Warning: This article is only for study and research reference purposes, please do not use it for illegal purposes. In the previous article "Mobike Unofficial Big Data Analysis", I mentioned my data analysis of Mobike during the Spring Festival. In the following series of articles, I will further elaborate on how my crawler can efficiently crawl to of these data. Why crawl Mobike’s data? Mobike was the first shared bicycle to enter Chengdu. Every day when I get off the subway station, I can see many bicycles in the APP, but when I get there, I find that the bicycles are not there. Some cars are hidden somewhere; some cars may be behind high-rise buildings and cannot be found due to GPS errors; some cars are placed in residential areas, separated by a wall so that cyclists cannot get to them. So is there a way to obtain the data of these bicycles to analyze whether these bicycles have become zombie bicycles? Did someone deliberately put it in the community so that no one can access it? With these questions in mind, I started researching how to obtain this data. Where to get the data? If you can see the data, there is always a way to get it automatically. It’s just that the method of obtaining data determines the efficiency of obtaining data. For the task of data analysis of Mobike, this crawler
1. Mobike crawler analysis-find API
##Introduction: Warning: This article is only for learning and research reference purposes, please do not use it for illegal purposes Purpose. In the previous article "Mobike Unofficial Big Data Analysis", I mentioned my data analysis of Mobike during the Spring Festival. In the following series of articles, I will further elaborate on how my crawler can efficiently crawl to of these data. Why climb the data of Mobike? Mobike is the first shared bicycle to enter Chengdu. Every day when I get off the subway station, I can see many bicycles in the APP, but when I walk there, I find that the bicycles are not there. Some cars are hidden somewhere; some cars may be in high...
2. Using Python for big data analysis
#Introduction: It is no exaggeration to say that big data has become an indispensable part of any business communication. Desktop and mobile search provide data to marketers and companies around the world at an unprecedented scale, and with the advent of the Internet of Things, the amount of data available for consumption will grow exponentially. This consumption data is a gold mine for companies that want to better target customers, understand how people use their products or services, and collect information to improve profits.
3. Preface to Big Data Analysis Beyond Hadoop
#4 implemented by Google.
Impala: a new generation of open source big data analysis engine
5.
Using Hadoop MapReduce for big data analysis
Introduction: Source: http://www.ibm.com/developerworks/cn/java/j-javadev2-15/index.html When Google released its image search feature in 2001, there were only 250 million indexed images. In less than 10 years, This massive search feature can already retrieve more than 10 billion images, with 35 hours of content uploaded to YouTube every minute. Allegedly, T6. Big Data Analysis: Using Hunk with Hadoop or ElasticMapReduce Introduction: Author Jonathan Allen, translator Zhang Xiaopeng Hunk is a Splunk company A relatively new product for detecting and visualizing Hadoop and other NoSQL data stores, its new version will support Amazon's Elastic MapReduce. Using Hunk with Hadoop Hadoop consists of two units. The first is a storage unit called HDFS. HDFS can 7. Microsoft releases a preview version of SQL Server 2014 to show memory Database Technology #Introduction: At this year’s TechEd conference, Microsoft announced the first technical preview version of SQL Server 2014, which will be officially available for download this month, and the product is officially The release time is initially scheduled for the end of this year. The biggest highlight of the new version is the in-memory OLTP (On-Line Transaction Processing, online transaction processing system) at the table granularity level and the ability to provide real-time big data analysis ##8. Similar How does the Java language handle "big data analysis"? Friends with experience, please share it Introduction: My understanding of "big data analysis" is to make some algorithm calls on existing data and return it to the matching group (such as Baidu Alliance , Taobao Alliance) So how is it handled specifically in programming projects? Is it just like my understanding? It feels like it’s not that simple... All the big data analysis I see is recruiting... [Related Q&A recommendations]: php - How does the Java language handle "big data analysis"? Friends with experience, please share it #html - What is the functional principle of getting the text from the web page in the Evernote clipping function?
The above is the detailed content of Recommended courses on big data analysis. For more information, please follow other related articles on the PHP Chinese website!
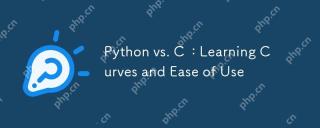
Python is easier to learn and use, while C is more powerful but complex. 1. Python syntax is concise and suitable for beginners. Dynamic typing and automatic memory management make it easy to use, but may cause runtime errors. 2.C provides low-level control and advanced features, suitable for high-performance applications, but has a high learning threshold and requires manual memory and type safety management.
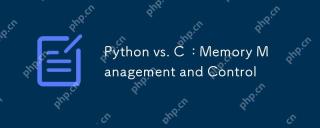
Python and C have significant differences in memory management and control. 1. Python uses automatic memory management, based on reference counting and garbage collection, simplifying the work of programmers. 2.C requires manual management of memory, providing more control but increasing complexity and error risk. Which language to choose should be based on project requirements and team technology stack.
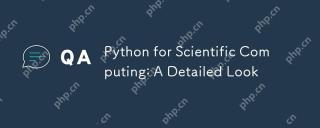
Python's applications in scientific computing include data analysis, machine learning, numerical simulation and visualization. 1.Numpy provides efficient multi-dimensional arrays and mathematical functions. 2. SciPy extends Numpy functionality and provides optimization and linear algebra tools. 3. Pandas is used for data processing and analysis. 4.Matplotlib is used to generate various graphs and visual results.
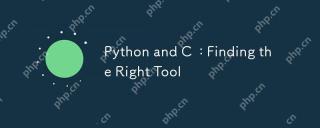
Whether to choose Python or C depends on project requirements: 1) Python is suitable for rapid development, data science, and scripting because of its concise syntax and rich libraries; 2) C is suitable for scenarios that require high performance and underlying control, such as system programming and game development, because of its compilation and manual memory management.
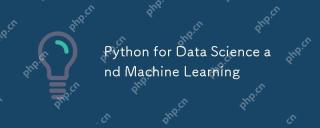
Python is widely used in data science and machine learning, mainly relying on its simplicity and a powerful library ecosystem. 1) Pandas is used for data processing and analysis, 2) Numpy provides efficient numerical calculations, and 3) Scikit-learn is used for machine learning model construction and optimization, these libraries make Python an ideal tool for data science and machine learning.
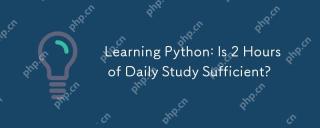
Is it enough to learn Python for two hours a day? It depends on your goals and learning methods. 1) Develop a clear learning plan, 2) Select appropriate learning resources and methods, 3) Practice and review and consolidate hands-on practice and review and consolidate, and you can gradually master the basic knowledge and advanced functions of Python during this period.
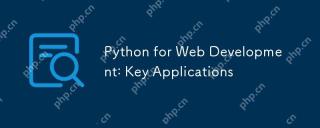
Key applications of Python in web development include the use of Django and Flask frameworks, API development, data analysis and visualization, machine learning and AI, and performance optimization. 1. Django and Flask framework: Django is suitable for rapid development of complex applications, and Flask is suitable for small or highly customized projects. 2. API development: Use Flask or DjangoRESTFramework to build RESTfulAPI. 3. Data analysis and visualization: Use Python to process data and display it through the web interface. 4. Machine Learning and AI: Python is used to build intelligent web applications. 5. Performance optimization: optimized through asynchronous programming, caching and code
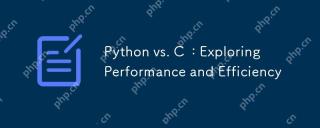
Python is better than C in development efficiency, but C is higher in execution performance. 1. Python's concise syntax and rich libraries improve development efficiency. 2.C's compilation-type characteristics and hardware control improve execution performance. When making a choice, you need to weigh the development speed and execution efficiency based on project needs.


Hot AI Tools

Undresser.AI Undress
AI-powered app for creating realistic nude photos

AI Clothes Remover
Online AI tool for removing clothes from photos.

Undress AI Tool
Undress images for free

Clothoff.io
AI clothes remover

Video Face Swap
Swap faces in any video effortlessly with our completely free AI face swap tool!

Hot Article

Hot Tools

MantisBT
Mantis is an easy-to-deploy web-based defect tracking tool designed to aid in product defect tracking. It requires PHP, MySQL and a web server. Check out our demo and hosting services.

SAP NetWeaver Server Adapter for Eclipse
Integrate Eclipse with SAP NetWeaver application server.

MinGW - Minimalist GNU for Windows
This project is in the process of being migrated to osdn.net/projects/mingw, you can continue to follow us there. MinGW: A native Windows port of the GNU Compiler Collection (GCC), freely distributable import libraries and header files for building native Windows applications; includes extensions to the MSVC runtime to support C99 functionality. All MinGW software can run on 64-bit Windows platforms.

PhpStorm Mac version
The latest (2018.2.1) professional PHP integrated development tool

Atom editor mac version download
The most popular open source editor