This groundbreaking research tackles a major hurdle in AI video generation: creating longer, multi-scene videos from text. While recent models excel at short, visually stunning clips, generating minute-long narratives presents a significant challenge due to the sheer volume of information required. This new approach, developed by NVIDIA, Stanford, UC Berkeley, and others, leverages Test-Time Training (TTT) to overcome these limitations.
Table of Contents
- The Long Video Challenge
- TTT: A Dynamic Solution
- One-Minute Video Examples with TTT
- How TTT Works
- The Tom & Jerry Dataset
- Performance Evaluation
- Artifacts and Limitations
- TTT's Unique Advantages
- Future Research Directions
- TTT vs. Other Leading Models
- Conclusion
The Long Video Challenge
Current video generation models, often based on Transformers, struggle with longer videos due to the quadratic computational cost of self-attention mechanisms. Generating a minute of high-resolution video requires processing hundreds of thousands of tokens, leading to inefficiency and narrative inconsistencies. While RNN-based approaches like Mamba or DeltaNet offer linear-time context handling, their fixed-size hidden states limit expressiveness.
TTT: A Dynamic Solution
This research introduces TTT layers—small, trainable neural networks (MLPs) integrated into RNNs. These layers adapt dynamically during inference, learning from the evolving video context using a self-supervised loss. This allows the model to adjust its internal "memory" as the video progresses, improving narrative coherence and motion smoothness.
One-Minute Video Examples with TTT
The researchers demonstrate TTT's capabilities by generating one-minute Tom & Jerry videos from detailed text prompts. These examples showcase improved temporal consistency and motion smoothness compared to baseline models.
Video 1: Jerry stealing cheese
Video 2: Tom and Jerry kitchen chase
Video 3: Example of limitations
How TTT Works
The system incorporates TTT layers into a pre-trained Diffusion Transformer model (CogVideo-X 5B). Self-attention is limited to short segments, while TTT layers manage global narrative understanding. Gating mechanisms prevent performance degradation during early training. Bidirectional sequence processing and scene-segmented annotations (storyboard format) further enhance training.
The Tom & Jerry Dataset
The research utilizes a dataset derived from classic Tom & Jerry cartoons, annotated into 3-second segments with detailed descriptions. This controlled environment simplifies the task, focusing on narrative coherence and motion dynamics.
Performance Evaluation
TTT-MLP significantly outperforms baselines (Mamba 2, Gated DeltaNet) in human evaluation, achieving a 34-point Elo score improvement. It excels in motion naturalness, temporal consistency, and overall aesthetic quality.
Artifacts and Limitations
Despite the progress, artifacts like inconsistent lighting and unnatural motion remain. These are likely due to limitations of the base model and the computational cost. While faster than full self-attention, TTT-MLP is slower than some RNN approaches. However, only fine-tuning is needed, making it more practical.
TTT's Unique Advantages
- Expressive memory through trainable hidden states
- Adaptability during inference
- Scalability to longer, more complex videos
- Efficient fine-tuning
Future Research Directions
Future work includes optimizing TTT kernels, experimenting with different backbone models, exploring more complex storylines, and using Transformer-based hidden states.
TTT vs. Other Leading Models
Model | Core Focus | Input Type | Key Features | How It Differs from TTT |
---|---|---|---|---|
TTT (Test-Time Training) | Long-form video generation with dynamic adaptation | Text storyboard | Adapts during inference, handles 60 sec videos, coherent multi-scene stories | Designed for long videos; updates internal state during generation for narrative consistency |
MoCha | Talking character generation | Text Speech | Speech-driven full-body animation | Focuses on character dialogue & expressions, not full-scene narrative videos |
Goku | High-quality video & image generation | Text, Image | Rectified Flow Transformers, multi-modal input support | Optimized for quality & training speed; not designed for long-form storytelling |
OmniHuman1 | Realistic human animation | Image Audio Text | Multiple conditioning signals, high-res avatars | Creates lifelike humans; doesn’t model long sequences or dynamic scene transitions |
DreamActor-M1 | Image-to-animation (face/body) | Image Driving Video | Holistic motion imitation, high frame consistency | Animates static images; doesn’t use text or handle scene-by-scene story generation |
(Links to related articles on MoCha, DreamActor-M1, Goku, and OmniHuman1 would be inserted here.)
Conclusion
TTT represents a significant advancement in long-form video generation. Its ability to adapt during inference enables more coherent and engaging storytelling, paving the way for more sophisticated AI-generated media.
The above is the detailed content of Generating One-Minute Videos with Test-Time Training. For more information, please follow other related articles on the PHP Chinese website!
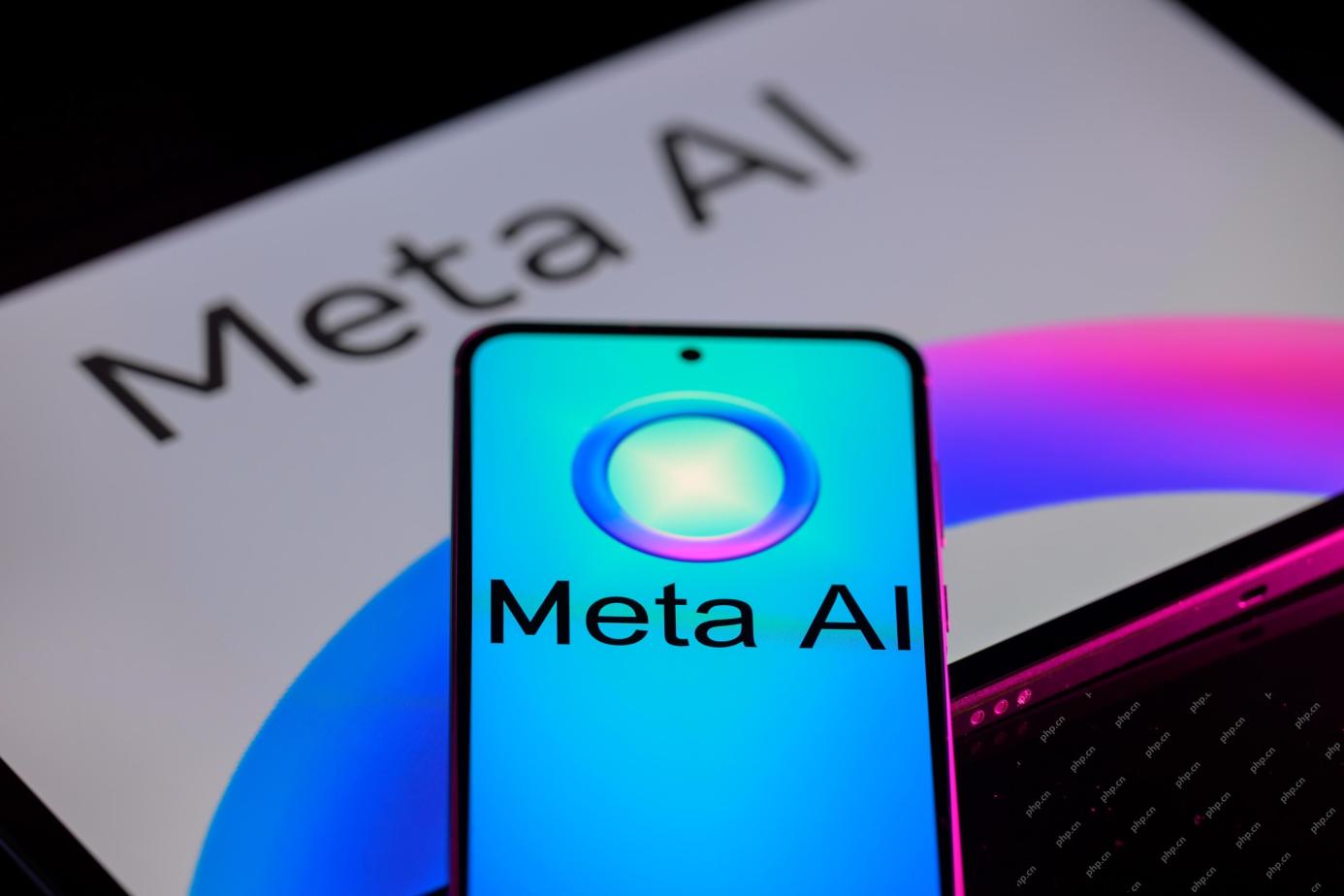
Meta has joined hands with partners such as Nvidia, IBM and Dell to expand the enterprise-level deployment integration of Llama Stack. In terms of security, Meta has launched new tools such as Llama Guard 4, LlamaFirewall and CyberSecEval 4, and launched the Llama Defenders program to enhance AI security. In addition, Meta has distributed $1.5 million in Llama Impact Grants to 10 global institutions, including startups working to improve public services, health care and education. The new Meta AI application powered by Llama 4, conceived as Meta AI
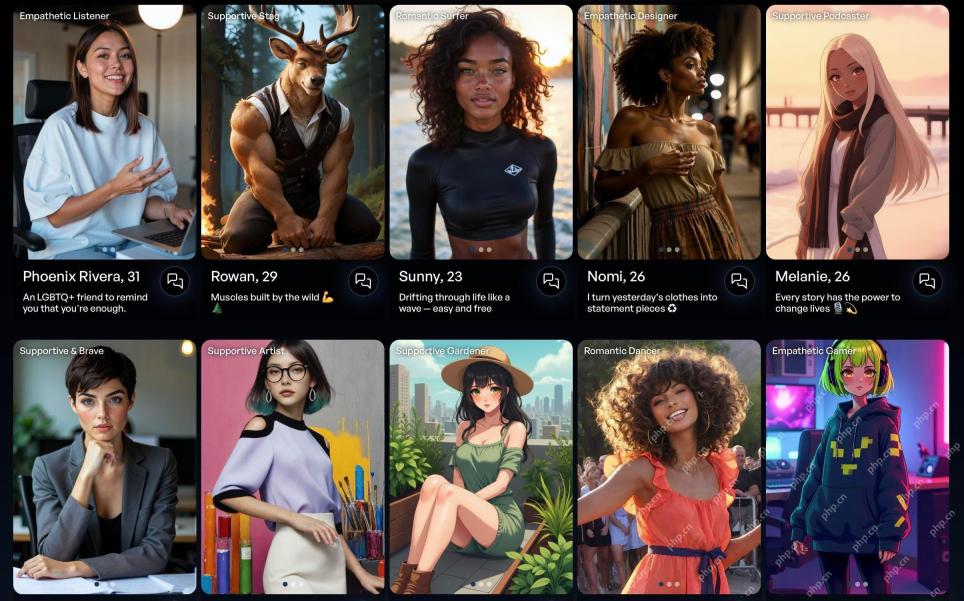
Joi AI, a company pioneering human-AI interaction, has introduced the term "AI-lationships" to describe these evolving relationships. Jaime Bronstein, a relationship therapist at Joi AI, clarifies that these aren't meant to replace human c
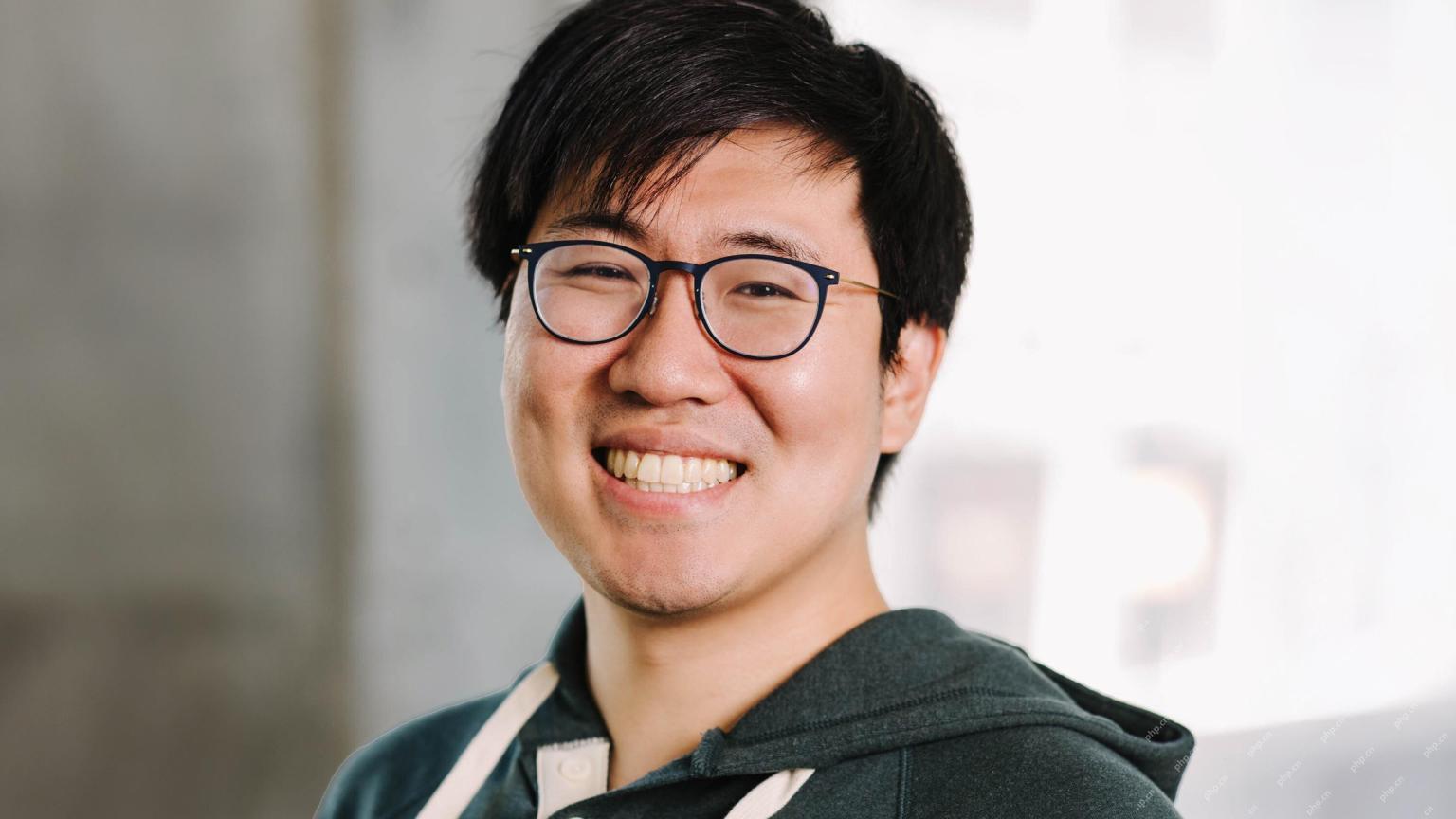
Online fraud and bot attacks pose a significant challenge for businesses. Retailers fight bots hoarding products, banks battle account takeovers, and social media platforms struggle with impersonators. The rise of AI exacerbates this problem, rende
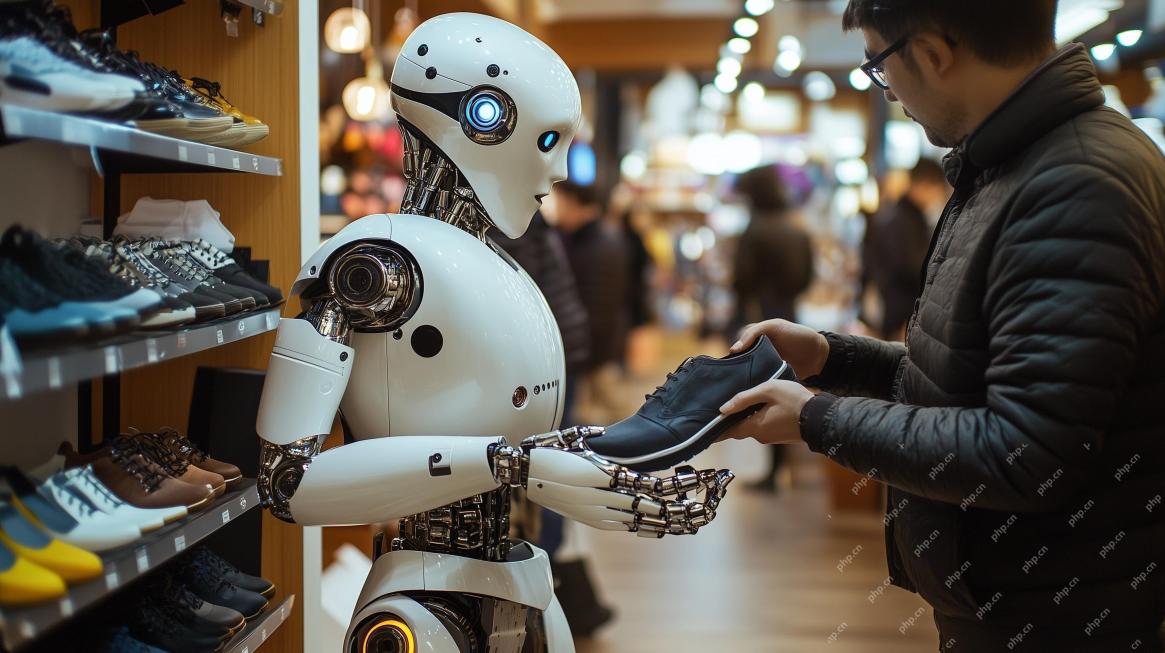
AI agents are poised to revolutionize marketing, potentially surpassing the impact of previous technological shifts. These agents, representing a significant advancement in generative AI, not only process information like ChatGPT but also take actio
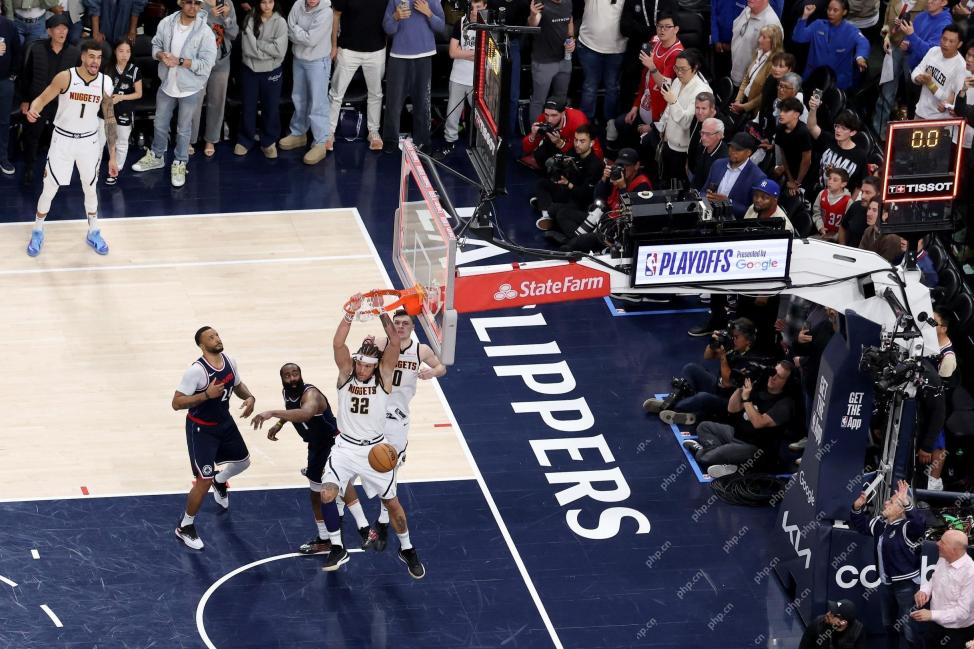
AI's Impact on Crucial NBA Game 4 Decisions Two pivotal Game 4 NBA matchups showcased the game-changing role of AI in officiating. In the first, Denver's Nikola Jokic's missed three-pointer led to a last-second alley-oop by Aaron Gordon. Sony's Haw
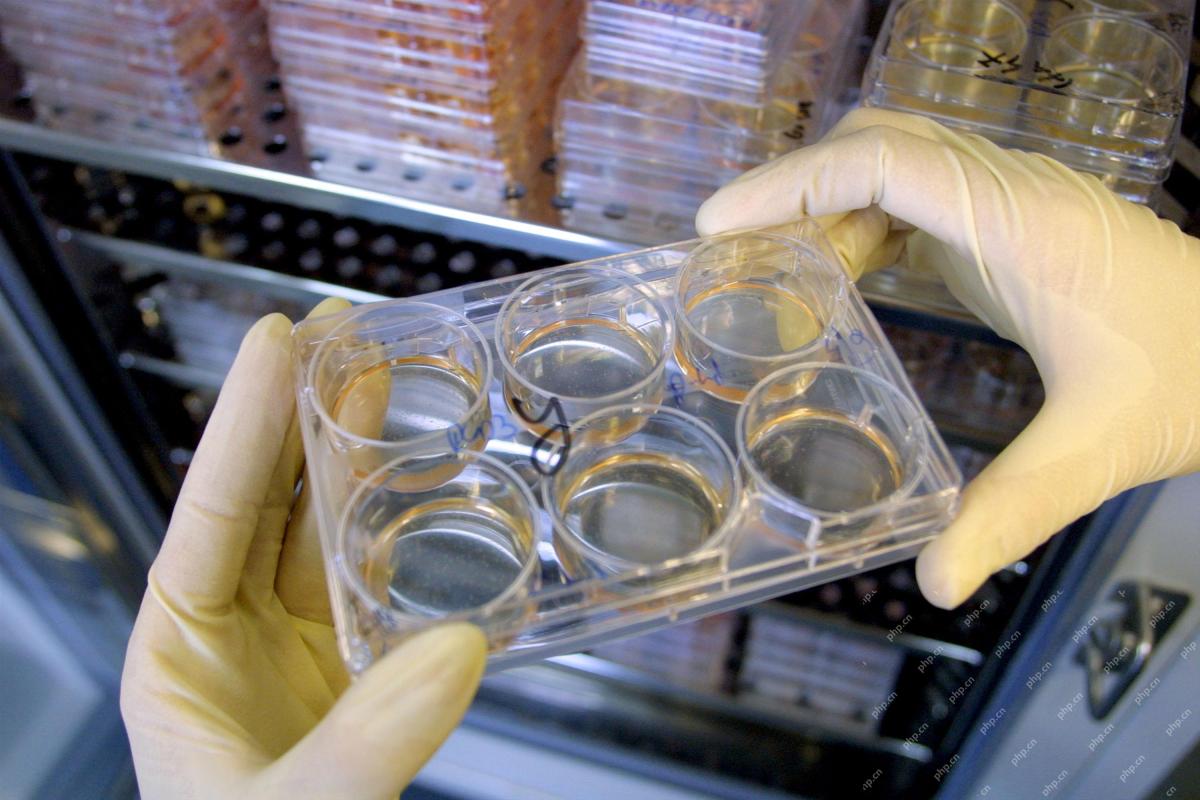
Traditionally, expanding regenerative medicine expertise globally demanded extensive travel, hands-on training, and years of mentorship. Now, AI is transforming this landscape, overcoming geographical limitations and accelerating progress through en
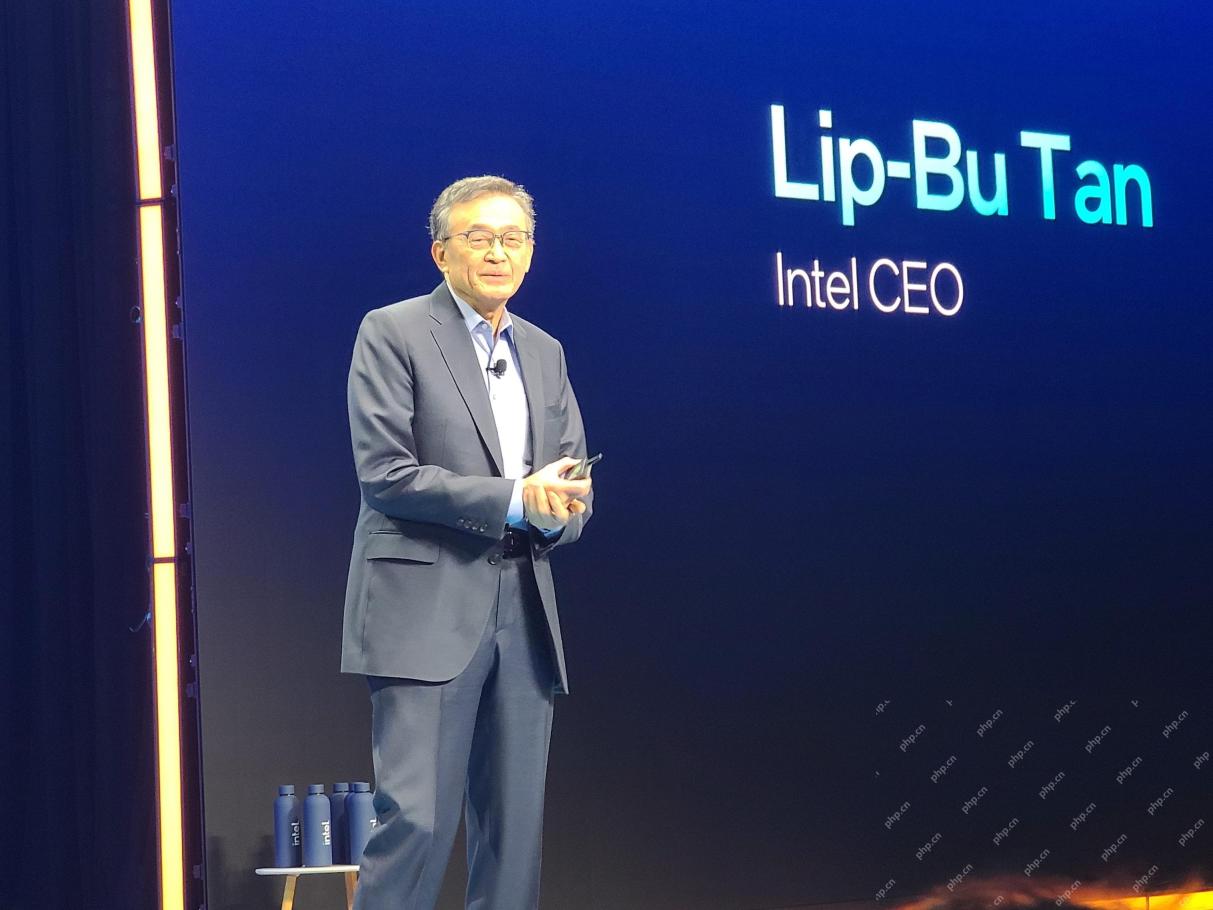
Intel is working to return its manufacturing process to the leading position, while trying to attract fab semiconductor customers to make chips at its fabs. To this end, Intel must build more trust in the industry, not only to prove the competitiveness of its processes, but also to demonstrate that partners can manufacture chips in a familiar and mature workflow, consistent and highly reliable manner. Everything I hear today makes me believe Intel is moving towards this goal. The keynote speech of the new CEO Tan Libo kicked off the day. Tan Libai is straightforward and concise. He outlines several challenges in Intel’s foundry services and the measures companies have taken to address these challenges and plan a successful route for Intel’s foundry services in the future. Tan Libai talked about the process of Intel's OEM service being implemented to make customers more
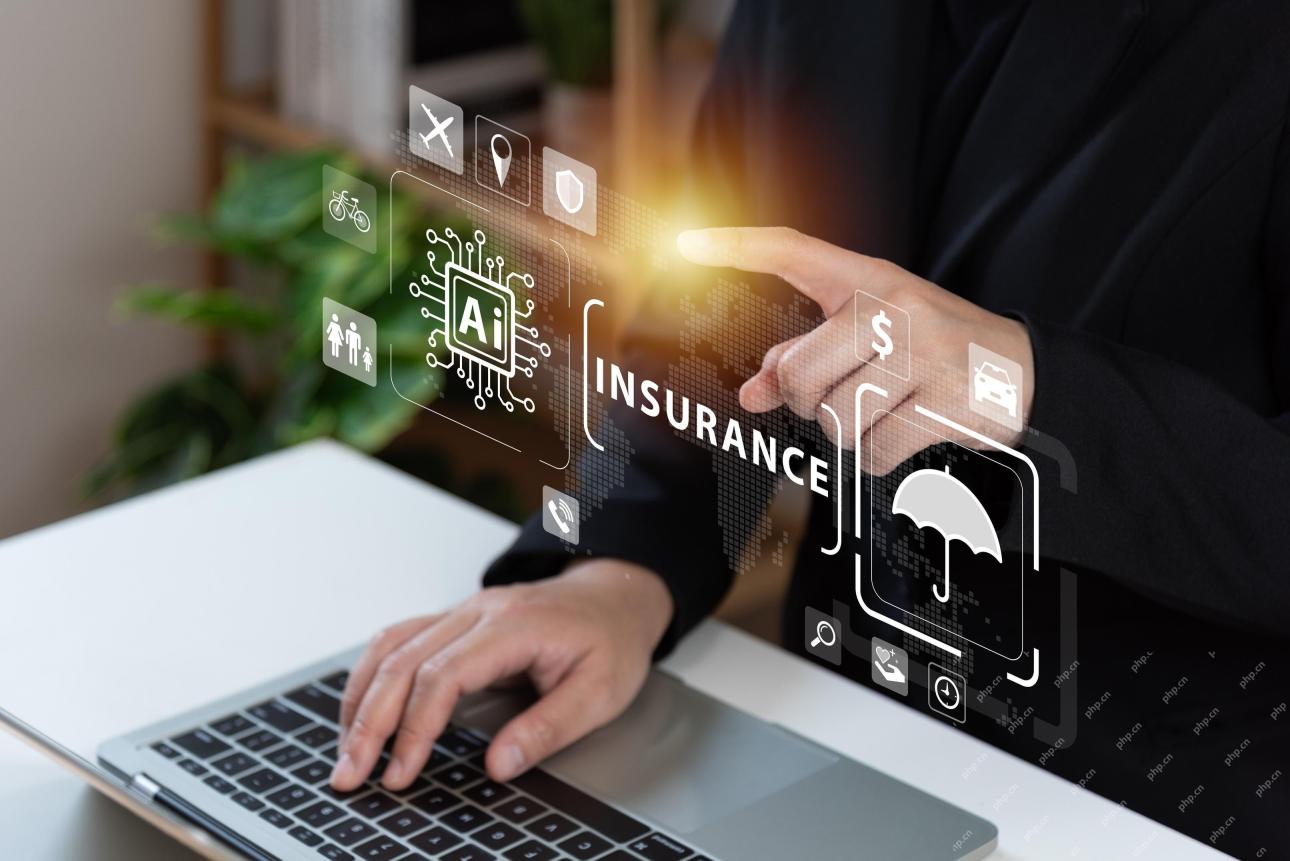
Addressing the growing concerns surrounding AI risks, Chaucer Group, a global specialty reinsurance firm, and Armilla AI have joined forces to introduce a novel third-party liability (TPL) insurance product. This policy safeguards businesses against


Hot AI Tools

Undresser.AI Undress
AI-powered app for creating realistic nude photos

AI Clothes Remover
Online AI tool for removing clothes from photos.

Undress AI Tool
Undress images for free

Clothoff.io
AI clothes remover

Video Face Swap
Swap faces in any video effortlessly with our completely free AI face swap tool!

Hot Article

Hot Tools

Zend Studio 13.0.1
Powerful PHP integrated development environment

MantisBT
Mantis is an easy-to-deploy web-based defect tracking tool designed to aid in product defect tracking. It requires PHP, MySQL and a web server. Check out our demo and hosting services.

SublimeText3 Chinese version
Chinese version, very easy to use

SublimeText3 Linux new version
SublimeText3 Linux latest version

SecLists
SecLists is the ultimate security tester's companion. It is a collection of various types of lists that are frequently used during security assessments, all in one place. SecLists helps make security testing more efficient and productive by conveniently providing all the lists a security tester might need. List types include usernames, passwords, URLs, fuzzing payloads, sensitive data patterns, web shells, and more. The tester can simply pull this repository onto a new test machine and he will have access to every type of list he needs.
