


What are some common monitoring tools for Python applications?
There are several common monitoring tools that are widely used for monitoring Python applications. Here are some of the most popular ones:
- Prometheus: Prometheus is an open-source monitoring and alerting toolkit that is highly popular for monitoring Python applications. It collects metrics from configured targets at given intervals, evaluates rule expressions, displays the results, and can trigger alerts if some condition is observed to be true.
- Grafana: Often used in conjunction with Prometheus, Grafana is an open-source platform for monitoring and observability. It allows you to query, visualize, alert on, and understand your metrics no matter where they are stored.
- New Relic: New Relic is a comprehensive monitoring tool that provides deep insights into the performance of Python applications. It offers real-time monitoring, application performance management (APM), and infrastructure monitoring.
- Datadog: Datadog is another popular monitoring and analytics platform that provides full-stack observability for large-scale Python applications. It integrates well with various other tools and services, making it a versatile choice for monitoring.
- Sentry: Sentry is primarily known for error tracking and monitoring, but it also provides performance monitoring features for Python applications. It helps developers to identify and fix issues quickly.
- Elastic APM: Part of the Elastic Stack, Elastic APM provides application performance monitoring for Python applications. It helps in tracking the performance of your application and identifying bottlenecks.
What are the key features to look for in Python application monitoring tools?
When selecting a monitoring tool for Python applications, it's important to consider the following key features:
- Real-time Monitoring: The ability to monitor your application in real-time is crucial for identifying and resolving issues quickly. Real-time data helps in understanding the current state of your application.
- Performance Metrics: The tool should be able to collect and display a wide range of performance metrics such as CPU usage, memory consumption, response times, and throughput. These metrics are essential for diagnosing performance issues.
- Alerting and Notifications: Effective monitoring tools should have robust alerting mechanisms that notify you when certain thresholds are breached or when anomalies are detected. This helps in proactive issue resolution.
- Scalability: The tool should be able to scale with your application. As your Python project grows, the monitoring tool should be able to handle increased load and complexity without performance degradation.
- Integration Capabilities: The ability to integrate with other tools and services in your tech stack is important. This includes integration with logging tools, CI/CD pipelines, and other monitoring systems.
- Ease of Use: The tool should have a user-friendly interface that makes it easy to set up, configure, and navigate. A steep learning curve can hinder the adoption and effectiveness of the tool.
- Customization: The ability to customize dashboards, alerts, and metrics according to your specific needs is crucial. Different applications may require different monitoring parameters.
- Historical Data and Trends: Access to historical data and the ability to analyze trends over time can help in understanding long-term performance patterns and making informed decisions.
How can monitoring tools help improve the performance of Python applications?
Monitoring tools play a crucial role in improving the performance of Python applications in several ways:
- Identifying Bottlenecks: Monitoring tools help in identifying performance bottlenecks by providing detailed metrics on various aspects of the application. For example, if a particular function is consuming too much CPU time, the tool can highlight this, allowing developers to optimize that part of the code.
- Proactive Issue Resolution: With real-time monitoring and alerting, issues can be detected and resolved before they impact users. This proactive approach helps in maintaining high performance and availability of the application.
- Resource Optimization: By monitoring resource usage such as CPU, memory, and disk I/O, developers can optimize resource allocation. This can lead to better utilization of available resources and improved overall performance.
- Load Balancing: Monitoring tools can help in understanding the load distribution across different parts of the application. This information can be used to implement effective load balancing strategies, ensuring that no single component becomes a performance bottleneck.
- Performance Tuning: Historical data and trend analysis provided by monitoring tools can help in performance tuning. By understanding how the application has performed over time, developers can make informed decisions about where to focus optimization efforts.
- Error Tracking and Resolution: Tools like Sentry not only monitor performance but also track errors. By quickly identifying and resolving errors, the overall performance and reliability of the application can be improved.
- Scalability Planning: Monitoring tools provide insights into how the application scales under different loads. This information is crucial for planning future scalability improvements and ensuring that the application can handle increased traffic without performance degradation.
Which monitoring tools are best suited for different sizes of Python projects?
The choice of monitoring tool can vary depending on the size and complexity of your Python project. Here's a breakdown of which tools might be best suited for different sizes of projects:
-
Small Projects:
- Sentry: For small projects, Sentry is an excellent choice due to its ease of setup and focus on error tracking. It's free for small projects and provides essential performance monitoring features.
- Elastic APM: Elastic APM is also suitable for small projects, especially if you're already using other parts of the Elastic Stack. It's lightweight and easy to integrate.
-
Medium Projects:
- New Relic: New Relic offers a good balance of features and ease of use, making it suitable for medium-sized projects. It provides comprehensive monitoring and performance insights without being overly complex.
- Datadog: Datadog is another good option for medium projects, especially if you need to integrate with a variety of other tools and services. It offers a wide range of monitoring capabilities and is scalable.
-
Large Projects:
- Prometheus and Grafana: For large-scale projects, the combination of Prometheus and Grafana is highly recommended. Prometheus is extremely scalable and can handle large volumes of metrics, while Grafana provides powerful visualization and alerting capabilities.
- Datadog: Datadog is also well-suited for large projects due to its scalability and comprehensive feature set. It can handle the complexity and volume of data generated by large applications.
In summary, the choice of monitoring tool should be based on the specific needs and scale of your Python project. Small projects might benefit from simpler tools like Sentry or Elastic APM, while medium and large projects may require more robust solutions like New Relic, Datadog, or the Prometheus-Grafana combination.
The above is the detailed content of What are some common monitoring tools for Python applications?. For more information, please follow other related articles on the PHP Chinese website!
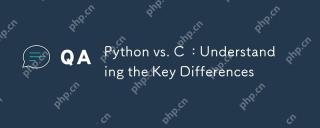
Python and C each have their own advantages, and the choice should be based on project requirements. 1) Python is suitable for rapid development and data processing due to its concise syntax and dynamic typing. 2)C is suitable for high performance and system programming due to its static typing and manual memory management.
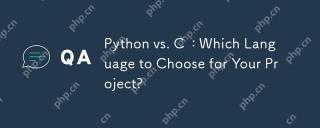
Choosing Python or C depends on project requirements: 1) If you need rapid development, data processing and prototype design, choose Python; 2) If you need high performance, low latency and close hardware control, choose C.
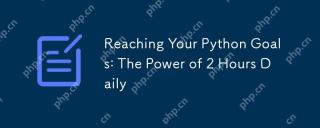
By investing 2 hours of Python learning every day, you can effectively improve your programming skills. 1. Learn new knowledge: read documents or watch tutorials. 2. Practice: Write code and complete exercises. 3. Review: Consolidate the content you have learned. 4. Project practice: Apply what you have learned in actual projects. Such a structured learning plan can help you systematically master Python and achieve career goals.
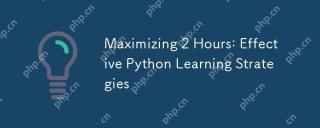
Methods to learn Python efficiently within two hours include: 1. Review the basic knowledge and ensure that you are familiar with Python installation and basic syntax; 2. Understand the core concepts of Python, such as variables, lists, functions, etc.; 3. Master basic and advanced usage by using examples; 4. Learn common errors and debugging techniques; 5. Apply performance optimization and best practices, such as using list comprehensions and following the PEP8 style guide.
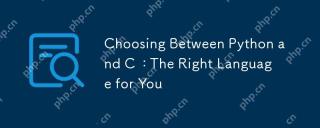
Python is suitable for beginners and data science, and C is suitable for system programming and game development. 1. Python is simple and easy to use, suitable for data science and web development. 2.C provides high performance and control, suitable for game development and system programming. The choice should be based on project needs and personal interests.
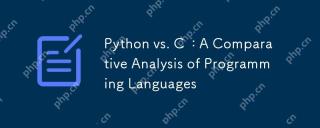
Python is more suitable for data science and rapid development, while C is more suitable for high performance and system programming. 1. Python syntax is concise and easy to learn, suitable for data processing and scientific computing. 2.C has complex syntax but excellent performance and is often used in game development and system programming.
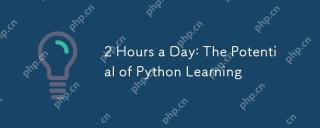
It is feasible to invest two hours a day to learn Python. 1. Learn new knowledge: Learn new concepts in one hour, such as lists and dictionaries. 2. Practice and exercises: Use one hour to perform programming exercises, such as writing small programs. Through reasonable planning and perseverance, you can master the core concepts of Python in a short time.
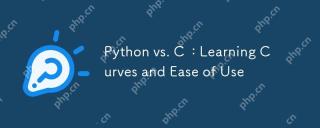
Python is easier to learn and use, while C is more powerful but complex. 1. Python syntax is concise and suitable for beginners. Dynamic typing and automatic memory management make it easy to use, but may cause runtime errors. 2.C provides low-level control and advanced features, suitable for high-performance applications, but has a high learning threshold and requires manual memory and type safety management.


Hot AI Tools

Undresser.AI Undress
AI-powered app for creating realistic nude photos

AI Clothes Remover
Online AI tool for removing clothes from photos.

Undress AI Tool
Undress images for free

Clothoff.io
AI clothes remover

Video Face Swap
Swap faces in any video effortlessly with our completely free AI face swap tool!

Hot Article

Hot Tools

MantisBT
Mantis is an easy-to-deploy web-based defect tracking tool designed to aid in product defect tracking. It requires PHP, MySQL and a web server. Check out our demo and hosting services.

PhpStorm Mac version
The latest (2018.2.1) professional PHP integrated development tool

MinGW - Minimalist GNU for Windows
This project is in the process of being migrated to osdn.net/projects/mingw, you can continue to follow us there. MinGW: A native Windows port of the GNU Compiler Collection (GCC), freely distributable import libraries and header files for building native Windows applications; includes extensions to the MSVC runtime to support C99 functionality. All MinGW software can run on 64-bit Windows platforms.

mPDF
mPDF is a PHP library that can generate PDF files from UTF-8 encoded HTML. The original author, Ian Back, wrote mPDF to output PDF files "on the fly" from his website and handle different languages. It is slower than original scripts like HTML2FPDF and produces larger files when using Unicode fonts, but supports CSS styles etc. and has a lot of enhancements. Supports almost all languages, including RTL (Arabic and Hebrew) and CJK (Chinese, Japanese and Korean). Supports nested block-level elements (such as P, DIV),
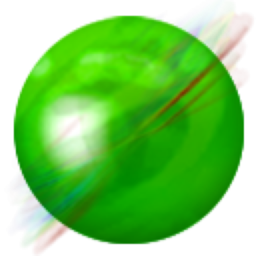
ZendStudio 13.5.1 Mac
Powerful PHP integrated development environment