroids[cent,:] = np.mean(ptsInClust,axis = 0) return centroids,clusterAssment def biKmeans(dataSet,k,distMeans = distEclud): m = np.shape(dataSet)[0] clusterAssment = np.mat(np.zeros((m,2))) centroid0 = np.mean(dataSet,axis=0).tolist() centList = [centroid0] for j in range(m): clusterAssment[j,1] = distMeans(dataSet[j,:],np.mat(centroid0))**2 while (len(centList)<k): lowestSSE = np.inf for i in range(len(centList)): ptsInCurrCluster = dataSet[np.nonzero(clusterAssment[:,0].A == i)[0],:] centroidMat,splitClustAss = kMeans(ptsInCurrCluster,2,distMeans) sseSplit = np.sum(splitClustAss[:,1]) sseNotSplit = np.sum(clusterAssment[np.nonzero(clusterAssment[:,0].A != i)[0],1]) if (sseSplit + sseNotSplit) < lowestSSE: bestCentToSplit = i bestNewCents = centroidMat.copy() bestClustAss = splitClustAss.copy() lowestSSE = sseSplit + sseNotSplit print('the best cent to split is ',bestCentToSplit) # print('the len of the bestClust') bestClustAss[np.nonzero(bestClustAss[:,0].A == 1)[0],0] = len(centList) bestClustAss[np.nonzero(bestClustAss[:,0].A == 0)[0],0] = bestCentToSplit clusterAssment[np.nonzero(clusterAssment[:,0].A == bestCentToSplit)[0],:] = bestClustAss.copy() centList[bestCentToSplit] = bestNewCents[0,:].tolist()[0] centList.append(bestNewCents[1,:].tolist()[0]) return np.mat(centList),clusterAssment data = loadDataSet('testSet2.txt') muCentroids, clusterAssing = biKmeans(data,3) fig = plt.figure(0) ax = fig.add_subplot(111) ax.scatter(data[:,0],data[:,1],c = clusterAssing[:,0].A,cmap=plt.cm.Paired) ax.scatter(muCentroids[:,0],muCentroids[:,1]) plt.show() print(clusterAssing) print(muCentroids)
Code and data set download: K-means
Let Mahout KMeans cluster analysis run on Hadoop
##cvKMeans2 mean cluster analysis + code analysis + Grayscale color image clustering
Detailed example explanation of simple web page image grabbing using Python
The above is the detailed content of Detailed explanation of python implementation of kMeans algorithm. For more information, please follow other related articles on the PHP Chinese website!
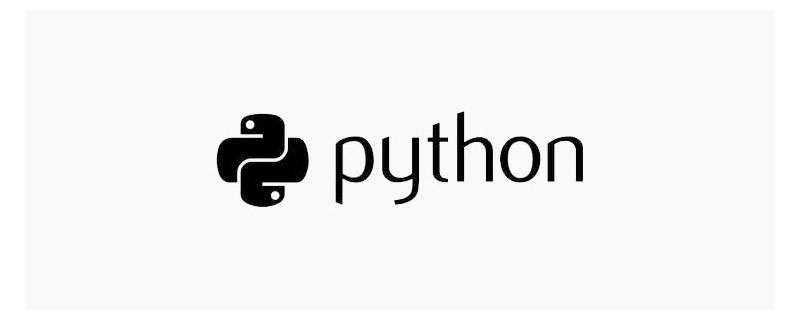
本篇文章给大家带来了关于Python的相关知识,其中主要介绍了关于Seaborn的相关问题,包括了数据可视化处理的散点图、折线图、条形图等等内容,下面一起来看一下,希望对大家有帮助。
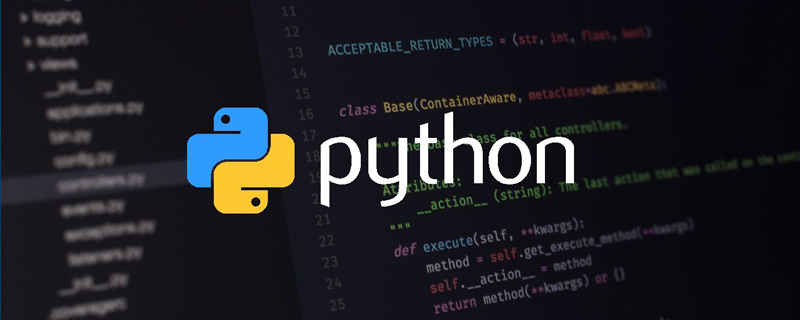
本篇文章给大家带来了关于Python的相关知识,其中主要介绍了关于进程池与进程锁的相关问题,包括进程池的创建模块,进程池函数等等内容,下面一起来看一下,希望对大家有帮助。
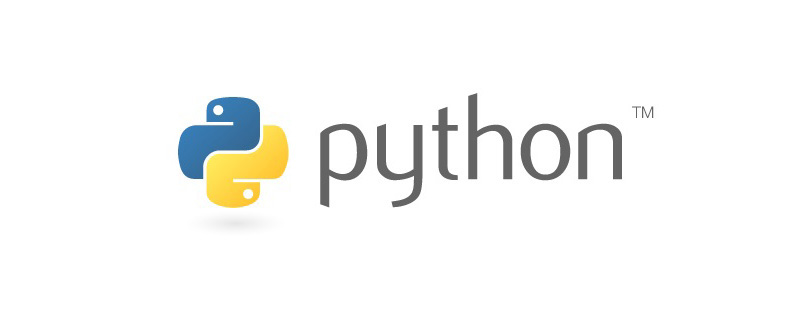
本篇文章给大家带来了关于Python的相关知识,其中主要介绍了关于简历筛选的相关问题,包括了定义 ReadDoc 类用以读取 word 文件以及定义 search_word 函数用以筛选的相关内容,下面一起来看一下,希望对大家有帮助。
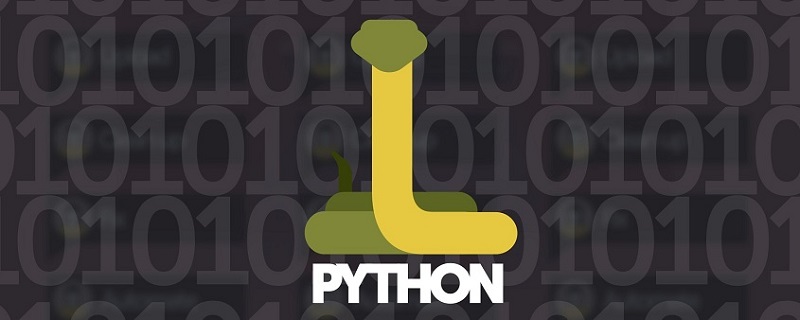
本篇文章给大家带来了关于Python的相关知识,其中主要介绍了关于数据类型之字符串、数字的相关问题,下面一起来看一下,希望对大家有帮助。
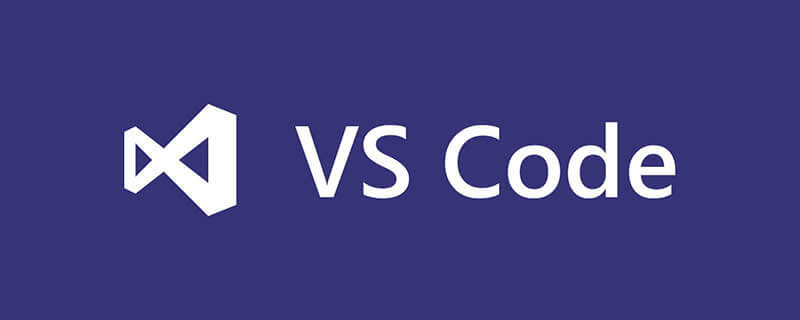
VS Code的确是一款非常热门、有强大用户基础的一款开发工具。本文给大家介绍一下10款高效、好用的插件,能够让原本单薄的VS Code如虎添翼,开发效率顿时提升到一个新的阶段。
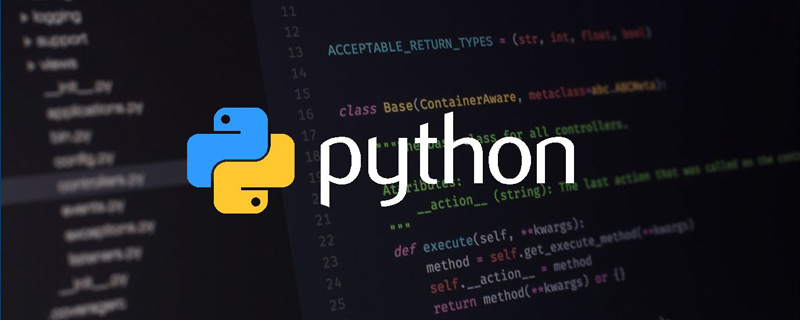
本篇文章给大家带来了关于Python的相关知识,其中主要介绍了关于numpy模块的相关问题,Numpy是Numerical Python extensions的缩写,字面意思是Python数值计算扩展,下面一起来看一下,希望对大家有帮助。
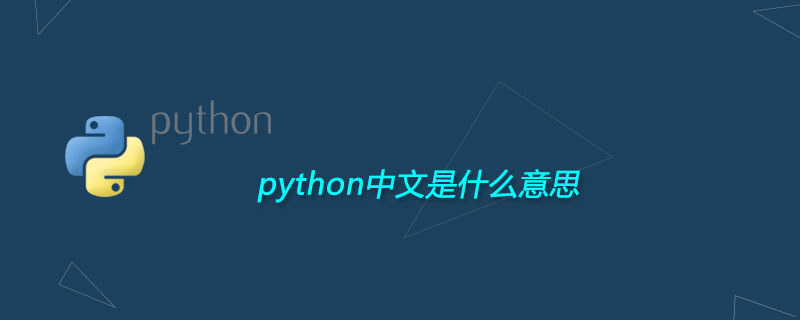
pythn的中文意思是巨蟒、蟒蛇。1989年圣诞节期间,Guido van Rossum在家闲的没事干,为了跟朋友庆祝圣诞节,决定发明一种全新的脚本语言。他很喜欢一个肥皂剧叫Monty Python,所以便把这门语言叫做python。


Hot AI Tools

Undresser.AI Undress
AI-powered app for creating realistic nude photos

AI Clothes Remover
Online AI tool for removing clothes from photos.

Undress AI Tool
Undress images for free

Clothoff.io
AI clothes remover

AI Hentai Generator
Generate AI Hentai for free.

Hot Article

Hot Tools

mPDF
mPDF is a PHP library that can generate PDF files from UTF-8 encoded HTML. The original author, Ian Back, wrote mPDF to output PDF files "on the fly" from his website and handle different languages. It is slower than original scripts like HTML2FPDF and produces larger files when using Unicode fonts, but supports CSS styles etc. and has a lot of enhancements. Supports almost all languages, including RTL (Arabic and Hebrew) and CJK (Chinese, Japanese and Korean). Supports nested block-level elements (such as P, DIV),

Notepad++7.3.1
Easy-to-use and free code editor

SAP NetWeaver Server Adapter for Eclipse
Integrate Eclipse with SAP NetWeaver application server.
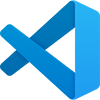
VSCode Windows 64-bit Download
A free and powerful IDE editor launched by Microsoft

DVWA
Damn Vulnerable Web App (DVWA) is a PHP/MySQL web application that is very vulnerable. Its main goals are to be an aid for security professionals to test their skills and tools in a legal environment, to help web developers better understand the process of securing web applications, and to help teachers/students teach/learn in a classroom environment Web application security. The goal of DVWA is to practice some of the most common web vulnerabilities through a simple and straightforward interface, with varying degrees of difficulty. Please note that this software
