This article explains Python decorators, functions modifying other functions without altering their core. It details their mechanism using nested functions and closures, showcasing improved code readability and maintainability through reduced duplic
What are Python Decorators and How Do They Work?
Python decorators are a powerful and expressive feature that allows you to modify or enhance functions and methods in a clean and readable way. They are essentially a form of metaprogramming, allowing you to wrap additional functionality around an existing function without modifying its core behavior. At their heart, decorators are functions that take another function as input and return a modified version of that function.
This modification happens through a process involving nested functions and closures. A decorator typically uses a nested function to wrap the original function. This nested function then calls the original function, potentially adding extra functionality before, after, or even around the original function's execution. The closure ensures that the nested function retains access to the variables in its enclosing scope, even after the outer function has finished executing.
Here's a simple example:
def my_decorator(func): def wrapper(): print("Before function execution") func() print("After function execution") return wrapper @my_decorator def say_hello(): print("Hello!") say_hello()
In this example, my_decorator
is the decorator. It takes say_hello
as input and returns the wrapper
function. The @my_decorator
syntax is syntactic sugar that applies the decorator to say_hello
. When say_hello()
is called, it actually executes the wrapper
function, which prints messages before and after the original say_hello()
function's execution. The output will be:
<code>Before function execution Hello! After function execution</code>
Can decorators improve code readability and maintainability in Python?
Yes, decorators can significantly improve code readability and maintainability in Python when used appropriately. They achieve this in several ways:
- Reducing Code Duplication: Decorators allow you to encapsulate common functionality that might otherwise be repeated across multiple functions. This leads to more concise and less repetitive code.
- Improving Code Organization: By separating concerns, decorators help organize code more effectively. For example, logging, timing, or authentication logic can be neatly encapsulated in decorators, leaving the core function logic cleaner and easier to understand.
- Enhancing Reusability: Once a decorator is defined, it can be easily reused across many different functions, promoting code reuse and consistency.
- Simplifying Complex Logic: Decorators can help manage complex logic in a more structured and manageable way. Instead of embedding complex logic within each function, you can abstract it into a decorator, making the code easier to read, debug, and maintain.
However, overuse of decorators can lead to decreased readability if they become too complex or obscure the underlying function's purpose. A balance is key.
What are some practical examples of using decorators in Python projects?
Decorators find wide application in various aspects of Python programming. Here are a few practical examples:
- Logging: A decorator can log function entry and exit times, arguments, and return values, aiding in debugging and monitoring.
- Timing: A decorator can measure the execution time of a function, helping identify performance bottlenecks.
- Authentication: A decorator can check user authentication before allowing access to a function, ensuring security.
- Input Validation: A decorator can validate function input arguments, preventing unexpected errors.
- Caching: A decorator can cache the results of a function call, improving performance for computationally expensive functions.
- Rate Limiting: A decorator can limit the rate at which a function is called, preventing overload.
Are there any common pitfalls to avoid when implementing decorators in Python?
While decorators are powerful, there are potential pitfalls to avoid:
- Overuse: Overusing decorators can make code harder to understand and debug. Use them judiciously where they genuinely improve readability and maintainability.
- Debugging Challenges: Debugging decorated functions can be slightly more challenging because the actual execution flow involves the decorator's wrapper function. Using a debugger effectively is crucial.
- Complex Decorators: Avoid creating overly complex decorators. If a decorator becomes too large or intricate, it's a sign that it might need to be refactored into smaller, more manageable components.
-
Incorrect Use with Arguments: When decorators need to accept arguments, they require additional complexity using nested functions and functools.wraps. Failing to use
functools.wraps
correctly can lead to issues with function metadata (like__name__
and__doc__
). - Not understanding closures: A lack of understanding of closures can lead to unexpected behavior, particularly with the scope of variables within the decorator.
By carefully considering these potential issues and adhering to best practices, you can harness the power of decorators effectively and enhance your Python code.
The above is the detailed content of What are Python Decorators and How Do They Work?. For more information, please follow other related articles on the PHP Chinese website!
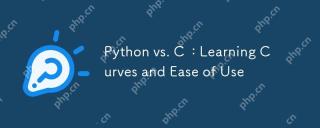
Python is easier to learn and use, while C is more powerful but complex. 1. Python syntax is concise and suitable for beginners. Dynamic typing and automatic memory management make it easy to use, but may cause runtime errors. 2.C provides low-level control and advanced features, suitable for high-performance applications, but has a high learning threshold and requires manual memory and type safety management.
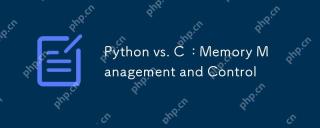
Python and C have significant differences in memory management and control. 1. Python uses automatic memory management, based on reference counting and garbage collection, simplifying the work of programmers. 2.C requires manual management of memory, providing more control but increasing complexity and error risk. Which language to choose should be based on project requirements and team technology stack.
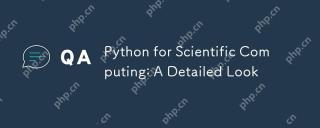
Python's applications in scientific computing include data analysis, machine learning, numerical simulation and visualization. 1.Numpy provides efficient multi-dimensional arrays and mathematical functions. 2. SciPy extends Numpy functionality and provides optimization and linear algebra tools. 3. Pandas is used for data processing and analysis. 4.Matplotlib is used to generate various graphs and visual results.
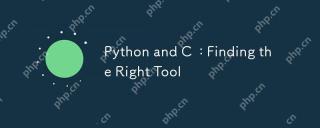
Whether to choose Python or C depends on project requirements: 1) Python is suitable for rapid development, data science, and scripting because of its concise syntax and rich libraries; 2) C is suitable for scenarios that require high performance and underlying control, such as system programming and game development, because of its compilation and manual memory management.
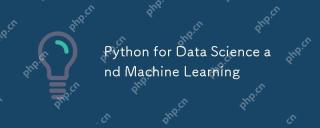
Python is widely used in data science and machine learning, mainly relying on its simplicity and a powerful library ecosystem. 1) Pandas is used for data processing and analysis, 2) Numpy provides efficient numerical calculations, and 3) Scikit-learn is used for machine learning model construction and optimization, these libraries make Python an ideal tool for data science and machine learning.
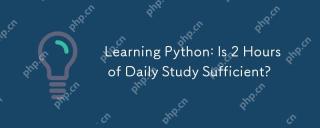
Is it enough to learn Python for two hours a day? It depends on your goals and learning methods. 1) Develop a clear learning plan, 2) Select appropriate learning resources and methods, 3) Practice and review and consolidate hands-on practice and review and consolidate, and you can gradually master the basic knowledge and advanced functions of Python during this period.
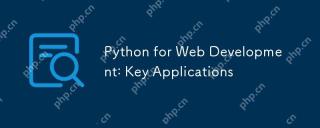
Key applications of Python in web development include the use of Django and Flask frameworks, API development, data analysis and visualization, machine learning and AI, and performance optimization. 1. Django and Flask framework: Django is suitable for rapid development of complex applications, and Flask is suitable for small or highly customized projects. 2. API development: Use Flask or DjangoRESTFramework to build RESTfulAPI. 3. Data analysis and visualization: Use Python to process data and display it through the web interface. 4. Machine Learning and AI: Python is used to build intelligent web applications. 5. Performance optimization: optimized through asynchronous programming, caching and code
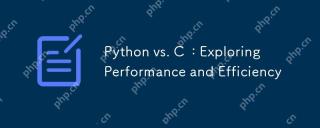
Python is better than C in development efficiency, but C is higher in execution performance. 1. Python's concise syntax and rich libraries improve development efficiency. 2.C's compilation-type characteristics and hardware control improve execution performance. When making a choice, you need to weigh the development speed and execution efficiency based on project needs.


Hot AI Tools

Undresser.AI Undress
AI-powered app for creating realistic nude photos

AI Clothes Remover
Online AI tool for removing clothes from photos.

Undress AI Tool
Undress images for free

Clothoff.io
AI clothes remover

Video Face Swap
Swap faces in any video effortlessly with our completely free AI face swap tool!

Hot Article

Hot Tools

MantisBT
Mantis is an easy-to-deploy web-based defect tracking tool designed to aid in product defect tracking. It requires PHP, MySQL and a web server. Check out our demo and hosting services.
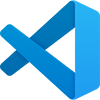
VSCode Windows 64-bit Download
A free and powerful IDE editor launched by Microsoft

SublimeText3 Linux new version
SublimeText3 Linux latest version

SAP NetWeaver Server Adapter for Eclipse
Integrate Eclipse with SAP NetWeaver application server.
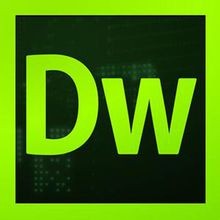
Dreamweaver CS6
Visual web development tools