


Web抓取
Web站点使用HTML描述,这意味着每个web页面是一个结构化的文档。有时从中 获取数据同时保持它的结构是有用的。web站点不总是以容易处理的格式, 如 csv 或者 json 提供它们的数据。
这正是web抓取出场的时机。Web抓取是使用计算机程序将web页面数据进行收集 并整理成所需格式,同时保存其结构的实践。
lxml和Requests
lxml(http://lxml.de/)是一个优美的扩展库,用来快速解析XML以及HTML文档 即使所处理的标签非常混乱。我们也将使用 Requests (http://docs.python-requests.org/en/latest/#)模块取代内建的urllib2模块,因为其速度更快而且可读性更好。你可以通过使用 pip install lxml 与 pip install requests 命令来安装这两个模块。
让我们以下面的导入开始:
from lxml import html import requests
下一步我们将使用 requests.get 来从web页面中取得我们的数据, 通过使用 html 模块解析它,并将结果保存到 tree 中。
page = requests.get('http://econpy.pythonanywhere.com/ex/001.html') tree = html.fromstring(page.text)
tree 现在包含了整个HTML文件到一个优雅的树结构中,我们可以使用两种 方法访问:XPath以及CSS选择器。在这个例子中,我们将选择前者。
XPath是一种在结构化文档(如HTML或XML)中定位信息的方式。一个关于XPath的 不错的介绍参见 W3Schools 。
有很多工具可以获取元素的XPath,如Firefox的FireBug或者Chrome的Inspector。 如果你使用Chrome,你可以右键元素,选择 ‘Inspect element',高亮这段代码, 再次右击,并选择 ‘Copy XPath'。
在进行一次快速分析后,我们看到在页面中的数据保存在两个元素中,一个是title是 ‘buyer-name' 的p,另一个class是 ‘item-price' 的span:
<p title="buyer-name">Carson Busses</p> <span class="item-price">$29.95</span>
知道这个后,我们可以创建正确的XPath查询并且使用lxml的 xpath 函数, 像下面这样:
#这将创建buyers的列表: buyers = tree.xpath('//p[@title="buyer-name"]/text()') #这将创建prices的列表: prices = tree.xpath('//span[@class="item-price"]/text()')
让我们看看我们得到了什么:
print 'Buyers: ', buyers print 'Prices: ', prices Buyers: ['Carson Busses', 'Earl E. Byrd', 'Patty Cakes', 'Derri Anne Connecticut', 'Moe Dess', 'Leda Doggslife', 'Dan Druff', 'Al Fresco', 'Ido Hoe', 'Howie Kisses', 'Len Lease', 'Phil Meup', 'Ira Pent', 'Ben D. Rules', 'Ave Sectomy', 'Gary Shattire', 'Bobbi Soks', 'Sheila Takya', 'Rose Tattoo', 'Moe Tell'] Prices: ['$29.95', '$8.37', '$15.26', '$19.25', '$19.25', '$13.99', '$31.57', '$8.49', '$14.47', '$15.86', '$11.11', '$15.98', '$16.27', '$7.50', '$50.85', '$14.26', '$5.68', '$15.00', '$114.07', '$10.09']
恭喜!我们已经成功地通过lxml与Request,从一个web页面中抓取了所有我们想要的 数据。我们将它们以列表的形式存在内存中。现在我们可以对它做各种很酷的事情了: 我们可以使用Python分析它,或者我们可以将之保存为一个文件并向世界分享。
我们可以考虑一些更酷的想法:修改这个脚本来遍历该例数据集中剩余的页面,或者 使用多线程重写这个应用从而提升它的速度。
更多Python使用lxml模块和Requests模块抓取HTML页面相关文章请关注PHP中文网!
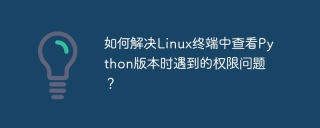
Solution to permission issues when viewing Python version in Linux terminal When you try to view Python version in Linux terminal, enter python...
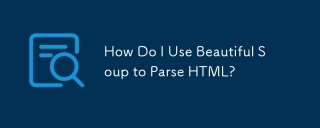
This article explains how to use Beautiful Soup, a Python library, to parse HTML. It details common methods like find(), find_all(), select(), and get_text() for data extraction, handling of diverse HTML structures and errors, and alternatives (Sel
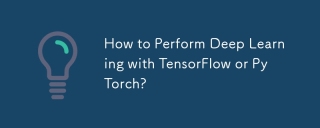
This article compares TensorFlow and PyTorch for deep learning. It details the steps involved: data preparation, model building, training, evaluation, and deployment. Key differences between the frameworks, particularly regarding computational grap
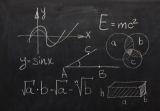
Python's statistics module provides powerful data statistical analysis capabilities to help us quickly understand the overall characteristics of data, such as biostatistics and business analysis. Instead of looking at data points one by one, just look at statistics such as mean or variance to discover trends and features in the original data that may be ignored, and compare large datasets more easily and effectively. This tutorial will explain how to calculate the mean and measure the degree of dispersion of the dataset. Unless otherwise stated, all functions in this module support the calculation of the mean() function instead of simply summing the average. Floating point numbers can also be used. import random import statistics from fracti
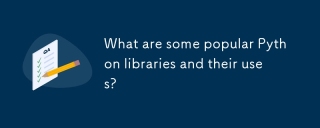
The article discusses popular Python libraries like NumPy, Pandas, Matplotlib, Scikit-learn, TensorFlow, Django, Flask, and Requests, detailing their uses in scientific computing, data analysis, visualization, machine learning, web development, and H
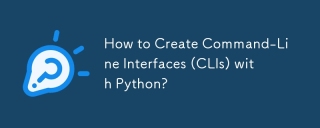
This article guides Python developers on building command-line interfaces (CLIs). It details using libraries like typer, click, and argparse, emphasizing input/output handling, and promoting user-friendly design patterns for improved CLI usability.
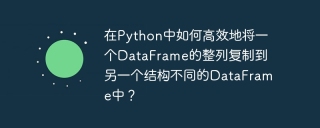
When using Python's pandas library, how to copy whole columns between two DataFrames with different structures is a common problem. Suppose we have two Dats...
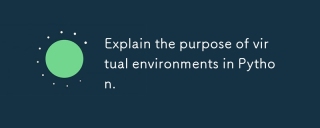
The article discusses the role of virtual environments in Python, focusing on managing project dependencies and avoiding conflicts. It details their creation, activation, and benefits in improving project management and reducing dependency issues.


Hot AI Tools

Undresser.AI Undress
AI-powered app for creating realistic nude photos

AI Clothes Remover
Online AI tool for removing clothes from photos.

Undress AI Tool
Undress images for free

Clothoff.io
AI clothes remover

AI Hentai Generator
Generate AI Hentai for free.

Hot Article

Hot Tools

Atom editor mac version download
The most popular open source editor
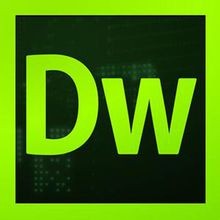
Dreamweaver CS6
Visual web development tools
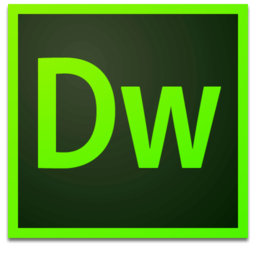
Dreamweaver Mac version
Visual web development tools

Notepad++7.3.1
Easy-to-use and free code editor

MinGW - Minimalist GNU for Windows
This project is in the process of being migrated to osdn.net/projects/mingw, you can continue to follow us there. MinGW: A native Windows port of the GNU Compiler Collection (GCC), freely distributable import libraries and header files for building native Windows applications; includes extensions to the MSVC runtime to support C99 functionality. All MinGW software can run on 64-bit Windows platforms.