


14 Powerful Techniques Defining the Evolution of Embedding - Analytics Vidhya
This article explores the evolution of text embeddings, from simple count-based methods to sophisticated context-aware models. It highlights the role of leaderboards like MTEB in evaluating embedding performance and the accessibility of cutting-edge models through platforms like Hugging Face.
Table of Contents:
- MTEB Leaderboard Rankings
- Count Vectorization
- One-Hot Encoding
- TF-IDF
- Okapi BM25
- Word2Vec
- GloVe
- FastText
- Doc2Vec
- InferSent
- Universal Sentence Encoder (USE)
- Node2Vec
- ELMo
- BERT and its Variants (SBERT, DistilBERT, RoBERTa)
- CLIP and BLIP
- Embedding Comparison
- Conclusion
MTEB Leaderboard Rankings:
Modern large language models (LLMs) produce embeddings as part of their architecture. These can be fine-tuned for various tasks, making them highly versatile. The Massive Text Embedding Benchmark (MTEB) Leaderboard ranks these models based on performance across tasks like classification and retrieval, aiding practitioners in model selection.
The article then delves into classic vectorization techniques, demonstrating their role as foundational elements for modern embeddings.
Count Vectorization: This simple method represents text as vectors based on word counts. While straightforward and interpretable, it suffers from high dimensionality and lacks semantic context.
Code Example: (Code snippet included showing sklearn's CountVectorizer)
Output: (Image of CountVectorizer output included)
One-Hot Encoding: This technique assigns unique binary vectors to each word. Simple but highly inefficient for large vocabularies and lacks semantic understanding.
Code Example: (Code snippet included showing sklearn's CountVectorizer with binary=True)
Output: (Image of One-Hot Encoding output included)
The article compares CountVectorizer and One-Hot Encoding, highlighting their respective strengths and weaknesses. (Image comparing the two included)
TF-IDF (Term Frequency-Inverse Document Frequency): TF-IDF weighs word importance by considering both frequency within a document and rarity across the corpus. It improves upon raw counts but still lacks contextual understanding.
Code Example: (Code snippet included showing sklearn's TfidfVectorizer)
Output: (Image of TF-IDF output included)
Okapi BM25: A probabilistic model primarily for document ranking, BM25 enhances TF-IDF by accounting for document length and term frequency saturation. It's not a true embedding method but is influential in information retrieval.
Code Example: (Code snippet included showing BM25 implementation)
Output: (Image of BM25 output included)
Word2Vec (CBOW and Skip-gram): Word2Vec uses neural networks to learn dense vector representations capturing semantic and syntactic relationships. CBOW predicts a word from its context, while skip-gram predicts context from a word.
Code Example: (Code snippet included showing gensim's Word2Vec)
Output: (Image of Word2Vec output and visualizations included)
GloVe (Global Vectors for Word Representation): GloVe combines global co-occurrence statistics with local context to generate embeddings. It's known for its consistent representation across contexts.
Code Example: (Code snippet included showing GloVe implementation)
Output: (Image of GloVe output and visualization included)
FastText: FastText extends Word2Vec by incorporating subword information, improving handling of rare words and morphologically rich languages.
Code Example: (Code snippet included showing FastText implementation)
Output: (Image of FastText output included)
Doc2Vec: Doc2Vec extends Word2Vec to generate fixed-length vectors for variable-length documents, enabling document-level analysis.
Code Example: (Code snippet included showing Doc2Vec implementation)
Output: (Image of Doc2Vec output included)
InferSent: InferSent uses supervised learning on natural language inference datasets to generate high-quality sentence embeddings.
Code Example: (Reference to Kaggle notebook)
Output: (Image of InferSent output included)
Universal Sentence Encoder (USE): USE provides general-purpose sentence embeddings suitable for various NLP tasks with minimal fine-tuning.
Code Example: (Code snippet included showing USE implementation)
Output: (Image of USE output and visualization included)
Node2Vec: Node2Vec is a graph embedding technique applicable to NLP tasks involving network data.
Code Example: (Code snippet included showing Node2Vec implementation)
Output: (Image of Node2Vec output and visualization included)
ELMo (Embeddings from Language Models): ELMo generates dynamic word embeddings that change based on context, capturing syntactic and semantic nuances.
Code Example: (Reference to external article)
BERT and its Variants (SBERT, DistilBERT, RoBERTa): BERT uses a transformer architecture for bidirectional context understanding. SBERT is optimized for sentence comparison, DistilBERT is a lighter version, and RoBERTa improves BERT's training.
Code Example: (Code snippet included showing implementation of BERT variants)
Output: (Image of BERT variant output included)
CLIP and BLIP: CLIP and BLIP are multimodal models that generate embeddings for both images and text, enabling cross-modal understanding.
Code Example: (Code snippet included showing CLIP implementation)
Output: (Image of CLIP output included)
Embedding Comparison Table: (Table comparing all embedding methods included)
Conclusion: The article concludes by emphasizing the significant advancements in embedding techniques and the accessibility of these models for various applications. It highlights the importance of choosing the right embedding method based on specific needs and goals.
The above is the detailed content of 14 Powerful Techniques Defining the Evolution of Embedding - Analytics Vidhya. For more information, please follow other related articles on the PHP Chinese website!
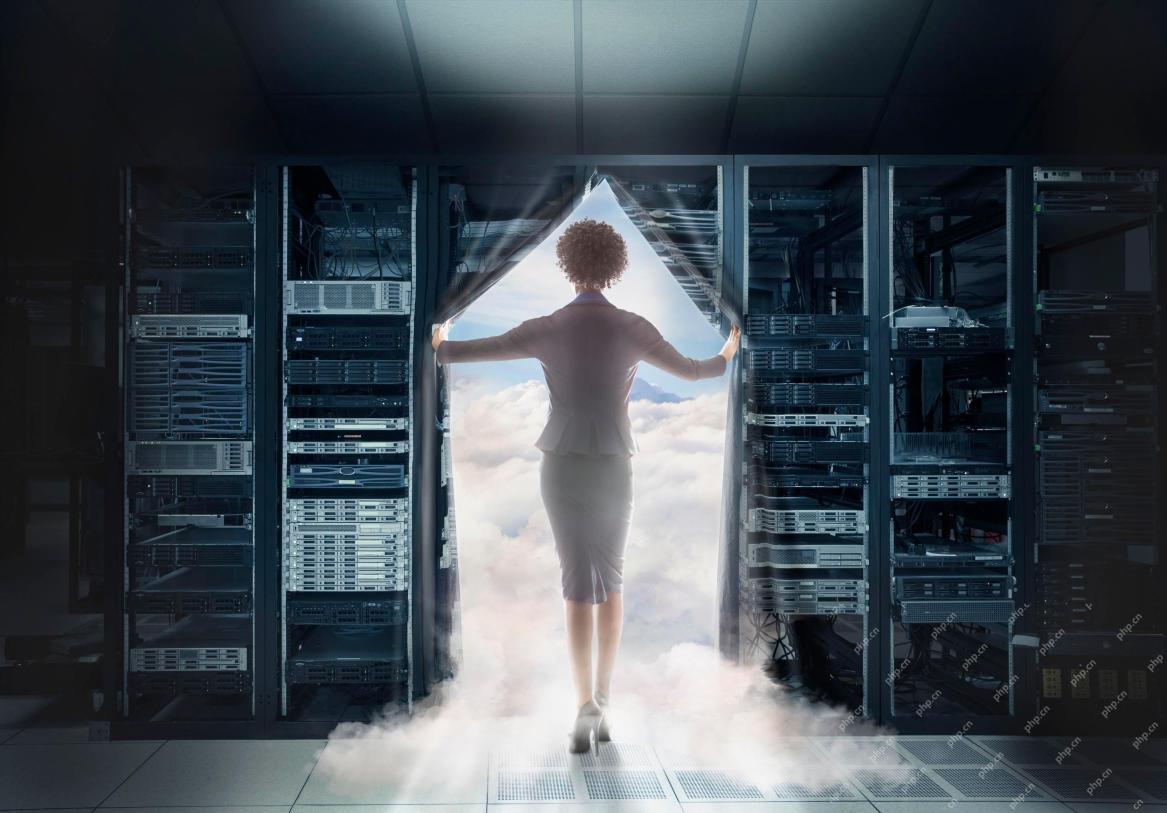
In John Rawls' seminal 1971 book The Theory of Justice, he proposed a thought experiment that we should take as the core of today's AI design and use decision-making: the veil of ignorance. This philosophy provides a simple tool for understanding equity and also provides a blueprint for leaders to use this understanding to design and implement AI equitably. Imagine that you are making rules for a new society. But there is a premise: you don’t know in advance what role you will play in this society. You may end up being rich or poor, healthy or disabled, belonging to a majority or marginal minority. Operating under this "veil of ignorance" prevents rule makers from making decisions that benefit themselves. On the contrary, people will be more motivated to formulate public
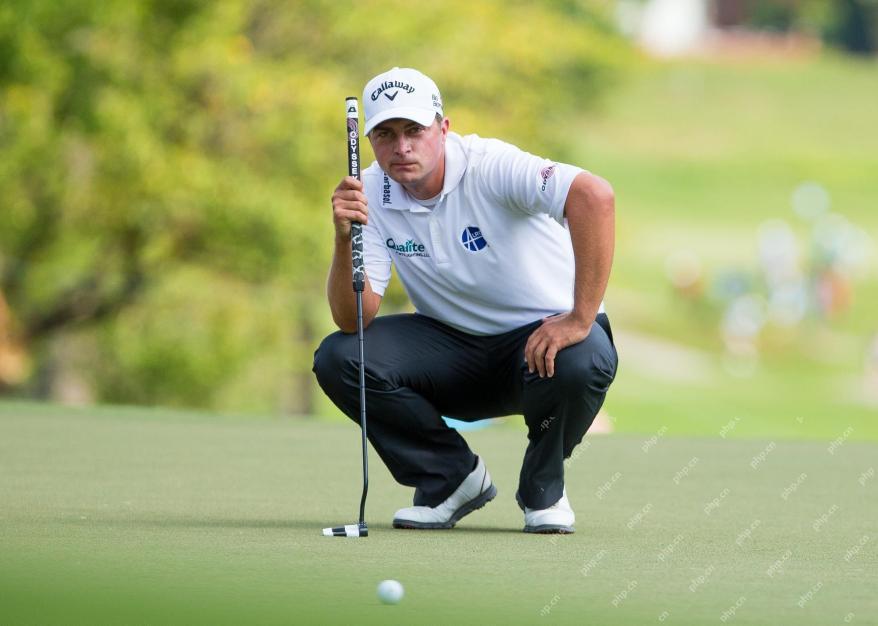
Numerous companies specialize in robotic process automation (RPA), offering bots to automate repetitive tasks—UiPath, Automation Anywhere, Blue Prism, and others. Meanwhile, process mining, orchestration, and intelligent document processing speciali
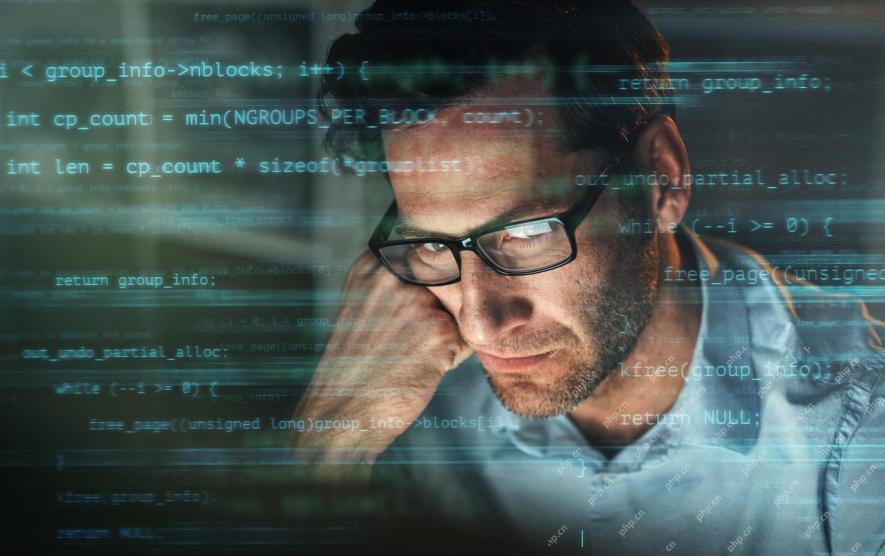
The future of AI is moving beyond simple word prediction and conversational simulation; AI agents are emerging, capable of independent action and task completion. This shift is already evident in tools like Anthropic's Claude. AI Agents: Research a
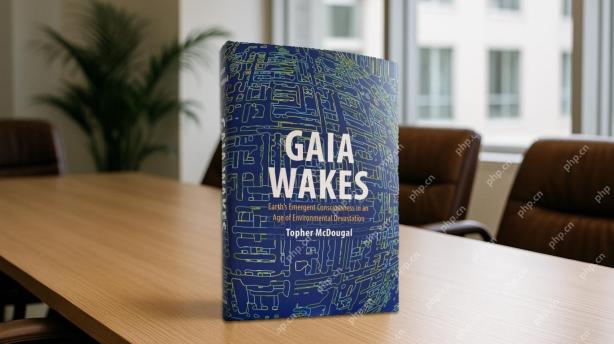
Rapid technological advancements necessitate a forward-looking perspective on the future of work. What happens when AI transcends mere productivity enhancement and begins shaping our societal structures? Topher McDougal's upcoming book, Gaia Wakes:
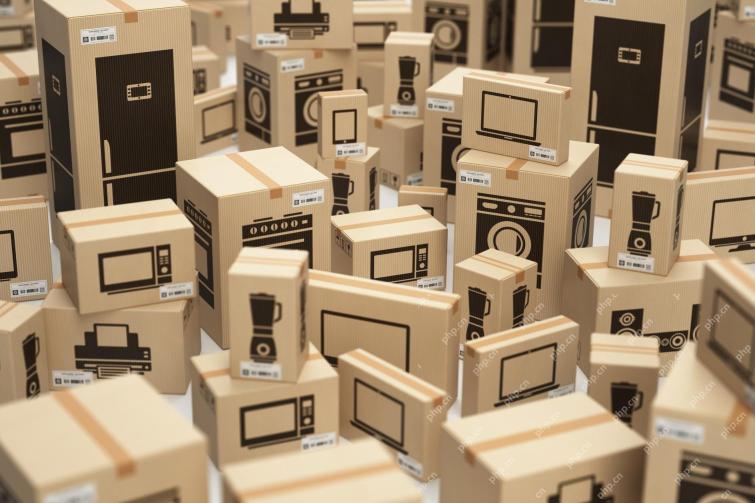
Product classification, often involving complex codes like "HS 8471.30" from systems such as the Harmonized System (HS), is crucial for international trade and domestic sales. These codes ensure correct tax application, impacting every inv
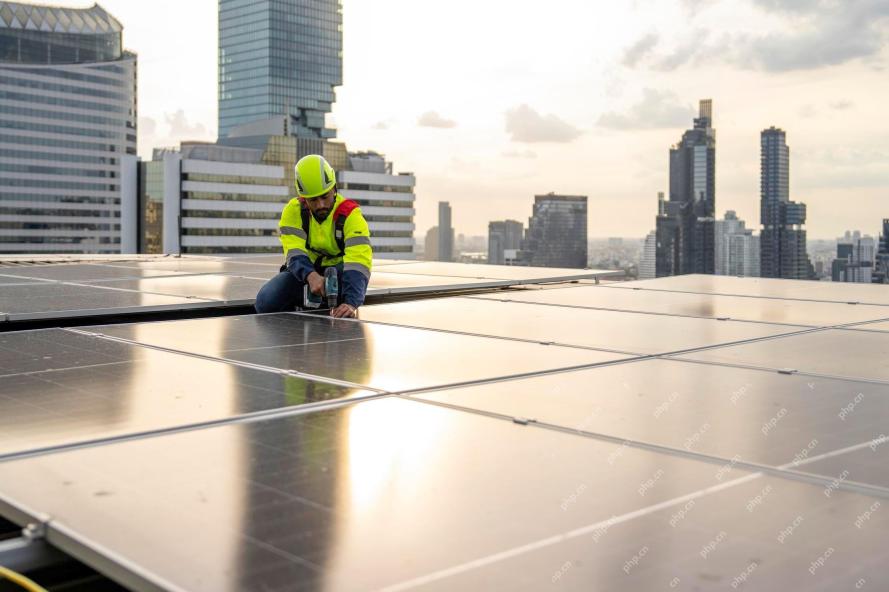
The future of energy consumption in data centers and climate technology investment This article explores the surge in energy consumption in AI-driven data centers and its impact on climate change, and analyzes innovative solutions and policy recommendations to address this challenge. Challenges of energy demand: Large and ultra-large-scale data centers consume huge power, comparable to the sum of hundreds of thousands of ordinary North American families, and emerging AI ultra-large-scale centers consume dozens of times more power than this. In the first eight months of 2024, Microsoft, Meta, Google and Amazon have invested approximately US$125 billion in the construction and operation of AI data centers (JP Morgan, 2024) (Table 1). Growing energy demand is both a challenge and an opportunity. According to Canary Media, the looming electricity
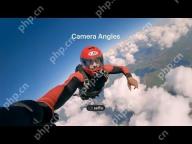
Generative AI is revolutionizing film and television production. Luma's Ray 2 model, as well as Runway's Gen-4, OpenAI's Sora, Google's Veo and other new models, are improving the quality of generated videos at an unprecedented speed. These models can easily create complex special effects and realistic scenes, even short video clips and camera-perceived motion effects have been achieved. While the manipulation and consistency of these tools still need to be improved, the speed of progress is amazing. Generative video is becoming an independent medium. Some models are good at animation production, while others are good at live-action images. It is worth noting that Adobe's Firefly and Moonvalley's Ma
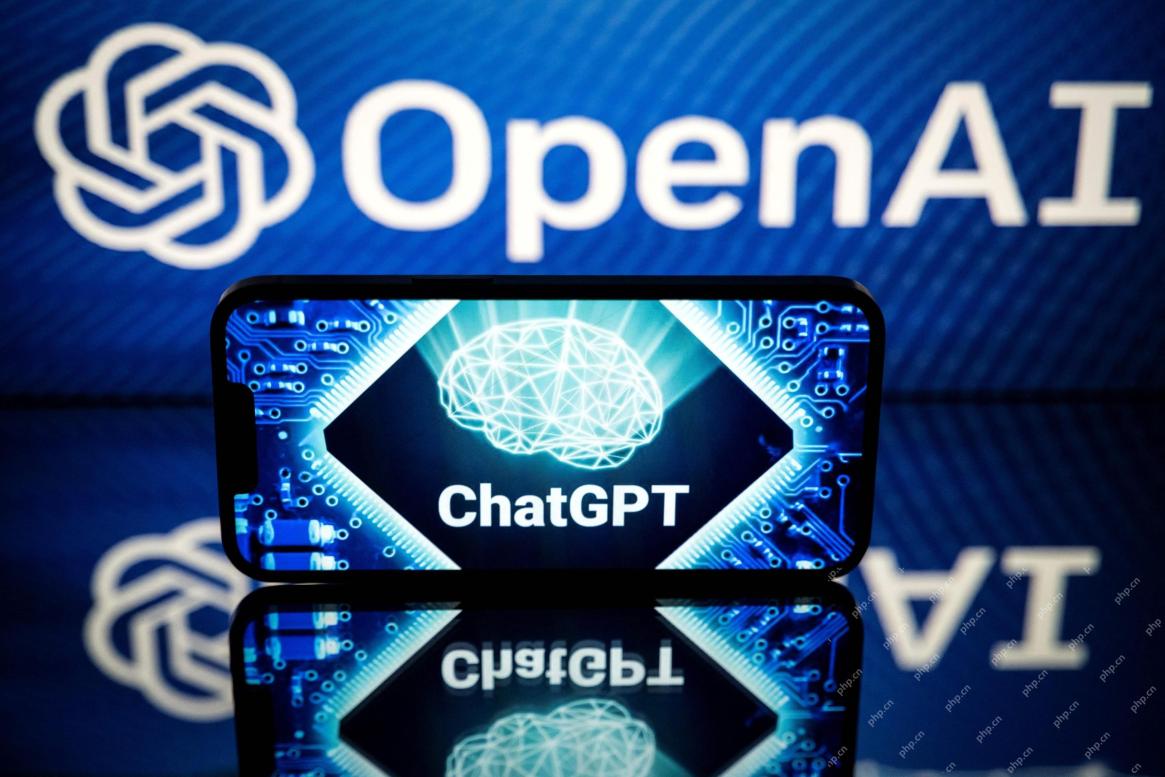
ChatGPT user experience declines: is it a model degradation or user expectations? Recently, a large number of ChatGPT paid users have complained about their performance degradation, which has attracted widespread attention. Users reported slower responses to models, shorter answers, lack of help, and even more hallucinations. Some users expressed dissatisfaction on social media, pointing out that ChatGPT has become “too flattering” and tends to verify user views rather than provide critical feedback. This not only affects the user experience, but also brings actual losses to corporate customers, such as reduced productivity and waste of computing resources. Evidence of performance degradation Many users have reported significant degradation in ChatGPT performance, especially in older models such as GPT-4 (which will soon be discontinued from service at the end of this month). this


Hot AI Tools

Undresser.AI Undress
AI-powered app for creating realistic nude photos

AI Clothes Remover
Online AI tool for removing clothes from photos.

Undress AI Tool
Undress images for free

Clothoff.io
AI clothes remover

Video Face Swap
Swap faces in any video effortlessly with our completely free AI face swap tool!

Hot Article

Hot Tools
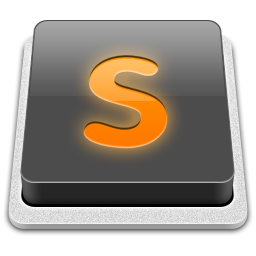
SublimeText3 Mac version
God-level code editing software (SublimeText3)

SAP NetWeaver Server Adapter for Eclipse
Integrate Eclipse with SAP NetWeaver application server.

Atom editor mac version download
The most popular open source editor

SecLists
SecLists is the ultimate security tester's companion. It is a collection of various types of lists that are frequently used during security assessments, all in one place. SecLists helps make security testing more efficient and productive by conveniently providing all the lists a security tester might need. List types include usernames, passwords, URLs, fuzzing payloads, sensitive data patterns, web shells, and more. The tester can simply pull this repository onto a new test machine and he will have access to every type of list he needs.

SublimeText3 Linux new version
SublimeText3 Linux latest version
