


How do I use TTL (Time-To-Live) indexes in MongoDB to automatically remove expired data?
How do I use TTL (Time-To-Live) indexes in MongoDB to automatically remove expired data?
To use TTL (Time-To-Live) indexes in MongoDB to automatically remove expired data, you need to follow these steps:
-
Identify the Field for Expiration: First, identify the field in your document that indicates when the document should expire. This field must be of type
Date
. -
Create a TTL Index: Use the
createIndex
method to create a TTL index on the expiration field. Here is an example command in the MongoDB shell:db.collection.createIndex( { "createdAt": 1 }, { expireAfterSeconds: 3600 } )
In this example,
createdAt
is the field used for expiration, andexpireAfterSeconds
is set to 3600 seconds (1 hour). Any document with acreatedAt
date older than the current time minus 3600 seconds will be automatically removed. -
Ensure the Field is Indexed Correctly: Make sure the field you choose is suitable for TTL indexing. The field should be of type
Date
, and you should consider whether it’s appropriate for your application to delete documents based on this field. -
Test and Monitor: After setting up the TTL index, monitor the collection to ensure documents are being removed as expected. You can use commands like
db.collection.stats()
to check the current state of the collection. -
Adjust as Needed: Based on monitoring and application needs, you might need to adjust the
expireAfterSeconds
value to ensure documents are deleted at the appropriate time.
What are the best practices for setting TTL values in MongoDB to ensure optimal performance?
Setting the right TTL values in MongoDB is crucial for maintaining performance and efficient data management. Here are some best practices to consider:
- Understand Your Data Lifecycle: Determine how long your data needs to be retained based on your business or application requirements. This will help you set appropriate TTL values.
- Start with a Conservative Estimate: If unsure, start with a longer TTL and gradually decrease it. This helps prevent accidental data loss and allows you to monitor the impact on your system.
- Avoid Frequent Deletions: Setting TTL values that result in very frequent deletions can lead to performance issues. Try to balance the need for fresh data with the overhead of document removal.
- Consider Peak Load Times: If your application has peak usage times, set TTL values so that deletions occur during off-peak hours to minimize the impact on performance.
- Monitor and Adjust: Regularly monitor the performance impact of TTL deletions using MongoDB's monitoring tools. Adjust TTL values based on the insights you gather.
- Use Efficient Indexing: Ensure that the TTL index is used efficiently. Avoid creating multiple TTL indexes on the same collection, as it can increase the workload on the MongoDB server.
- Test in a Staging Environment: Before applying TTL settings in production, test them in a staging environment to understand their impact on your specific workload and data patterns.
Can TTL indexes in MongoDB be used on collections with compound indexes, and if so, how?
Yes, TTL indexes in MongoDB can be used on collections that also have compound indexes. Here's how you can set it up:
-
Create the TTL Index: You create the TTL index as you normally would. For example:
db.collection.createIndex( { "createdAt": 1 }, { expireAfterSeconds: 3600 } )
-
Create the Compound Index: You can then create a compound index on the same collection. For instance:
db.collection.createIndex( { "status": 1, "createdAt": 1 } )
This index will be used for queries and sorting, while the TTL index will still work to remove expired documents.
- Ensure Non-Conflicting Indexes: Make sure that the TTL index and the compound index do not conflict. For example, having multiple TTL indexes on the same collection is not recommended, as it can increase the workload on the MongoDB server.
- Consider Performance Implications: Adding multiple indexes, including a TTL index, can affect performance. Monitor your system closely to ensure that the additional indexing does not cause undue overhead.
How can I monitor and troubleshoot issues related to TTL indexes in MongoDB?
Monitoring and troubleshooting TTL indexes in MongoDB involves a few key steps:
-
Monitor the Collection Statistics: Use the
db.collection.stats()
command to check the current state of your collection. Look for thettl
field, which will show the number of documents removed due to TTL:db.collection.stats()
- Check the MongoDB Logs: MongoDB logs will show when documents are deleted due to TTL. You can find these entries by searching for "TTLMonitor" in the log files.
- Use MongoDB's Monitoring Tools: Tools like MongoDB Atlas or third-party monitoring solutions can help you track the performance impact of TTL deletions. Pay attention to metrics such as operation execution times and the rate of document deletions.
-
Analyze the TTL Index: Use the
db.collection.getIndexes()
command to ensure the TTL index is properly created and to check its settings:db.collection.getIndexes()
- Set Up Alerts: Configure alerts to notify you if the rate of deletions exceeds a certain threshold or if there are issues with the TTL index.
-
Troubleshoot TTL Index Issues:
- Document Not Being Removed: If documents are not being removed as expected, verify that the TTL index is set correctly and that the date field used for TTL is in the correct format.
- Performance Impact: If you notice a performance impact, consider adjusting the TTL value to reduce the frequency of deletions, or reassess whether TTL is necessary for that collection.
- Index Overhead: If multiple TTL indexes are causing overhead, consider consolidating them or re-evaluating whether all are necessary.
By following these steps, you can effectively monitor and troubleshoot any issues related to TTL indexes in MongoDB.
The above is the detailed content of How do I use TTL (Time-To-Live) indexes in MongoDB to automatically remove expired data?. For more information, please follow other related articles on the PHP Chinese website!
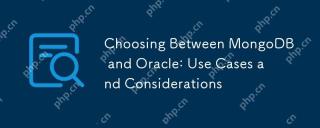
MongoDB is suitable for processing large-scale, unstructured data, and Oracle is suitable for scenarios that require strict data consistency and complex queries. 1.MongoDB provides flexibility and scalability, suitable for variable data structures. 2. Oracle provides strong transaction support and data consistency, suitable for enterprise-level applications. Data structure, scalability and performance requirements need to be considered when choosing.
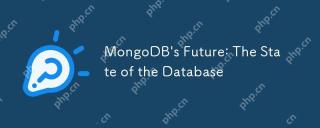
MongoDB's future is full of possibilities: 1. The development of cloud-native databases, 2. The fields of artificial intelligence and big data are focused, 3. The improvement of security and compliance. MongoDB continues to advance and make breakthroughs in technological innovation, market position and future development direction.
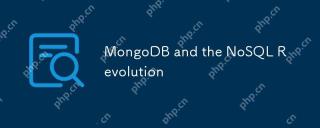
MongoDB is a document-based NoSQL database designed to provide high-performance, scalable and flexible data storage solutions. 1) It uses BSON format to store data, which is suitable for processing semi-structured or unstructured data. 2) Realize horizontal expansion through sharding technology and support complex queries and data processing. 3) Pay attention to index optimization, data modeling and performance monitoring when using it to give full play to its advantages.
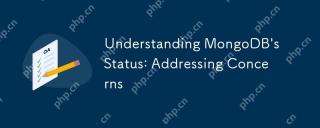
MongoDB is suitable for project needs, but it needs to be used optimized. 1) Performance: Optimize indexing strategies and use sharding technology. 2) Security: Enable authentication and data encryption. 3) Scalability: Use replica sets and sharding technologies.
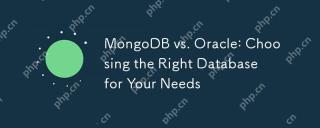
MongoDB is suitable for unstructured data and high scalability requirements, while Oracle is suitable for scenarios that require strict data consistency. 1.MongoDB flexibly stores data in different structures, suitable for social media and the Internet of Things. 2. Oracle structured data model ensures data integrity and is suitable for financial transactions. 3.MongoDB scales horizontally through shards, and Oracle scales vertically through RAC. 4.MongoDB has low maintenance costs, while Oracle has high maintenance costs but is fully supported.
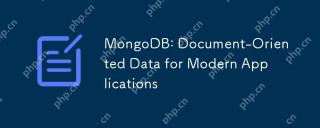
MongoDB has changed the way of development with its flexible documentation model and high-performance storage engine. Its advantages include: 1. Patternless design, allowing fast iteration; 2. The document model supports nesting and arrays, enhancing data structure flexibility; 3. The automatic sharding function supports horizontal expansion, suitable for large-scale data processing.
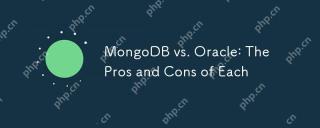
MongoDB is suitable for projects that iterate and process large-scale unstructured data quickly, while Oracle is suitable for enterprise-level applications that require high reliability and complex transaction processing. MongoDB is known for its flexible document storage and efficient read and write operations, suitable for modern web applications and big data analysis; Oracle is known for its strong data management capabilities and SQL support, and is widely used in industries such as finance and telecommunications.
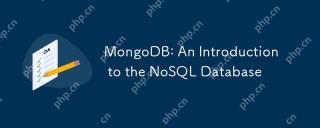
MongoDB is a document-based NoSQL database that uses BSON format to store data, suitable for processing complex and unstructured data. 1) Its document model is flexible and suitable for frequently changing data structures. 2) MongoDB uses WiredTiger storage engine and query optimizer to support efficient data operations and queries. 3) Basic operations include inserting, querying, updating and deleting documents. 4) Advanced usage includes using an aggregation framework for complex data analysis. 5) Common errors include connection problems, query performance problems, and data consistency problems. 6) Performance optimization and best practices include index optimization, data modeling, sharding, caching, monitoring and tuning.


Hot AI Tools

Undresser.AI Undress
AI-powered app for creating realistic nude photos

AI Clothes Remover
Online AI tool for removing clothes from photos.

Undress AI Tool
Undress images for free

Clothoff.io
AI clothes remover

Video Face Swap
Swap faces in any video effortlessly with our completely free AI face swap tool!

Hot Article

Hot Tools

DVWA
Damn Vulnerable Web App (DVWA) is a PHP/MySQL web application that is very vulnerable. Its main goals are to be an aid for security professionals to test their skills and tools in a legal environment, to help web developers better understand the process of securing web applications, and to help teachers/students teach/learn in a classroom environment Web application security. The goal of DVWA is to practice some of the most common web vulnerabilities through a simple and straightforward interface, with varying degrees of difficulty. Please note that this software
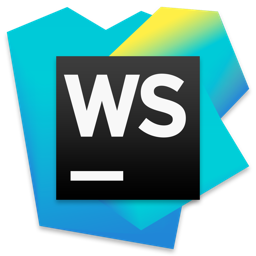
WebStorm Mac version
Useful JavaScript development tools

Atom editor mac version download
The most popular open source editor
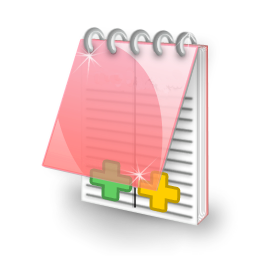
EditPlus Chinese cracked version
Small size, syntax highlighting, does not support code prompt function

MinGW - Minimalist GNU for Windows
This project is in the process of being migrated to osdn.net/projects/mingw, you can continue to follow us there. MinGW: A native Windows port of the GNU Compiler Collection (GCC), freely distributable import libraries and header files for building native Windows applications; includes extensions to the MSVC runtime to support C99 functionality. All MinGW software can run on 64-bit Windows platforms.
