English name: Decision Tree
The decision tree is a typical classification method. The data is first processed, the inductive algorithm is used to generate readable rules and decision trees, and then the decision is used to analyze the new data. . Essentially a decision tree is the process of classifying data through a series of rules.
Decision tree is a supervised learning method, mainly used for classification and regression. The goal of the algorithm is to create a model that predicts the target variable by inferring data features and learning decision rules.
The decision tree is similar to the if-else structure. The result is that you have to generate a tree that can continuously judge and select from the root of the tree to the leaf nodes. But the if-else judgment conditions here are not manually set, but automatically generated by the computer based on the algorithm we provide.
Decision tree elements
Decision points
are several possibilities The choice of plan is the best plan chosen in the end. If the decision is a multi-level decision, there can be multiple decision points in the middle of the decision tree, and the decision point at the root of the decision tree is the final decision plan.
State node
represents the economic effect (expected value) of the alternative. By comparing the economic effect of each state node, according to a certain Decision criteria can be used to select the best solution. The branches derived from the state nodes are called probability branches. The number of probability branches represents the number of possible natural states that may occur. The probability of the occurrence of the state must be noted on each branch.
Result Node
Mark the profit and loss value of each plan under various natural states on the right end of the result node
Advantages and disadvantages of decision tree group
Advantages of decision tree
Easy to understand, clear principles, decision tree can be visualized
The reasoning process is easy to understand, and the decision-making reasoning process can be expressed in the if-else form
The reasoning process completely depends on the value characteristics of the attribute variables
Can automatically ignore attribute variables that do not contribute to the target variable, and also provide a reference for judging the importance of attribute variables and reducing the number of variables
Disadvantages of decision trees
It is possible to establish overly complex rules, that is, overfitting.
Decision trees are sometimes unstable, because small changes in the data may generate completely different decision trees.
Learning the optimal decision tree is an NP-complete problem. Therefore, actual decision tree learning algorithms are based on heuristic algorithms, such as greedy algorithms that achieve local optimal values at each node. Such an algorithm cannot guarantee to return a globally optimal decision tree. This problem can be alleviated by training multiple decision trees by randomly selecting features and samples.
Some problems are very difficult to learn because decision trees are difficult to express. Such as: XOR problem, parity check or multiplexer problem
If some factors dominate, the decision tree is biased. Therefore, it is recommended to balance the influencing factors of the data before fitting the decision tree.
Common algorithms for decision trees
There are many algorithms for decision trees, including CART, ID3, C4.5, C5.0, etc. Among them, ID3, C4.5, C5.0 is based on information entropy, while CART uses an index similar to entropy as a classification decision. After the decision tree is formed, it must be pruned.
Entropy: The degree of disorder of the system
ID3 algorithm
The ID3 algorithm is a classification decision tree algorithm. He finally classified the data into the form of a decision tree through a series of rules, and the basis of classification was entropy.
The ID3 algorithm is a classic decision tree learning algorithm proposed by Quinlan. The basic idea of the ID3 algorithm is to use information entropy as a measure for attribute selection of decision tree nodes. Each time, the attribute with the most information is prioritized, that is, the attribute that can minimize the entropy value to construct an entropy value. The fastest descending decision tree has an entropy value of 0 to the leaf node. At this time, the instances in the instance set corresponding to each leaf node belong to the same class.
Use the ID3 algorithm to realize early warning analysis of customer churn and find out the characteristics of customer churn to help telecommunications companies improve customer relationships in a targeted manner and avoid customer churn
Use the decision tree method to conduct Data mining generally has the following steps: data preprocessing, decision tree mining operations, pattern evaluation and application.
C4.5 algorithm
C4.5 is a further extension of ID3, which removes the limitations of features by discretizing continuous attributes. C4.5 converts the training tree into a series of if-then grammar rules. The accuracy of these rules can be determined to determine which ones should be adopted. If accuracy can be improved by removing a rule, pruning should be implemented.
The core algorithm of C4.5 and ID3 is the same, but the method used is different. C4.5 uses the information gain rate as the basis for division, which overcomes the problem of using information in the ID3 algorithm. Gain partitioning causes attribute selection to favor attributes with more values.
C5.0 algorithm
C5.0 uses smaller memory than C4.5, establishes smaller decision rules, and is more accurate.
CART algorithm
Classification and Regression Tree (CART - Classification And Regression Tree)) is a very interesting and very effective non-parametric classification and regression method. It achieves prediction purposes by constructing a binary tree. The classification and regression tree CART model was first proposed by Breiman et al. and has been commonly used in the field of statistics and data mining technology. It constructs prediction criteria in a completely different way from traditional statistics. It is given in the form of a binary tree, which is easy to understand, use and interpret. The prediction tree constructed by the CART model is in many cases more accurate than the algebraic prediction criteria constructed by commonly used statistical methods, and the more complex the data and the more variables there are, the more significant the superiority of the algorithm becomes. The key to the model is the construction of prediction criteria, accurately. Definition: Classification and regression first use known multivariate data to construct prediction criteria, and then predict one variable based on the values of other variables. In classification, people often first make various measurements on an object, and then use certain classification criteria to determine which category the object belongs to. For example, given the identification characteristics of a certain fossil, predict which family, which genus, or even which species the fossil belongs to. Another example is to predict whether there are minerals in the area based on the geological and geophysical information of a certain area. Regression is different from classification in that it is used to predict a certain value of an object rather than classifying the object. For example, given the characteristics of mineral resources in a certain area, predict the amount of resources in the area.
CART is very similar to C4.5, but it supports numerical target variables (regression) and does not generate decision rules. CART uses features and thresholds to obtain maximum information gain at each node to build a decision tree.
scikit-learn uses the CART algorithm
Sample code:
#! /usr/bin/env python#-*- coding:utf-8 -*-from sklearn import treeimport numpy as np# scikit-learn使用的决策树算法是CARTX = [[0,0],[1,1]] Y = ["A","B"] clf = tree.DecisionTreeClassifier() clf = clf.fit(X,Y) data1 = np.array([2.,2.]).reshape(1,-1)print clf.predict(data1) # 预测类别 print clf.predict_proba(data1) # 预测属于各个类的概率
Okay, that’s it, I hope it’s helpful You have help.
The github address of this article:
20170619_Decision Tree Algorithm.md
Welcome to add
The above is the detailed content of What is the decision tree algorithm?. For more information, please follow other related articles on the PHP Chinese website!
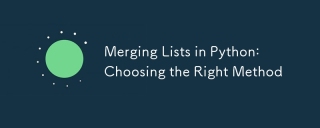
TomergelistsinPython,youcanusethe operator,extendmethod,listcomprehension,oritertools.chain,eachwithspecificadvantages:1)The operatorissimplebutlessefficientforlargelists;2)extendismemory-efficientbutmodifiestheoriginallist;3)listcomprehensionoffersf
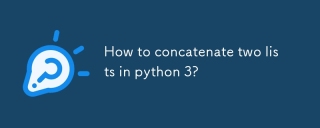
In Python 3, two lists can be connected through a variety of methods: 1) Use operator, which is suitable for small lists, but is inefficient for large lists; 2) Use extend method, which is suitable for large lists, with high memory efficiency, but will modify the original list; 3) Use * operator, which is suitable for merging multiple lists, without modifying the original list; 4) Use itertools.chain, which is suitable for large data sets, with high memory efficiency.
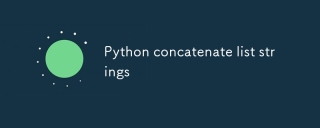
Using the join() method is the most efficient way to connect strings from lists in Python. 1) Use the join() method to be efficient and easy to read. 2) The cycle uses operators inefficiently for large lists. 3) The combination of list comprehension and join() is suitable for scenarios that require conversion. 4) The reduce() method is suitable for other types of reductions, but is inefficient for string concatenation. The complete sentence ends.
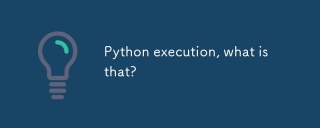
PythonexecutionistheprocessoftransformingPythoncodeintoexecutableinstructions.1)Theinterpreterreadsthecode,convertingitintobytecode,whichthePythonVirtualMachine(PVM)executes.2)TheGlobalInterpreterLock(GIL)managesthreadexecution,potentiallylimitingmul
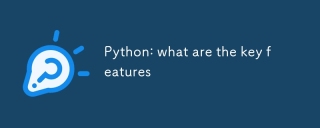
Key features of Python include: 1. The syntax is concise and easy to understand, suitable for beginners; 2. Dynamic type system, improving development speed; 3. Rich standard library, supporting multiple tasks; 4. Strong community and ecosystem, providing extensive support; 5. Interpretation, suitable for scripting and rapid prototyping; 6. Multi-paradigm support, suitable for various programming styles.
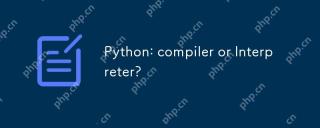
Python is an interpreted language, but it also includes the compilation process. 1) Python code is first compiled into bytecode. 2) Bytecode is interpreted and executed by Python virtual machine. 3) This hybrid mechanism makes Python both flexible and efficient, but not as fast as a fully compiled language.
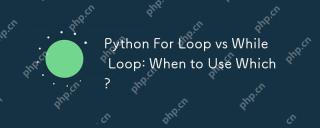
Useaforloopwheniteratingoverasequenceorforaspecificnumberoftimes;useawhileloopwhencontinuinguntilaconditionismet.Forloopsareidealforknownsequences,whilewhileloopssuitsituationswithundeterminediterations.
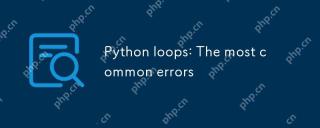
Pythonloopscanleadtoerrorslikeinfiniteloops,modifyinglistsduringiteration,off-by-oneerrors,zero-indexingissues,andnestedloopinefficiencies.Toavoidthese:1)Use'i


Hot AI Tools

Undresser.AI Undress
AI-powered app for creating realistic nude photos

AI Clothes Remover
Online AI tool for removing clothes from photos.

Undress AI Tool
Undress images for free

Clothoff.io
AI clothes remover

Video Face Swap
Swap faces in any video effortlessly with our completely free AI face swap tool!

Hot Article

Hot Tools

SublimeText3 Linux new version
SublimeText3 Linux latest version

SecLists
SecLists is the ultimate security tester's companion. It is a collection of various types of lists that are frequently used during security assessments, all in one place. SecLists helps make security testing more efficient and productive by conveniently providing all the lists a security tester might need. List types include usernames, passwords, URLs, fuzzing payloads, sensitive data patterns, web shells, and more. The tester can simply pull this repository onto a new test machine and he will have access to every type of list he needs.
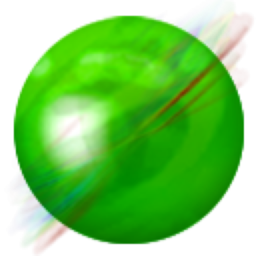
ZendStudio 13.5.1 Mac
Powerful PHP integrated development environment

DVWA
Damn Vulnerable Web App (DVWA) is a PHP/MySQL web application that is very vulnerable. Its main goals are to be an aid for security professionals to test their skills and tools in a legal environment, to help web developers better understand the process of securing web applications, and to help teachers/students teach/learn in a classroom environment Web application security. The goal of DVWA is to practice some of the most common web vulnerabilities through a simple and straightforward interface, with varying degrees of difficulty. Please note that this software

Notepad++7.3.1
Easy-to-use and free code editor
