Streamline AI Model Deployment with LitServe: A Comprehensive Guide
Serving machine learning models efficiently is crucial for real-time applications. While FastAPI excels at building RESTful APIs, it lacks the specialized features needed for optimal AI model deployment, particularly with resource-intensive models like Large Language Models (LLMs). LitServe, an open-source model serving engine built on FastAPI, addresses this gap by providing advanced functionalities for scalability and performance. This article explores LitServe's capabilities and demonstrates its use in creating high-performance AI servers.
Key Learning Outcomes
This guide will equip you with the knowledge to:
- Easily deploy and serve AI models using LitServe.
- Leverage batching, streaming, and GPU acceleration for enhanced model performance.
- Build a simple AI server through a practical example.
- Optimize model serving for high throughput and scalability.
This article is part of the Data Science Blogathon.
Understanding Model Serving and LitServe
Model serving is the process of deploying trained machine learning models into production environments, often via APIs, to enable real-time prediction generation. Challenges include managing high computational demands (especially with LLMs), optimizing resource utilization, and maintaining performance under varying loads.
LitServe simplifies this process by offering a fast, flexible, and scalable solution. It handles complex tasks like scaling, batching, and streaming, eliminating the need to build custom FastAPI servers for each model. It's compatible with local machines, cloud environments, and high-performance computing clusters.
LitServe's Core Features
- Accelerated Model Serving: LitServe significantly improves model serving speed compared to traditional methods.
- Multi-GPU Support: Utilizes multiple GPUs for parallel processing, minimizing latency.
- Batching and Streaming: Processes multiple requests concurrently (batching) or handles large responses efficiently (streaming).
LitServe also offers features like authentication and OpenAI specification compatibility, catering to diverse AI workloads.
Getting Started: A Simple Example
Install LitServe:
pip install litserve
A basic LitServe API can be defined as follows:
import litserve as ls class SimpleLitAPI(ls.LitAPI): def setup(self, device): self.model1 = lambda x: x**2 self.model2 = lambda x: x**3 def decode_request(self, request): return request["input"] def predict(self, x): squared = self.model1(x) cubed = self.model2(x) output = squared cubed return {"output": output} def encode_response(self, output): return {"output": output} if __name__ == "__main__": api = SimpleLitAPI() server = ls.LitServer(api, accelerator="gpu") # or "auto" server.run(port=8000)
This example demonstrates the core components: setup
(model initialization), decode_request
(input processing), predict
(inference), and encode_response
(output formatting).
Serving a Vision Model: Image Captioning
This section showcases LitServe's capabilities with a more realistic scenario: deploying an image captioning model from Hugging Face. (The full code is available on GitHub – [link to GitHub repo]).
The key steps involve:
- Loading the Model: Load a pre-trained VisionEncoderDecoderModel (e.g., from Hugging Face).
- Defining the LitAPI: Create a custom LitAPI class to handle image loading, preprocessing, caption generation, and response formatting.
- Running the Server: Instantiate the LitAPI and LitServer, specifying GPU acceleration if available.
Performance Optimization with Advanced Features
LitServe offers several features to optimize performance:
-
Batching: Process multiple requests simultaneously using
max_batch_size
inLitServer
. -
Streaming: Handle large inputs efficiently with
stream=True
. -
Device Management: Control GPU usage with the
devices
parameter.
Why Choose LitServe?
LitServe stands out due to its:
- Scalability: Easily handles increasing workloads.
- Optimized Performance: Batching, streaming, and GPU acceleration maximize throughput and minimize latency.
- Ease of Use: Simplifies model deployment.
- Advanced Feature Support: Provides features for complex AI applications.
Conclusion
LitServe simplifies AI model deployment, allowing developers to focus on building robust AI solutions. Its scalability, performance optimizations, and ease of use make it a valuable tool for various AI projects.
Key Takeaways
- LitServe simplifies AI model serving.
- Advanced features enhance performance.
- Suitable for various deployment environments.
- Supports complex AI workloads.
References
Frequently Asked Questions (FAQs)
-
Q1: LitServe vs. FastAPI? LitServe builds upon FastAPI's strengths but adds crucial features for efficient AI model serving, especially for resource-intensive models.
-
Q2: CPU/GPU Support? LitServe supports both.
-
Q3: Benefits of Batching? Improves throughput by processing multiple requests concurrently.
-
Q4: Model Compatibility? Supports various models (machine learning, deep learning, LLMs) and integrates with popular frameworks (PyTorch, TensorFlow, Hugging Face).
-
Q5: Integration with Existing Pipelines? Easy integration due to its FastAPI-based API and customizable
LitAPI
class.
(Note: Replace bracketed placeholders with actual links.)
The above is the detailed content of LitServe: The Future of Scalable AI Model Serving. For more information, please follow other related articles on the PHP Chinese website!
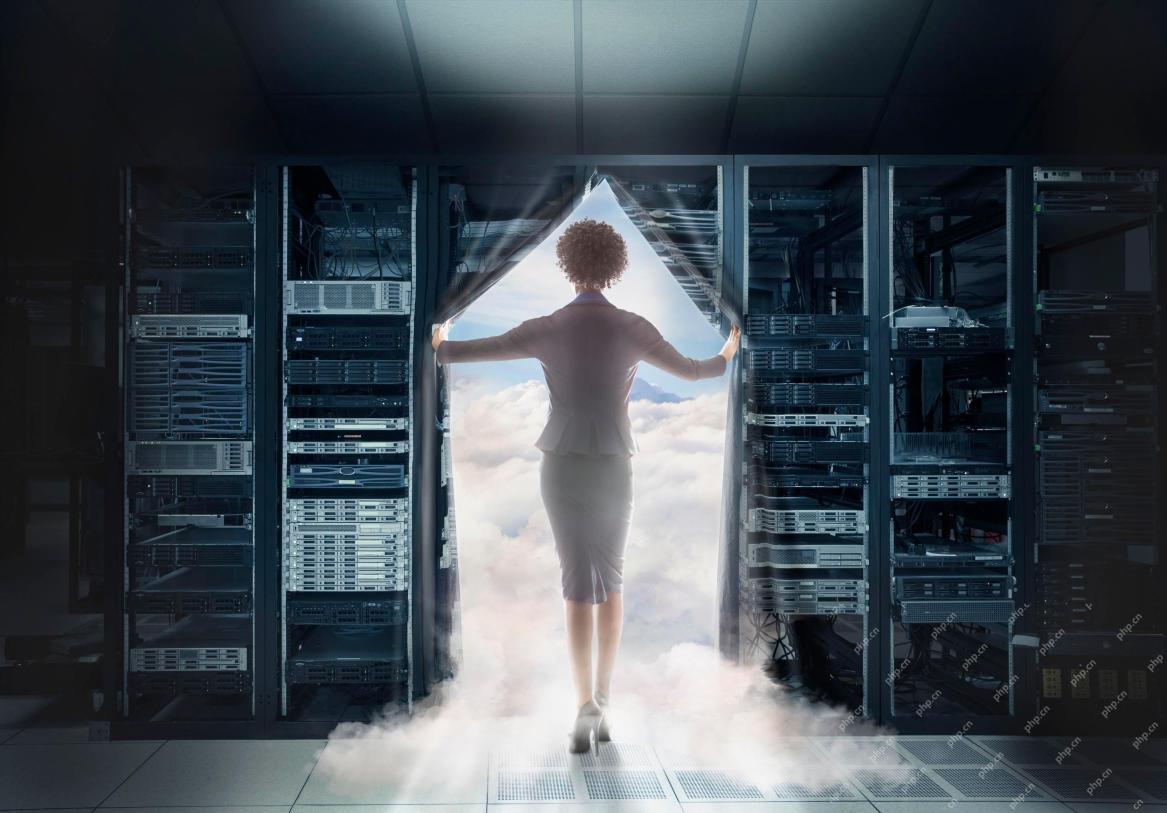
In John Rawls' seminal 1971 book The Theory of Justice, he proposed a thought experiment that we should take as the core of today's AI design and use decision-making: the veil of ignorance. This philosophy provides a simple tool for understanding equity and also provides a blueprint for leaders to use this understanding to design and implement AI equitably. Imagine that you are making rules for a new society. But there is a premise: you don’t know in advance what role you will play in this society. You may end up being rich or poor, healthy or disabled, belonging to a majority or marginal minority. Operating under this "veil of ignorance" prevents rule makers from making decisions that benefit themselves. On the contrary, people will be more motivated to formulate public
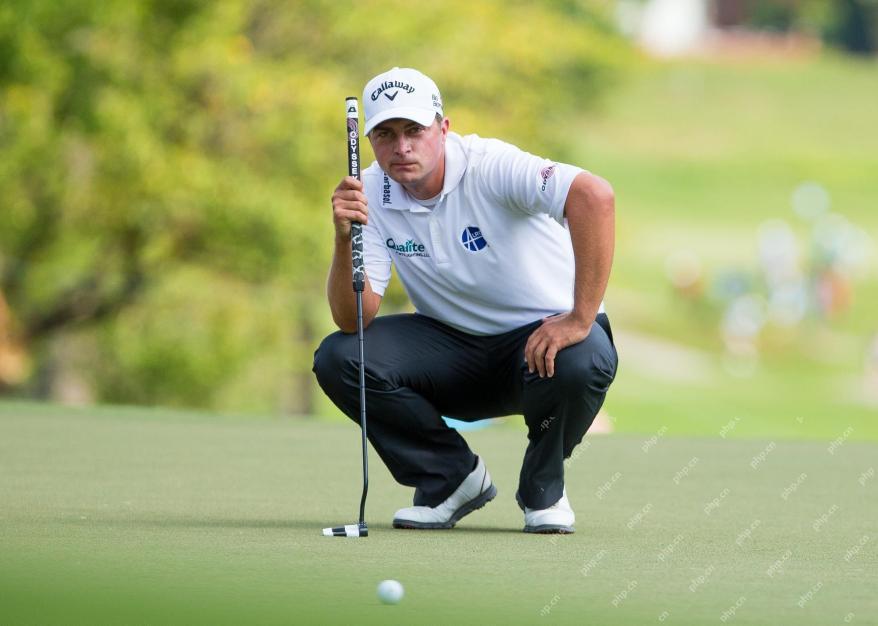
Numerous companies specialize in robotic process automation (RPA), offering bots to automate repetitive tasks—UiPath, Automation Anywhere, Blue Prism, and others. Meanwhile, process mining, orchestration, and intelligent document processing speciali
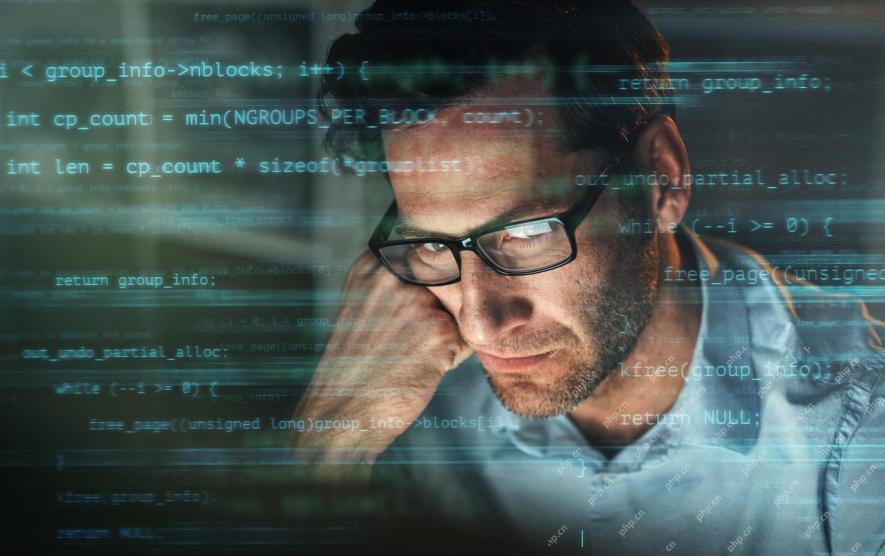
The future of AI is moving beyond simple word prediction and conversational simulation; AI agents are emerging, capable of independent action and task completion. This shift is already evident in tools like Anthropic's Claude. AI Agents: Research a
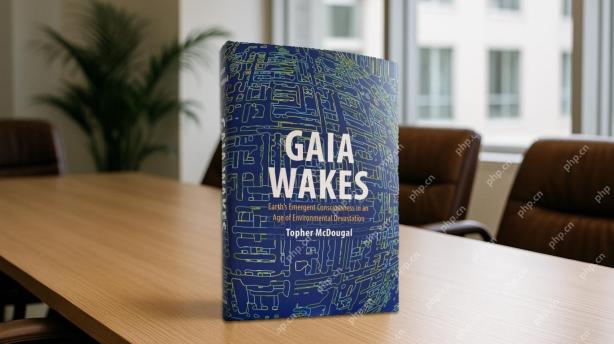
Rapid technological advancements necessitate a forward-looking perspective on the future of work. What happens when AI transcends mere productivity enhancement and begins shaping our societal structures? Topher McDougal's upcoming book, Gaia Wakes:
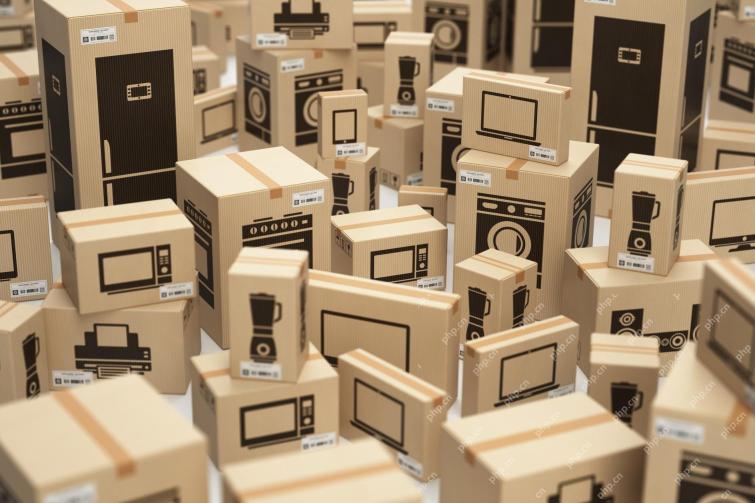
Product classification, often involving complex codes like "HS 8471.30" from systems such as the Harmonized System (HS), is crucial for international trade and domestic sales. These codes ensure correct tax application, impacting every inv
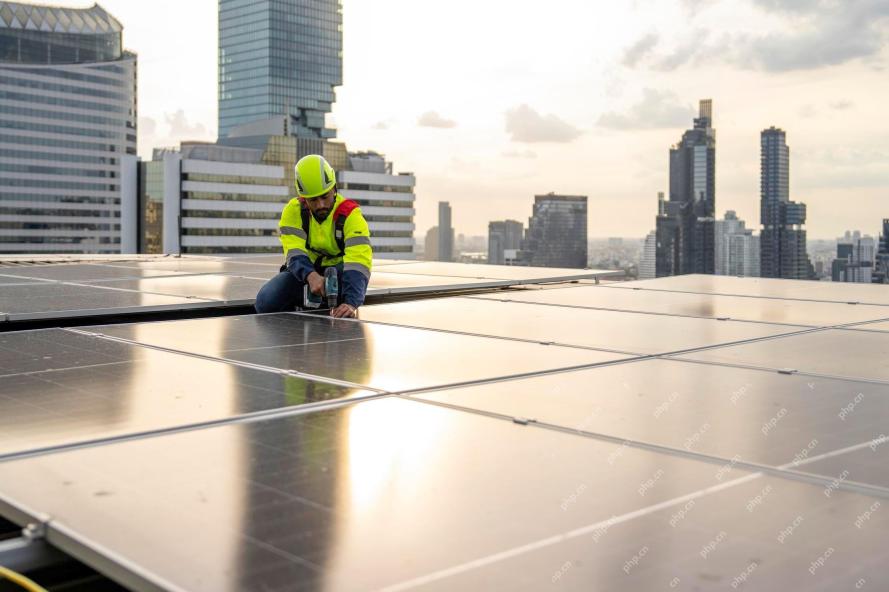
The future of energy consumption in data centers and climate technology investment This article explores the surge in energy consumption in AI-driven data centers and its impact on climate change, and analyzes innovative solutions and policy recommendations to address this challenge. Challenges of energy demand: Large and ultra-large-scale data centers consume huge power, comparable to the sum of hundreds of thousands of ordinary North American families, and emerging AI ultra-large-scale centers consume dozens of times more power than this. In the first eight months of 2024, Microsoft, Meta, Google and Amazon have invested approximately US$125 billion in the construction and operation of AI data centers (JP Morgan, 2024) (Table 1). Growing energy demand is both a challenge and an opportunity. According to Canary Media, the looming electricity
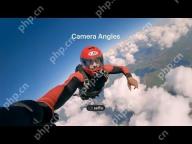
Generative AI is revolutionizing film and television production. Luma's Ray 2 model, as well as Runway's Gen-4, OpenAI's Sora, Google's Veo and other new models, are improving the quality of generated videos at an unprecedented speed. These models can easily create complex special effects and realistic scenes, even short video clips and camera-perceived motion effects have been achieved. While the manipulation and consistency of these tools still need to be improved, the speed of progress is amazing. Generative video is becoming an independent medium. Some models are good at animation production, while others are good at live-action images. It is worth noting that Adobe's Firefly and Moonvalley's Ma
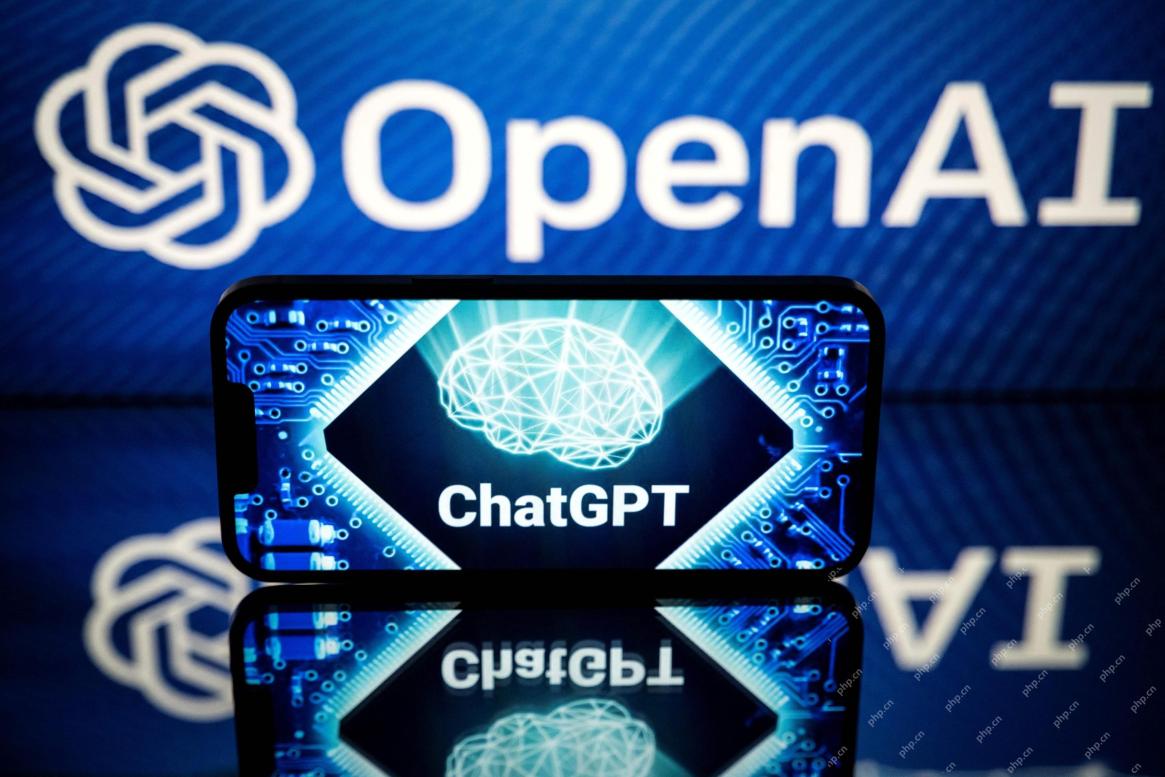
ChatGPT user experience declines: is it a model degradation or user expectations? Recently, a large number of ChatGPT paid users have complained about their performance degradation, which has attracted widespread attention. Users reported slower responses to models, shorter answers, lack of help, and even more hallucinations. Some users expressed dissatisfaction on social media, pointing out that ChatGPT has become “too flattering” and tends to verify user views rather than provide critical feedback. This not only affects the user experience, but also brings actual losses to corporate customers, such as reduced productivity and waste of computing resources. Evidence of performance degradation Many users have reported significant degradation in ChatGPT performance, especially in older models such as GPT-4 (which will soon be discontinued from service at the end of this month). this


Hot AI Tools

Undresser.AI Undress
AI-powered app for creating realistic nude photos

AI Clothes Remover
Online AI tool for removing clothes from photos.

Undress AI Tool
Undress images for free

Clothoff.io
AI clothes remover

Video Face Swap
Swap faces in any video effortlessly with our completely free AI face swap tool!

Hot Article

Hot Tools

SAP NetWeaver Server Adapter for Eclipse
Integrate Eclipse with SAP NetWeaver application server.

Zend Studio 13.0.1
Powerful PHP integrated development environment

Atom editor mac version download
The most popular open source editor
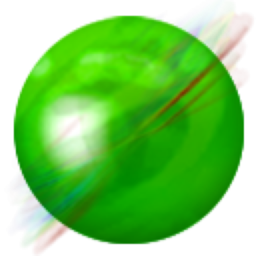
ZendStudio 13.5.1 Mac
Powerful PHP integrated development environment

mPDF
mPDF is a PHP library that can generate PDF files from UTF-8 encoded HTML. The original author, Ian Back, wrote mPDF to output PDF files "on the fly" from his website and handle different languages. It is slower than original scripts like HTML2FPDF and produces larger files when using Unicode fonts, but supports CSS styles etc. and has a lot of enhancements. Supports almost all languages, including RTL (Arabic and Hebrew) and CJK (Chinese, Japanese and Korean). Supports nested block-level elements (such as P, DIV),
