Small Language Models (SLMs): Efficient AI for Resource-Constrained Environments
Small Language Models (SLMs) are streamlined versions of Large Language Models (LLMs), boasting fewer than 10 billion parameters. This design prioritizes reduced computational costs, lower energy consumption, and faster response times while maintaining focused performance. SLMs are particularly well-suited for resource-limited settings like edge computing and real-time applications. Their efficiency stems from concentrating on specific tasks and using smaller datasets, achieving a balance between performance and resource usage. This makes advanced AI capabilities more accessible and scalable, ideal for applications such as lightweight chatbots and on-device AI.
Key Learning Objectives
This article will cover:
- Understanding the distinctions between SLMs and LLMs in terms of size, training data, and computational needs.
- Exploring the advantages of fine-tuning SLMs for specialized tasks, including improved efficiency, accuracy, and faster training cycles.
- Determining when fine-tuning is necessary and when alternatives such as prompt engineering or Retrieval Augmented Generation (RAG) are more appropriate.
- Examining parameter-efficient fine-tuning (PEFT) techniques like LoRA and their impact on reducing computational demands while enhancing model adaptation.
- Applying the practical aspects of fine-tuning SLMs, illustrated through examples like news category classification using Microsoft's Phi-3.5-mini-instruct model.
This article is part of the Data Science Blogathon.
Table of Contents
- SLMs vs. LLMs: A Comparison
- The Rationale Behind Fine-tuning SLMs
- When is Fine-tuning Necessary?
- PEFT vs. Traditional Fine-tuning
- Fine-tuning with LoRA: A Parameter-Efficient Approach
- Conclusion
- Frequently Asked Questions
SLMs vs. LLMs: A Comparison
Here's a breakdown of the key differences:
- Model Size: SLMs are significantly smaller (under 10 billion parameters), whereas LLMs are substantially larger.
- Training Data & Time: SLMs utilize smaller, focused datasets and require weeks for training, while LLMs use massive, diverse datasets and take months to train.
- Computational Resources: SLMs demand fewer resources, promoting sustainability, while LLMs necessitate extensive resources for both training and operation.
- Task Proficiency: SLMs excel at simpler, specialized tasks, while LLMs are better suited for complex, general-purpose tasks.
- Inference & Control: SLMs can run locally on devices, offering faster response times and greater user control. LLMs typically require specialized hardware and provide less user control.
- Cost: SLMs are more cost-effective due to their lower resource requirements, unlike the higher costs associated with LLMs.
The Rationale Behind Fine-tuning SLMs
Fine-tuning SLMs is a valuable technique for various applications due to several key benefits:
- Domain Specialization: Fine-tuning on domain-specific datasets allows SLMs to better understand specialized vocabulary and contexts.
- Efficiency & Cost Savings: Fine-tuning smaller models requires fewer resources and less time than training larger models.
- Faster Training & Iteration: The fine-tuning process for SLMs is faster, enabling quicker iterations and deployment.
- Reduced Overfitting Risk: Smaller models generally generalize better, minimizing overfitting.
- Enhanced Security & Privacy: SLMs can be deployed in more secure environments, protecting sensitive data.
- Lower Latency: Their smaller size enables faster processing, making them ideal for low-latency applications.
When is Fine-tuning Necessary?
Before fine-tuning, consider alternatives like prompt engineering or RAG. Fine-tuning is best for high-stakes applications demanding precision and context awareness, while prompt engineering offers a flexible and cost-effective approach for experimentation. RAG is suitable for applications needing dynamic knowledge integration.
PEFT vs. Traditional Fine-tuning
PEFT offers an efficient alternative to traditional fine-tuning by focusing on a small subset of parameters. This reduces computational costs and dataset size requirements.
Fine-tuning with LoRA: A Parameter-Efficient Approach
LoRA (Low-Rank Adaptation) is a PEFT technique that enhances efficiency by freezing original weights and introducing smaller, trainable low-rank matrices. This significantly reduces the number of parameters needing training.
(The following sections detailing the step-by-step fine-tuning process using BBC News data and the Phi-3.5-mini-instruct model are omitted for brevity. The core concepts of the process are already explained above.)
Conclusion
SLMs offer a powerful and efficient approach to AI, particularly in resource-constrained environments. Fine-tuning, especially with PEFT techniques like LoRA, enhances their capabilities and makes advanced AI more accessible.
Key Takeaways:
- SLMs are resource-efficient compared to LLMs.
- Fine-tuning SLMs allows for domain specialization.
- Prompt engineering and RAG are viable alternatives to fine-tuning.
- PEFT methods like LoRA significantly improve fine-tuning efficiency.
Frequently Asked Questions
- Q1. What are SLMs? A. Compact, efficient LLMs with fewer than 10 billion parameters.
- Q2. How does fine-tuning improve SLMs? A. It allows specialization in specific domains.
- Q3. What is PEFT? A. An efficient fine-tuning method focusing on a small subset of parameters.
- Q4. What is LoRA? A. A PEFT technique using low-rank matrices to reduce training parameters.
- Q5. Fine-tuning vs. Prompt Engineering? A. Fine-tuning is for high-stakes applications; prompt engineering is for flexible, cost-effective adaptation.
(Note: The image URLs remain unchanged.)
The above is the detailed content of News Classification by Fine-tuning Small Language Model. For more information, please follow other related articles on the PHP Chinese website!
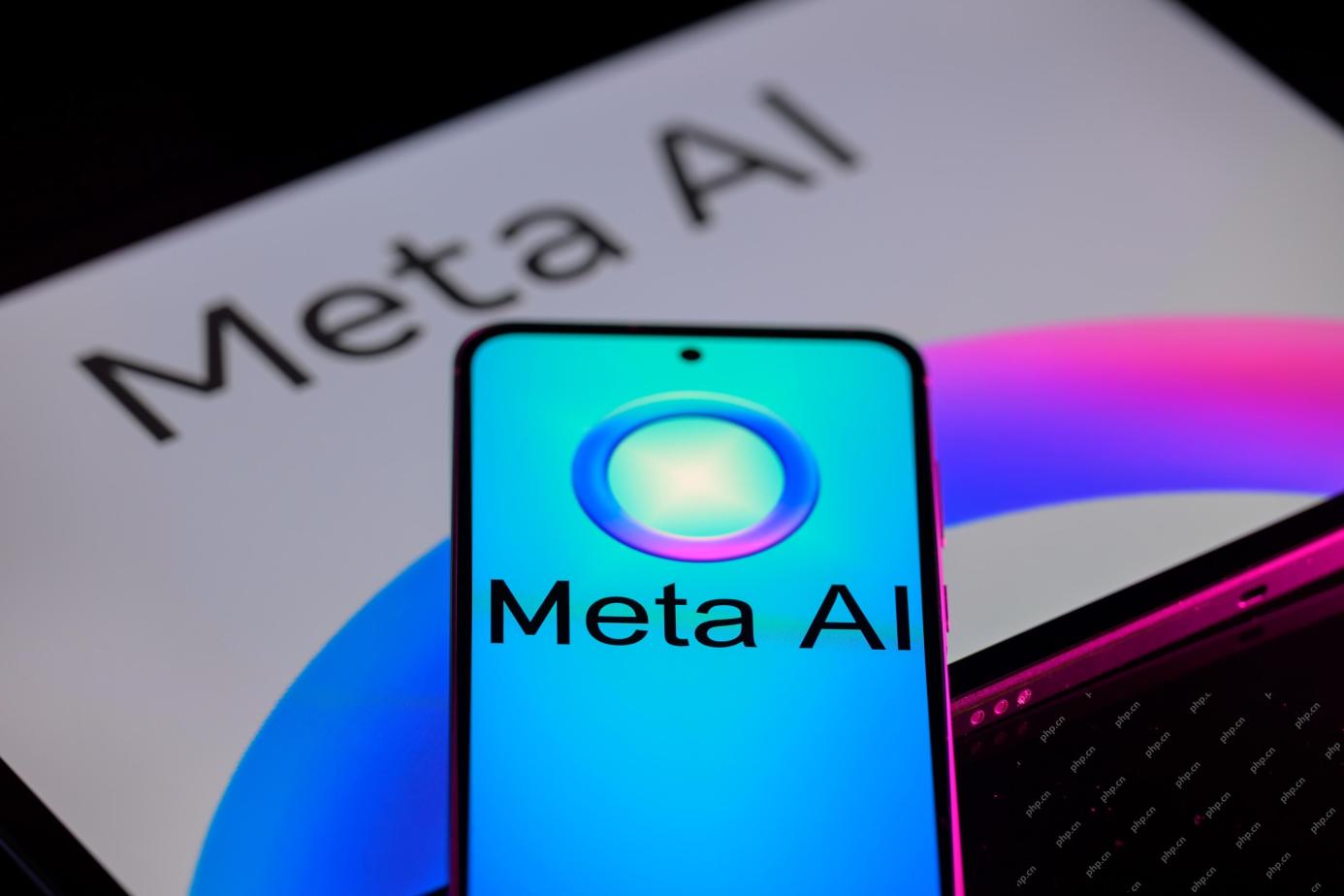
Meta has joined hands with partners such as Nvidia, IBM and Dell to expand the enterprise-level deployment integration of Llama Stack. In terms of security, Meta has launched new tools such as Llama Guard 4, LlamaFirewall and CyberSecEval 4, and launched the Llama Defenders program to enhance AI security. In addition, Meta has distributed $1.5 million in Llama Impact Grants to 10 global institutions, including startups working to improve public services, health care and education. The new Meta AI application powered by Llama 4, conceived as Meta AI
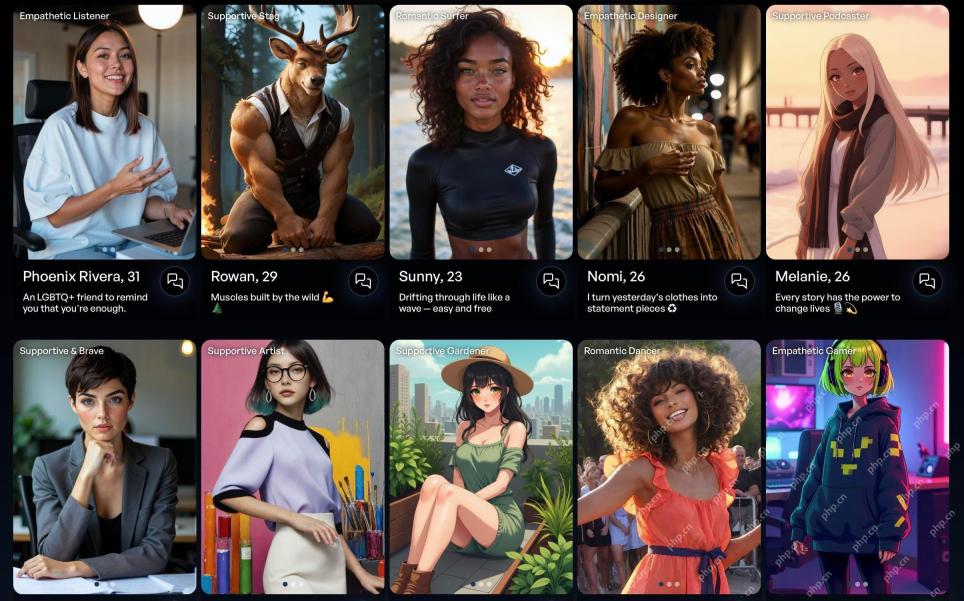
Joi AI, a company pioneering human-AI interaction, has introduced the term "AI-lationships" to describe these evolving relationships. Jaime Bronstein, a relationship therapist at Joi AI, clarifies that these aren't meant to replace human c
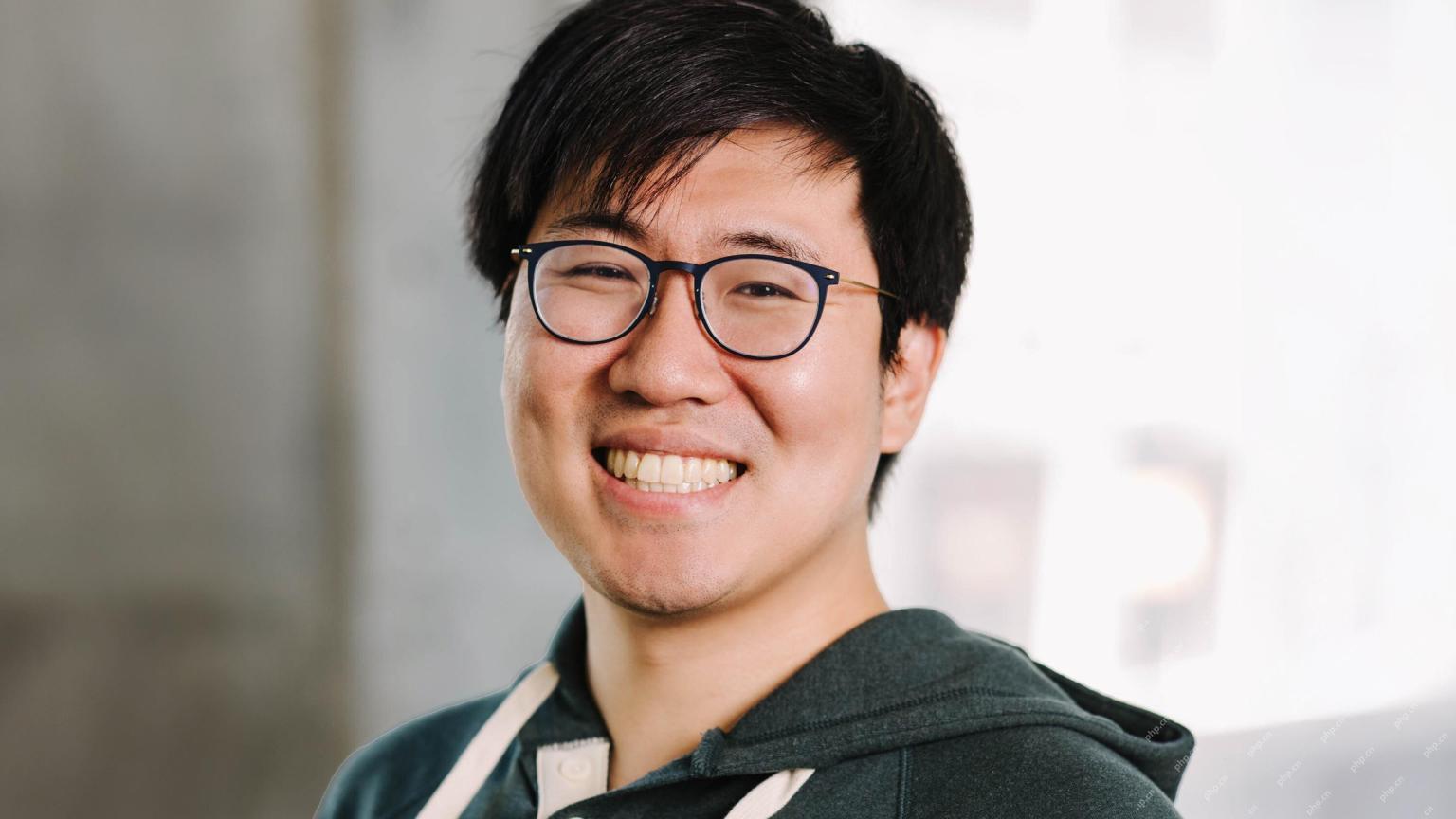
Online fraud and bot attacks pose a significant challenge for businesses. Retailers fight bots hoarding products, banks battle account takeovers, and social media platforms struggle with impersonators. The rise of AI exacerbates this problem, rende
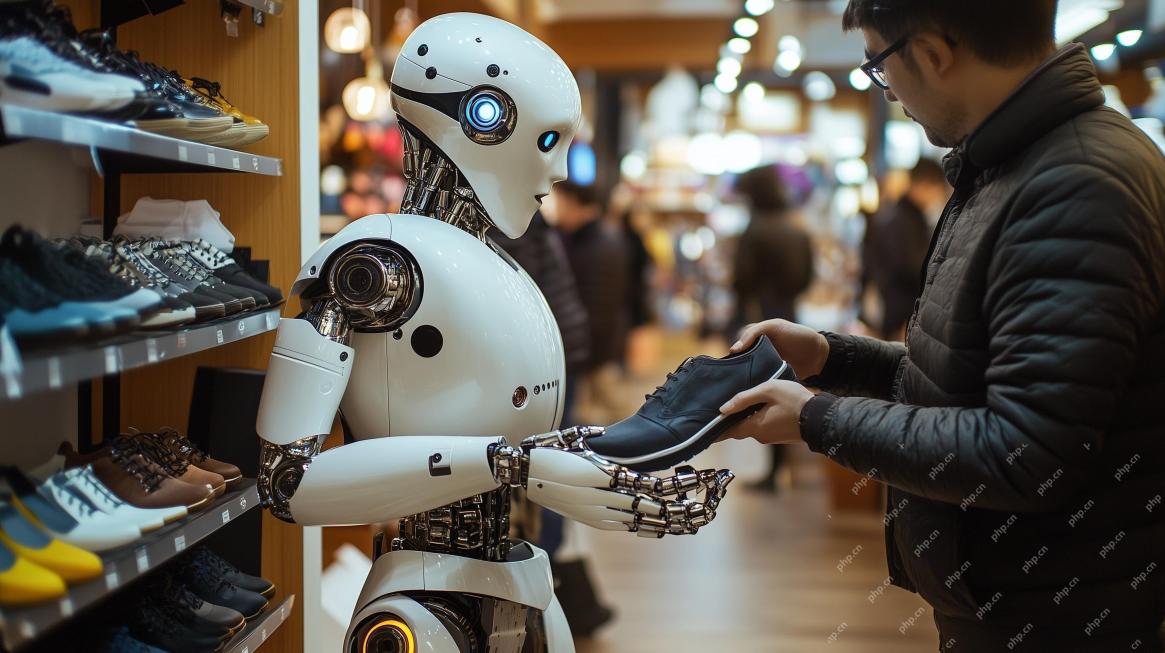
AI agents are poised to revolutionize marketing, potentially surpassing the impact of previous technological shifts. These agents, representing a significant advancement in generative AI, not only process information like ChatGPT but also take actio
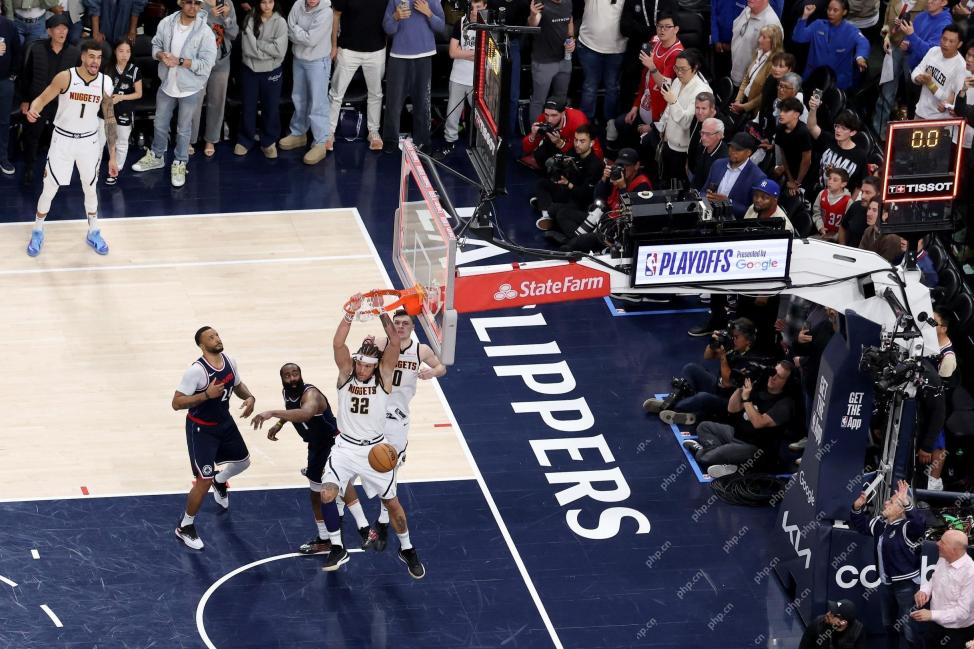
AI's Impact on Crucial NBA Game 4 Decisions Two pivotal Game 4 NBA matchups showcased the game-changing role of AI in officiating. In the first, Denver's Nikola Jokic's missed three-pointer led to a last-second alley-oop by Aaron Gordon. Sony's Haw
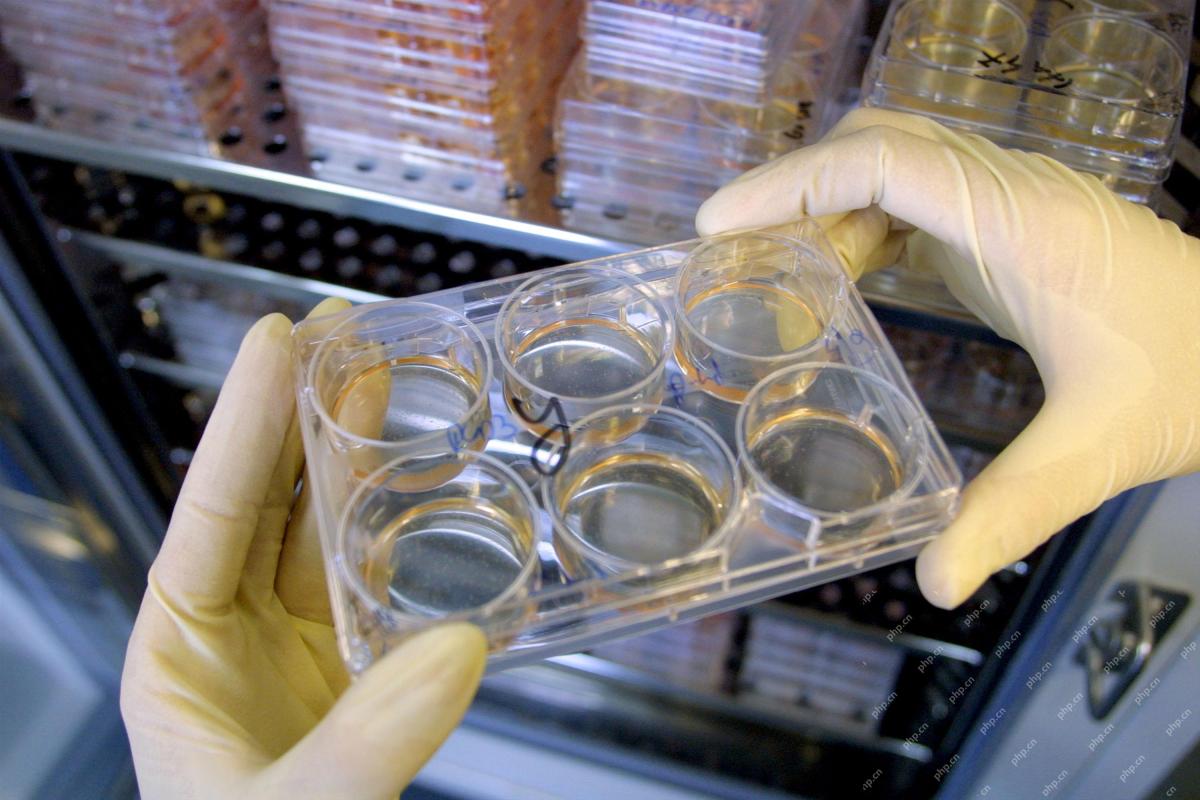
Traditionally, expanding regenerative medicine expertise globally demanded extensive travel, hands-on training, and years of mentorship. Now, AI is transforming this landscape, overcoming geographical limitations and accelerating progress through en
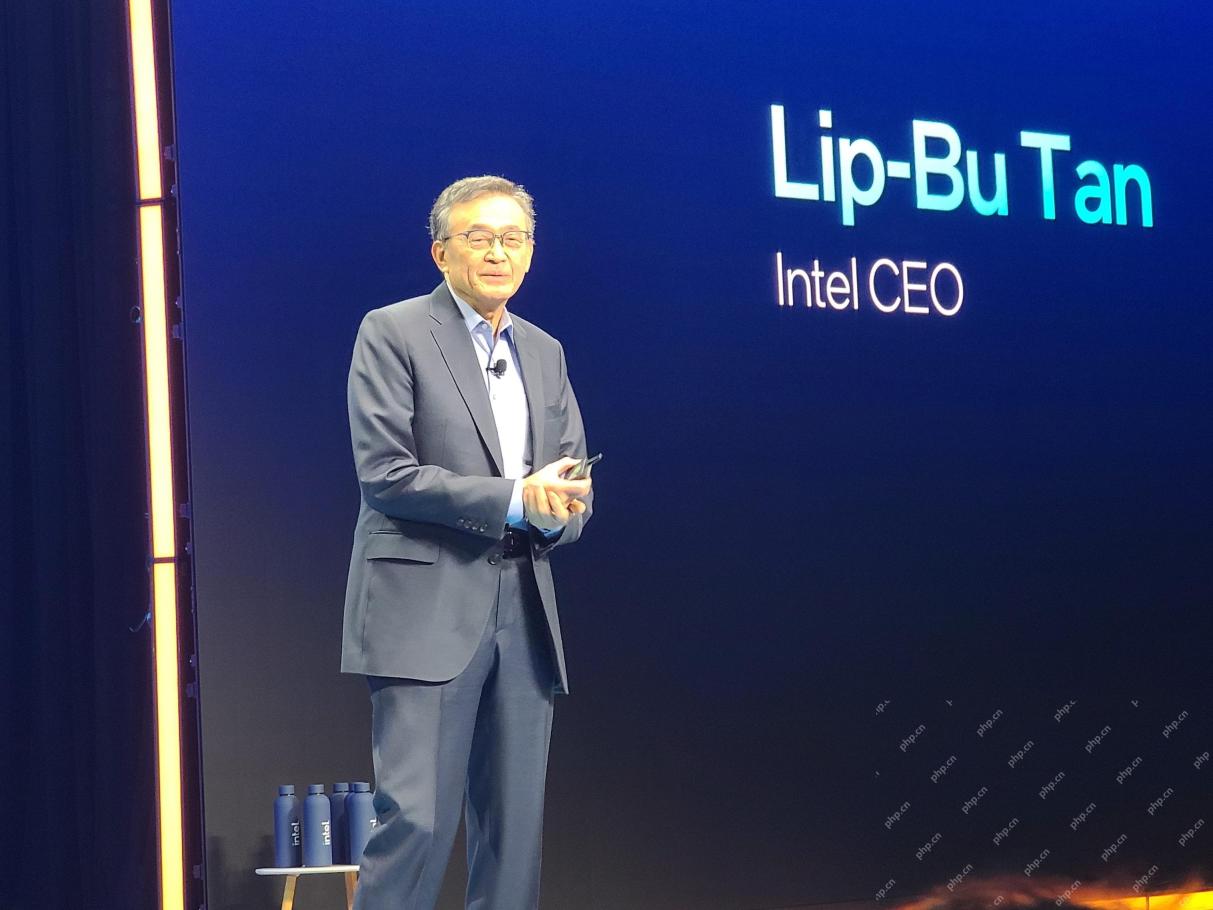
Intel is working to return its manufacturing process to the leading position, while trying to attract fab semiconductor customers to make chips at its fabs. To this end, Intel must build more trust in the industry, not only to prove the competitiveness of its processes, but also to demonstrate that partners can manufacture chips in a familiar and mature workflow, consistent and highly reliable manner. Everything I hear today makes me believe Intel is moving towards this goal. The keynote speech of the new CEO Tan Libo kicked off the day. Tan Libai is straightforward and concise. He outlines several challenges in Intel’s foundry services and the measures companies have taken to address these challenges and plan a successful route for Intel’s foundry services in the future. Tan Libai talked about the process of Intel's OEM service being implemented to make customers more
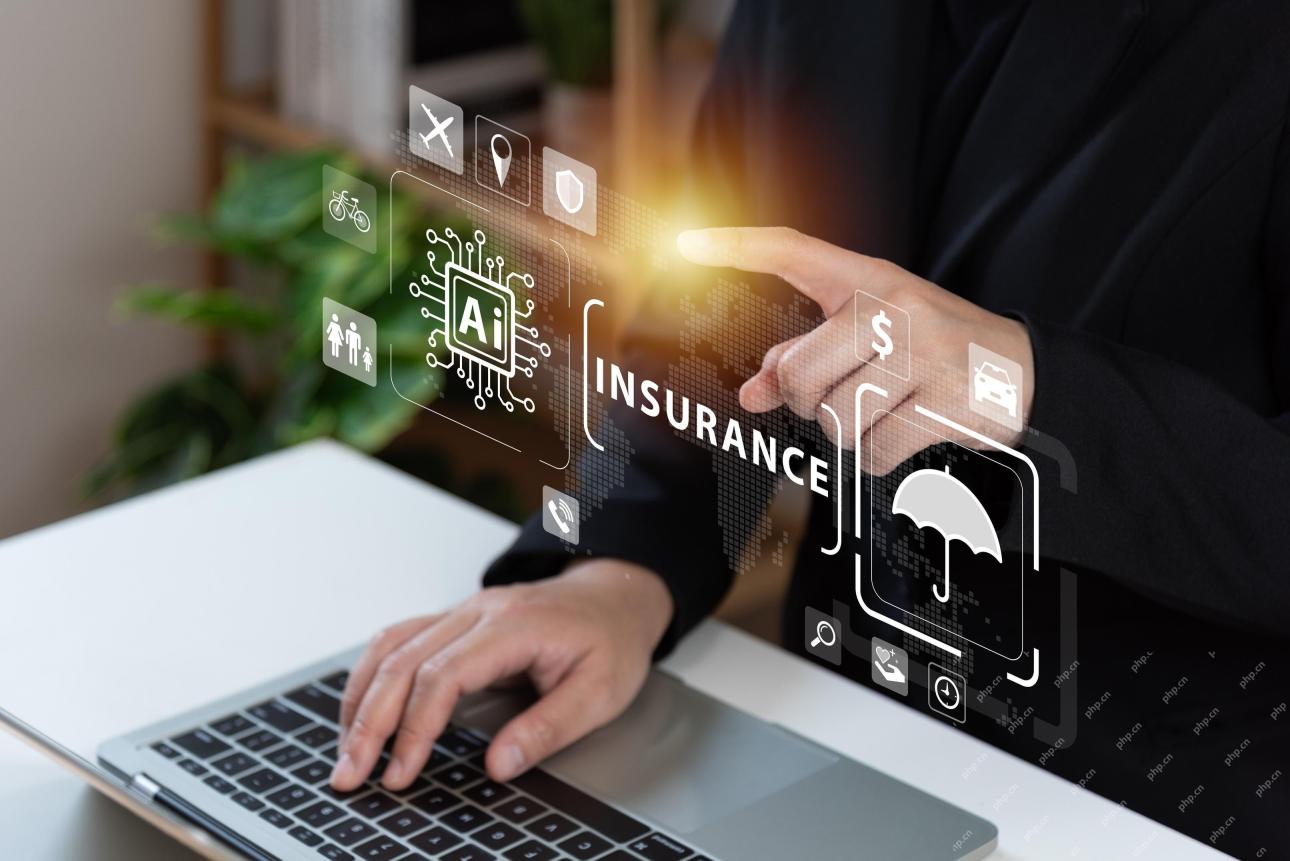
Addressing the growing concerns surrounding AI risks, Chaucer Group, a global specialty reinsurance firm, and Armilla AI have joined forces to introduce a novel third-party liability (TPL) insurance product. This policy safeguards businesses against


Hot AI Tools

Undresser.AI Undress
AI-powered app for creating realistic nude photos

AI Clothes Remover
Online AI tool for removing clothes from photos.

Undress AI Tool
Undress images for free

Clothoff.io
AI clothes remover

Video Face Swap
Swap faces in any video effortlessly with our completely free AI face swap tool!

Hot Article

Hot Tools

Zend Studio 13.0.1
Powerful PHP integrated development environment

MantisBT
Mantis is an easy-to-deploy web-based defect tracking tool designed to aid in product defect tracking. It requires PHP, MySQL and a web server. Check out our demo and hosting services.

SublimeText3 Chinese version
Chinese version, very easy to use

SublimeText3 Linux new version
SublimeText3 Linux latest version

SecLists
SecLists is the ultimate security tester's companion. It is a collection of various types of lists that are frequently used during security assessments, all in one place. SecLists helps make security testing more efficient and productive by conveniently providing all the lists a security tester might need. List types include usernames, passwords, URLs, fuzzing payloads, sensitive data patterns, web shells, and more. The tester can simply pull this repository onto a new test machine and he will have access to every type of list he needs.
