


What are race conditions and deadlocks? How can you prevent them in Python?
Race Conditions:
A race condition occurs in a multi-threaded or multi-process environment when the output depends on the sequence or timing of uncontrollable events. This happens when two or more threads can access shared data and they try to change it at the same time. Because the thread scheduling algorithm can swap between threads at any time, you don't know which thread will manipulate the data first. The result can be inconsistent or unexpected behavior.
Deadlocks:
A deadlock is a situation in which two or more threads are unable to proceed because each is waiting for the other to release a resource. This results in a circular wait condition, where no thread can progress. Deadlocks can lead to freezing or significant performance degradation of applications.
Preventing Race Conditions and Deadlocks in Python:
-
Use of Locks (Mutexes):
Locks can prevent race conditions by ensuring that only one thread can access a shared resource at a time. Python'sthreading.Lock
can be used to synchronize threads. For example:import threading shared_resource = 0 lock = threading.Lock() def modify_shared_resource(): global shared_resource with lock: shared_resource = 1 threads = [threading.Thread(target=modify_shared_resource) for _ in range(10)] for thread in threads: thread.start() for thread in threads: thread.join() print(shared_resource) # Should print 10
-
Avoid Circular Waits:
To prevent deadlocks, you can ensure that resources are always acquired in the same order. This eliminates the possibility of circular waits. If thread A acquires resource 1 and then resource 2, and thread B tries to acquire resource 2 and then resource 1, a deadlock can occur. By standardizing the order, this is prevented. -
Use of Semaphores:
Semaphores are a more flexible synchronization method that can allow a limited number of threads to access a resource. They are useful in scenarios where you want to limit the number of concurrent accesses to a shared resource. -
Use of Conditions:
Thethreading.Condition
class can be used to coordinate the actions of threads and prevent race conditions. Threads can wait on a condition until a certain state is reached. -
Avoiding Shared State:
Whenever possible, minimize the use of shared state. Immutable objects and local variables can reduce the risk of race conditions.
What specific techniques or tools can be used in Python to detect race conditions and deadlocks?
-
Threading Analyzers:
Tools likeThreadSanitizer
, which is part of the Clang compiler, can be used in Python via C extensions to detect race conditions. While it's not directly available for pure Python code, it can be useful when dealing with C extensions. -
Debugging with
pdb
:
Python's built-in debugger,pdb
, can be used to inspect the state of threads during execution. By setting breakpoints and examining the state of variables, you can detect potential race conditions. -
Logging and Profiling:
Usinglogging
to trace the execution path of your threads can help identify race conditions. Profiling tools likecProfile
can help detect performance bottlenecks that might be caused by deadlocks. -
Third-Party Tools:
- Py-Spy: A sampling profiler for Python programs. It can show the call stacks of all running threads, which can help detect deadlocks.
- Memory Profiler: Helps in understanding memory usage, which can indirectly help detect performance issues due to race conditions or deadlocks.
-
Concurrency Testing:
Tools likepytest
can be used with plugins such aspytest-xdist
to run tests in parallel, which can help expose race conditions.
How do race conditions and deadlocks impact the performance and reliability of Python applications?
Impact of Race Conditions:
-
Unpredictable Behavior:
Race conditions can lead to inconsistent results, making the application's behavior unpredictable and unreliable. -
Data Corruption:
When multiple threads modify shared data simultaneously, it can result in data corruption, leading to incorrect program states. -
Performance Degradation:
The need to retry operations or the overhead of synchronization mechanisms can lead to performance degradation.
Impact of Deadlocks:
-
Freezing:
Deadlocks can cause applications to freeze, stopping all progress until the deadlock is resolved or the application is terminated. -
Resource Starvation:
Threads waiting indefinitely for locked resources can lead to resource starvation, where other threads that need those resources are blocked. -
Performance Impact:
Deadlocks can severely impact the performance of an application, as threads are unable to proceed, leading to idle CPU time and wasted resources.
Overall Impact on Reliability:
Both race conditions and deadlocks compromise the reliability of Python applications. They introduce uncertainty and can cause the application to fail in ways that are difficult to reproduce and diagnose, leading to a poor user experience and increased maintenance costs.
What are the best practices for handling race conditions and deadlocks in multi-threaded Python programs?
-
Minimize Shared State:
Reduce the use of shared state by using thread-local storage or by passing data between threads using queues or other thread-safe data structures. -
Use Synchronization Primitives:
Utilize locks, semaphores, and condition variables from thethreading
module to ensure that access to shared resources is properly synchronized. -
Implement a Consistent Resource Acquisition Order:
To avoid deadlocks, always acquire resources in a consistent order across all threads. -
Timeouts on Locks:
Use timeouts when acquiring locks to prevent indefinite waiting, which can help detect and recover from potential deadlocks. -
Testing and Debugging:
Thoroughly test your multi-threaded code under various load conditions to identify race conditions and deadlocks. Use debugging tools to inspect thread states during execution. -
Use Higher-Level Abstractions:
Consider using higher-level concurrency abstractions likeasyncio
for I/O-bound tasks, which can simplify managing concurrent operations and reduce the risk of race conditions and deadlocks. -
Code Review and Static Analysis:
Regularly review your code for potential concurrency issues, and use static analysis tools to help identify potential problems before they occur in production. -
Education and Training:
Ensure that developers are educated about the principles of concurrent programming and the specific risks and challenges associated with multi-threading in Python.
By following these best practices, you can significantly reduce the risk of race conditions and deadlocks in your Python applications, leading to more reliable and performant software.
The above is the detailed content of What are race conditions and deadlocks? How can you prevent them in Python?. For more information, please follow other related articles on the PHP Chinese website!
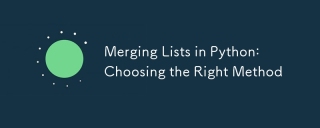
TomergelistsinPython,youcanusethe operator,extendmethod,listcomprehension,oritertools.chain,eachwithspecificadvantages:1)The operatorissimplebutlessefficientforlargelists;2)extendismemory-efficientbutmodifiestheoriginallist;3)listcomprehensionoffersf
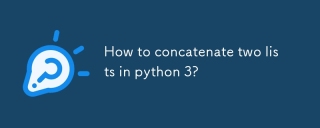
In Python 3, two lists can be connected through a variety of methods: 1) Use operator, which is suitable for small lists, but is inefficient for large lists; 2) Use extend method, which is suitable for large lists, with high memory efficiency, but will modify the original list; 3) Use * operator, which is suitable for merging multiple lists, without modifying the original list; 4) Use itertools.chain, which is suitable for large data sets, with high memory efficiency.
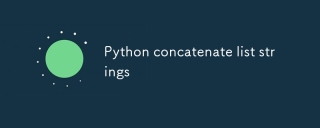
Using the join() method is the most efficient way to connect strings from lists in Python. 1) Use the join() method to be efficient and easy to read. 2) The cycle uses operators inefficiently for large lists. 3) The combination of list comprehension and join() is suitable for scenarios that require conversion. 4) The reduce() method is suitable for other types of reductions, but is inefficient for string concatenation. The complete sentence ends.
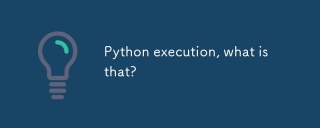
PythonexecutionistheprocessoftransformingPythoncodeintoexecutableinstructions.1)Theinterpreterreadsthecode,convertingitintobytecode,whichthePythonVirtualMachine(PVM)executes.2)TheGlobalInterpreterLock(GIL)managesthreadexecution,potentiallylimitingmul
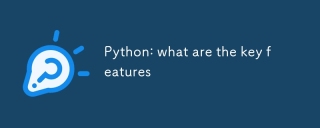
Key features of Python include: 1. The syntax is concise and easy to understand, suitable for beginners; 2. Dynamic type system, improving development speed; 3. Rich standard library, supporting multiple tasks; 4. Strong community and ecosystem, providing extensive support; 5. Interpretation, suitable for scripting and rapid prototyping; 6. Multi-paradigm support, suitable for various programming styles.
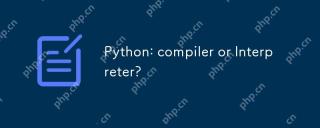
Python is an interpreted language, but it also includes the compilation process. 1) Python code is first compiled into bytecode. 2) Bytecode is interpreted and executed by Python virtual machine. 3) This hybrid mechanism makes Python both flexible and efficient, but not as fast as a fully compiled language.
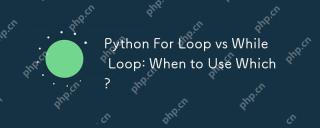
Useaforloopwheniteratingoverasequenceorforaspecificnumberoftimes;useawhileloopwhencontinuinguntilaconditionismet.Forloopsareidealforknownsequences,whilewhileloopssuitsituationswithundeterminediterations.
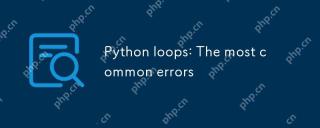
Pythonloopscanleadtoerrorslikeinfiniteloops,modifyinglistsduringiteration,off-by-oneerrors,zero-indexingissues,andnestedloopinefficiencies.Toavoidthese:1)Use'i


Hot AI Tools

Undresser.AI Undress
AI-powered app for creating realistic nude photos

AI Clothes Remover
Online AI tool for removing clothes from photos.

Undress AI Tool
Undress images for free

Clothoff.io
AI clothes remover

Video Face Swap
Swap faces in any video effortlessly with our completely free AI face swap tool!

Hot Article

Hot Tools

SublimeText3 English version
Recommended: Win version, supports code prompts!

PhpStorm Mac version
The latest (2018.2.1) professional PHP integrated development tool

SAP NetWeaver Server Adapter for Eclipse
Integrate Eclipse with SAP NetWeaver application server.

Safe Exam Browser
Safe Exam Browser is a secure browser environment for taking online exams securely. This software turns any computer into a secure workstation. It controls access to any utility and prevents students from using unauthorized resources.
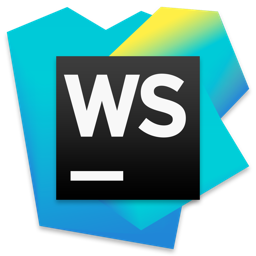
WebStorm Mac version
Useful JavaScript development tools
