This article introduces NumPy, Python's core numerical computing library. It details array creation, manipulation, operations (including broadcasting and linear algebra), and performance optimization techniques. Real-world applications in diverse f
How to Use NumPy for Numerical Computing in Python?
NumPy, short for Numerical Python, is the cornerstone of numerical computing in Python. Its core functionality revolves around the ndarray
(n-dimensional array) object, a powerful data structure that provides efficient storage and manipulation of large arrays of numerical data. Here's a breakdown of how to use NumPy effectively:
1. Installation: If you don't have it already, install NumPy using pip: pip install numpy
.
2. Importing NumPy: Begin by importing the library: import numpy as np
. The as np
convention is widely adopted for brevity.
3. Creating Arrays: NumPy offers several ways to create arrays:
-
From lists:
my_array = np.array([1, 2, 3, 4, 5])
creates a 1D array. Nested lists create multi-dimensional arrays:my_matrix = np.array([[1, 2], [3, 4]])
. -
Using functions:
np.zeros((3, 4))
creates a 3x4 array filled with zeros.np.ones((2, 2))
creates a 2x2 array of ones.np.arange(10)
creates a sequence from 0 to 9.np.linspace(0, 1, 11)
creates 11 evenly spaced points between 0 and 1.np.random.rand(3, 3)
generates a 3x3 array of random numbers between 0 and 1.
4. Array Operations: NumPy's strength lies in its ability to perform element-wise operations on arrays efficiently. For example:
-
my_array 2
adds 2 to each element. -
my_array * 3
multiplies each element by 3. -
my_array1 my_array2
adds corresponding elements of two arrays (element-wise addition). -
np.dot(my_array1, my_array2)
performs matrix multiplication (for 2D arrays).
5. Array Slicing and Indexing: Accessing array elements is intuitive: my_array[0]
gets the first element, my_matrix[1, 0]
gets the element at the second row and first column. Slicing allows extracting sub-arrays: my_array[1:4]
gets elements from index 1 to 3.
6. Broadcasting: NumPy's broadcasting rules allow operations between arrays of different shapes under certain conditions, simplifying code and improving efficiency.
7. Linear Algebra: NumPy provides functions for linear algebra operations like matrix inversion (np.linalg.inv()
), eigenvalue decomposition (np.linalg.eig()
), and solving linear equations (np.linalg.solve()
).
What are the most common NumPy functions used in scientific computing?
Many NumPy functions are crucial for scientific computing. Here are some of the most frequently used:
-
np.array()
: The fundamental function for creating arrays. -
np.arange()
andnp.linspace()
: For generating sequences of numbers. -
np.reshape()
: Changes the shape of an array without altering its data. -
np.sum()
,np.mean()
,np.std()
,np.max()
,np.min()
: For calculating statistical measures. -
np.dot()
: For matrix multiplication and dot products. -
np.transpose()
: For transposing matrices. -
np.linalg.solve()
andnp.linalg.inv()
: For solving linear equations and finding matrix inverses. -
np.fft.*
: Functions for Fast Fourier Transforms (essential in signal processing). -
np.random.*
: Functions for generating random numbers from various distributions. -
np.where()
: Conditional array creation.
How can I improve the performance of my numerical computations using NumPy?
NumPy's performance advantage stems from its use of vectorized operations and optimized C code under the hood. However, you can further enhance performance by:
- Vectorization: Avoid explicit loops whenever possible. NumPy's operations are inherently vectorized, meaning they operate on entire arrays at once, much faster than iterating through elements individually.
- Broadcasting: Leverage broadcasting to minimize the need for explicit array reshaping or looping.
-
Data Types: Choose appropriate data types for your arrays (e.g.,
np.float32
instead ofnp.float64
if precision isn't critical) to reduce memory usage and improve speed. -
Memory Management: Be mindful of memory usage, especially with large arrays. Consider using memory-mapped arrays (
np.memmap
) for very large datasets that don't fit entirely in RAM. -
Profiling: Use profiling tools (e.g.,
cProfile
) to identify performance bottlenecks in your code. - Numba or Cython: For computationally intensive parts of your code that can't be sufficiently optimized with NumPy alone, consider using Numba (just-in-time compilation) or Cython (combining Python and C) for significant speedups.
What are some examples of real-world applications where NumPy excels?
NumPy's versatility makes it invaluable across numerous scientific and engineering domains:
- Image Processing: Representing images as NumPy arrays allows for efficient manipulation, filtering, and transformation.
- Machine Learning: NumPy forms the foundation of many machine learning libraries (like scikit-learn), handling data preprocessing, feature engineering, and model training.
- Data Analysis: NumPy simplifies data manipulation, cleaning, and analysis, enabling efficient statistical calculations and data visualization.
- Financial Modeling: NumPy's capabilities are crucial for building financial models, performing risk assessments, and analyzing market data.
- Scientific Simulations: NumPy's speed and efficiency are essential for simulating physical systems, solving differential equations, and performing numerical analysis.
- Signal Processing: NumPy's FFT capabilities are vital for analyzing and manipulating signals in various applications, such as audio processing and telecommunications.
In summary, NumPy is a fundamental tool for anyone working with numerical data in Python, offering efficiency, versatility, and a rich set of functions for a wide array of applications.
The above is the detailed content of How to Use NumPy for Numerical Computing in Python?. For more information, please follow other related articles on the PHP Chinese website!
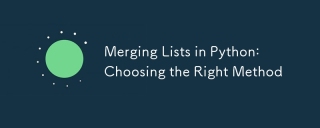
TomergelistsinPython,youcanusethe operator,extendmethod,listcomprehension,oritertools.chain,eachwithspecificadvantages:1)The operatorissimplebutlessefficientforlargelists;2)extendismemory-efficientbutmodifiestheoriginallist;3)listcomprehensionoffersf
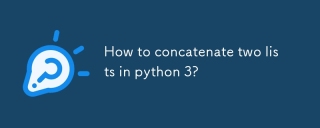
In Python 3, two lists can be connected through a variety of methods: 1) Use operator, which is suitable for small lists, but is inefficient for large lists; 2) Use extend method, which is suitable for large lists, with high memory efficiency, but will modify the original list; 3) Use * operator, which is suitable for merging multiple lists, without modifying the original list; 4) Use itertools.chain, which is suitable for large data sets, with high memory efficiency.
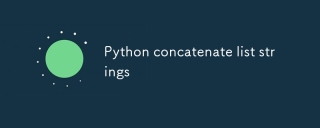
Using the join() method is the most efficient way to connect strings from lists in Python. 1) Use the join() method to be efficient and easy to read. 2) The cycle uses operators inefficiently for large lists. 3) The combination of list comprehension and join() is suitable for scenarios that require conversion. 4) The reduce() method is suitable for other types of reductions, but is inefficient for string concatenation. The complete sentence ends.
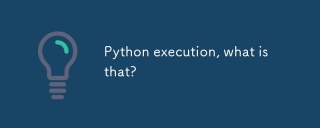
PythonexecutionistheprocessoftransformingPythoncodeintoexecutableinstructions.1)Theinterpreterreadsthecode,convertingitintobytecode,whichthePythonVirtualMachine(PVM)executes.2)TheGlobalInterpreterLock(GIL)managesthreadexecution,potentiallylimitingmul
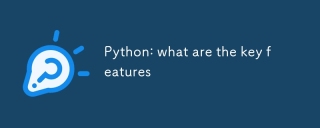
Key features of Python include: 1. The syntax is concise and easy to understand, suitable for beginners; 2. Dynamic type system, improving development speed; 3. Rich standard library, supporting multiple tasks; 4. Strong community and ecosystem, providing extensive support; 5. Interpretation, suitable for scripting and rapid prototyping; 6. Multi-paradigm support, suitable for various programming styles.
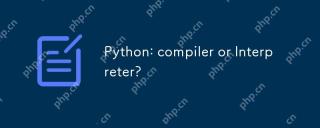
Python is an interpreted language, but it also includes the compilation process. 1) Python code is first compiled into bytecode. 2) Bytecode is interpreted and executed by Python virtual machine. 3) This hybrid mechanism makes Python both flexible and efficient, but not as fast as a fully compiled language.
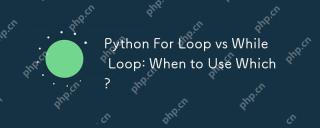
Useaforloopwheniteratingoverasequenceorforaspecificnumberoftimes;useawhileloopwhencontinuinguntilaconditionismet.Forloopsareidealforknownsequences,whilewhileloopssuitsituationswithundeterminediterations.
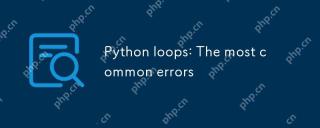
Pythonloopscanleadtoerrorslikeinfiniteloops,modifyinglistsduringiteration,off-by-oneerrors,zero-indexingissues,andnestedloopinefficiencies.Toavoidthese:1)Use'i


Hot AI Tools

Undresser.AI Undress
AI-powered app for creating realistic nude photos

AI Clothes Remover
Online AI tool for removing clothes from photos.

Undress AI Tool
Undress images for free

Clothoff.io
AI clothes remover

Video Face Swap
Swap faces in any video effortlessly with our completely free AI face swap tool!

Hot Article

Hot Tools
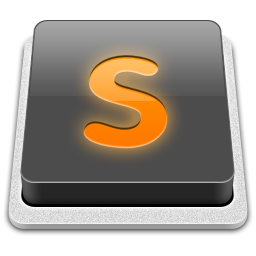
SublimeText3 Mac version
God-level code editing software (SublimeText3)

Zend Studio 13.0.1
Powerful PHP integrated development environment

Safe Exam Browser
Safe Exam Browser is a secure browser environment for taking online exams securely. This software turns any computer into a secure workstation. It controls access to any utility and prevents students from using unauthorized resources.

SublimeText3 English version
Recommended: Win version, supports code prompts!

PhpStorm Mac version
The latest (2018.2.1) professional PHP integrated development tool
