算法优缺点
优点:在数据较少的情况下依然有效,可以处理多类别问题
缺点:对输入数据的准备方式敏感
适用数据类型:标称型数据
算法思想:
朴素贝叶斯
比如我们想判断一个邮件是不是垃圾邮件,那么我们知道的是这个邮件中的词的分布,那么我们还要知道:垃圾邮件中某些词的出现是多少,就可以利用贝叶斯定理得到。
朴素贝叶斯分类器中的一个假设是:每个特征同等重要
贝叶斯分类是一类分类算法的总称,这类算法均以贝叶斯定理为基础,故统称为贝叶斯分类。
函数
loadDataSet()
创建数据集,这里的数据集是已经拆分好的单词组成的句子,表示的是某论坛的用户评论,标签1表示这个是骂人的
createVocabList(dataSet)
找出这些句子中总共有多少单词,以确定我们词向量的大小
setOfWords2Vec(vocabList, inputSet)
将句子根据其中的单词转成向量,这里用的是伯努利模型,即只考虑这个单词是否存在
bagOfWords2VecMN(vocabList, inputSet)
这个是将句子转成向量的另一种模型,多项式模型,考虑某个词的出现次数
trainNB0(trainMatrix,trainCatergory)
计算P(i)和P(w[i]|C[1])和P(w[i]|C[0]),这里有两个技巧,一个是开始的分子分母没有全部初始化为0是为了防止其中一个的概率为0导致整体为0,另一个是后面乘用对数防止因为精度问题结果为0
classifyNB(vec2Classify, p0Vec, p1Vec, pClass1)
根据贝叶斯公式计算这个向量属于两个集合中哪个的概率高
#coding=utf-8 from numpy import * def loadDataSet(): postingList=[['my', 'dog', 'has', 'flea', 'problems', 'help', 'please'], ['maybe', 'not', 'take', 'him', 'to', 'dog', 'park', 'stupid'], ['my', 'dalmation', 'is', 'so', 'cute', 'I', 'love', 'him'], ['stop', 'posting', 'stupid', 'worthless', 'garbage'], ['mr', 'licks', 'ate', 'my', 'steak', 'how', 'to', 'stop', 'him'], ['quit', 'buying', 'worthless', 'dog', 'food', 'stupid']] classVec = [0,1,0,1,0,1] #1 is abusive, 0 not return postingList,classVec #创建一个带有所有单词的列表 def createVocabList(dataSet): vocabSet = set([]) for document in dataSet: vocabSet = vocabSet | set(document) return list(vocabSet) def setOfWords2Vec(vocabList, inputSet): retVocabList = [0] * len(vocabList) for word in inputSet: if word in vocabList: retVocabList[vocabList.index(word)] = 1 else: print 'word ',word ,'not in dict' return retVocabList #另一种模型 def bagOfWords2VecMN(vocabList, inputSet): returnVec = [0]*len(vocabList) for word in inputSet: if word in vocabList: returnVec[vocabList.index(word)] += 1 return returnVec def trainNB0(trainMatrix,trainCatergory): numTrainDoc = len(trainMatrix) numWords = len(trainMatrix[0]) pAbusive = sum(trainCatergory)/float(numTrainDoc) #防止多个概率的成绩当中的一个为0 p0Num = ones(numWords) p1Num = ones(numWords) p0Denom = 2.0 p1Denom = 2.0 for i in range(numTrainDoc): if trainCatergory[i] == 1: p1Num +=trainMatrix[i] p1Denom += sum(trainMatrix[i]) else: p0Num +=trainMatrix[i] p0Denom += sum(trainMatrix[i]) p1Vect = log(p1Num/p1Denom)#处于精度的考虑,否则很可能到限归零 p0Vect = log(p0Num/p0Denom) return p0Vect,p1Vect,pAbusive def classifyNB(vec2Classify, p0Vec, p1Vec, pClass1): p1 = sum(vec2Classify * p1Vec) + log(pClass1) #element-wise mult p0 = sum(vec2Classify * p0Vec) + log(1.0 - pClass1) if p1 > p0: return 1 else: return 0 def testingNB(): listOPosts,listClasses = loadDataSet() myVocabList = createVocabList(listOPosts) trainMat=[] for postinDoc in listOPosts: trainMat.append(setOfWords2Vec(myVocabList, postinDoc)) p0V,p1V,pAb = trainNB0(array(trainMat),array(listClasses)) testEntry = ['love', 'my', 'dalmation'] thisDoc = array(setOfWords2Vec(myVocabList, testEntry)) print testEntry,'classified as: ',classifyNB(thisDoc,p0V,p1V,pAb) testEntry = ['stupid', 'garbage'] thisDoc = array(setOfWords2Vec(myVocabList, testEntry)) print testEntry,'classified as: ',classifyNB(thisDoc,p0V,p1V,pAb) def main(): testingNB() if __name__ == '__main__': main()
The above is the detailed content of pythonde Naive Bayes algorithm. For more information, please follow other related articles on the PHP Chinese website!
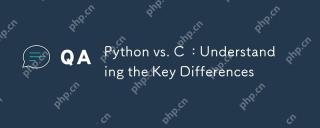
Python and C each have their own advantages, and the choice should be based on project requirements. 1) Python is suitable for rapid development and data processing due to its concise syntax and dynamic typing. 2)C is suitable for high performance and system programming due to its static typing and manual memory management.
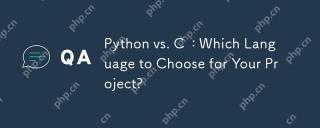
Choosing Python or C depends on project requirements: 1) If you need rapid development, data processing and prototype design, choose Python; 2) If you need high performance, low latency and close hardware control, choose C.
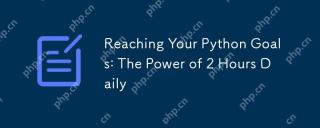
By investing 2 hours of Python learning every day, you can effectively improve your programming skills. 1. Learn new knowledge: read documents or watch tutorials. 2. Practice: Write code and complete exercises. 3. Review: Consolidate the content you have learned. 4. Project practice: Apply what you have learned in actual projects. Such a structured learning plan can help you systematically master Python and achieve career goals.
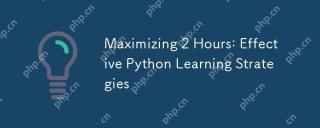
Methods to learn Python efficiently within two hours include: 1. Review the basic knowledge and ensure that you are familiar with Python installation and basic syntax; 2. Understand the core concepts of Python, such as variables, lists, functions, etc.; 3. Master basic and advanced usage by using examples; 4. Learn common errors and debugging techniques; 5. Apply performance optimization and best practices, such as using list comprehensions and following the PEP8 style guide.
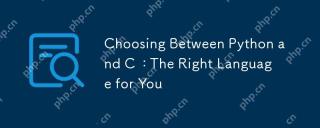
Python is suitable for beginners and data science, and C is suitable for system programming and game development. 1. Python is simple and easy to use, suitable for data science and web development. 2.C provides high performance and control, suitable for game development and system programming. The choice should be based on project needs and personal interests.
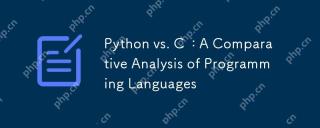
Python is more suitable for data science and rapid development, while C is more suitable for high performance and system programming. 1. Python syntax is concise and easy to learn, suitable for data processing and scientific computing. 2.C has complex syntax but excellent performance and is often used in game development and system programming.
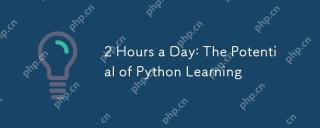
It is feasible to invest two hours a day to learn Python. 1. Learn new knowledge: Learn new concepts in one hour, such as lists and dictionaries. 2. Practice and exercises: Use one hour to perform programming exercises, such as writing small programs. Through reasonable planning and perseverance, you can master the core concepts of Python in a short time.
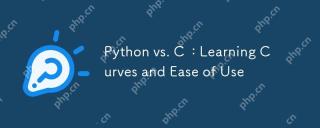
Python is easier to learn and use, while C is more powerful but complex. 1. Python syntax is concise and suitable for beginners. Dynamic typing and automatic memory management make it easy to use, but may cause runtime errors. 2.C provides low-level control and advanced features, suitable for high-performance applications, but has a high learning threshold and requires manual memory and type safety management.


Hot AI Tools

Undresser.AI Undress
AI-powered app for creating realistic nude photos

AI Clothes Remover
Online AI tool for removing clothes from photos.

Undress AI Tool
Undress images for free

Clothoff.io
AI clothes remover

Video Face Swap
Swap faces in any video effortlessly with our completely free AI face swap tool!

Hot Article

Hot Tools

DVWA
Damn Vulnerable Web App (DVWA) is a PHP/MySQL web application that is very vulnerable. Its main goals are to be an aid for security professionals to test their skills and tools in a legal environment, to help web developers better understand the process of securing web applications, and to help teachers/students teach/learn in a classroom environment Web application security. The goal of DVWA is to practice some of the most common web vulnerabilities through a simple and straightforward interface, with varying degrees of difficulty. Please note that this software
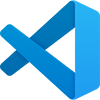
VSCode Windows 64-bit Download
A free and powerful IDE editor launched by Microsoft
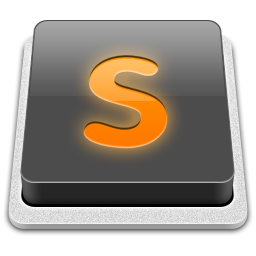
SublimeText3 Mac version
God-level code editing software (SublimeText3)

SAP NetWeaver Server Adapter for Eclipse
Integrate Eclipse with SAP NetWeaver application server.
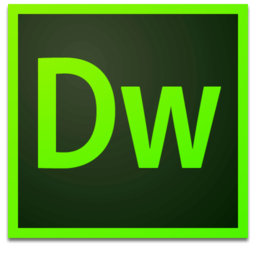
Dreamweaver Mac version
Visual web development tools