Self-Reflective Retrieval-Augmented Generation (Self-RAG): Enhancing LLMs with Adaptive Retrieval and Self-Critique
Large language models (LLMs) are transformative, but their reliance on parametric knowledge often leads to factual inaccuracies. Retrieval-Augmented Generation (RAG) aims to address this by incorporating external knowledge, but traditional RAG methods suffer from limitations. This article explores Self-RAG, a novel approach that significantly improves LLM quality and factuality.
Addressing the Shortcomings of Standard RAG
Standard RAG retrieves a fixed number of passages, regardless of relevance. This leads to several issues:
- Irrelevant Information: Retrieval of unnecessary documents dilutes the output quality.
- Lack of Adaptability: Inability to adjust retrieval based on task demands results in inconsistent performance.
- Inconsistent Outputs: Generated text may not align with retrieved information due to a lack of explicit training on knowledge integration.
- Absence of Self-Evaluation: No mechanism for evaluating the quality or relevance of retrieved passages or the generated output.
- Limited Source Attribution: Insufficient citation or indication of source support for generated text.
Introducing Self-RAG: Adaptive Retrieval and Self-Reflection
Self-RAG enhances LLMs by integrating adaptive retrieval and self-reflection. Unlike standard RAG, it dynamically retrieves passages only when necessary, using a "retrieve token." Crucially, it employs special reflection tokens—ISREL (relevance), ISSUP (support), and ISUSE (utility)—to assess its own generation process.
Key features of Self-RAG include:
- On-Demand Retrieval: Efficient retrieval only when needed.
- Reflection Tokens: Self-evaluation using ISREL, ISSUP, and ISUSE tokens.
- Self-Critique: Assessment of retrieved passage relevance and output quality.
- End-to-End Training: Simultaneous training of output generation and reflection token prediction.
- Customizable Decoding: Flexible adjustment of retrieval frequency and adaptation to different tasks.
The Self-RAG Workflow
- Input Processing and Retrieval Decision: The model determines if external knowledge is required.
- Retrieval of Relevant Passages: If needed, relevant passages are retrieved using a retriever model (e.g., Contriever-MS MARCO).
- Parallel Processing and Segment Generation: The generator model processes each retrieved passage, creating multiple continuation candidates with associated critique tokens.
- Self-Critique and Evaluation: Reflection tokens evaluate the relevance (ISREL), support (ISSUP), and utility (ISUSE) of each generated segment.
- Selection of the Best Segment and Output: A segment-level beam search selects the best output sequence based on a weighted score incorporating critique token probabilities.
- Training Process: A two-stage training process involves training a critic model offline to generate reflection tokens, followed by training the generator model using data augmented with these tokens.
Advantages of Self-RAG
Self-RAG offers several key advantages:
- Improved Factual Accuracy: On-demand retrieval and self-critique lead to higher factual accuracy.
- Enhanced Relevance: Adaptive retrieval ensures only relevant information is used.
- Better Citation and Verifiability: Detailed citations and assessments improve transparency and trustworthiness.
- Customizable Behavior: Reflection tokens allow for task-specific adjustments.
- Efficient Inference: Offline critic model training reduces inference overhead.
Implementation with LangChain and LangGraph
The article details a practical implementation using LangChain and LangGraph, covering dependency setup, data model definition, document processing, evaluator configuration, RAG chain setup, workflow functions, workflow construction, and testing. The code demonstrates how to build a Self-RAG system capable of handling various queries and evaluating the relevance and accuracy of its responses.
Limitations of Self-RAG
Despite its advantages, Self-RAG has limitations:
- Not Fully Supported Outputs: Outputs may not always be fully supported by the cited evidence.
- Potential for Factual Errors: While improved, factual errors can still occur.
- Model Size Trade-offs: Smaller models might sometimes outperform larger ones in factual precision.
- Customization Trade-offs: Adjusting reflection token weights may impact other aspects of the output (e.g., fluency).
Conclusion
Self-RAG represents a significant advancement in LLM technology. By combining adaptive retrieval with self-reflection, it addresses key limitations of standard RAG, resulting in more accurate, relevant, and verifiable outputs. The framework's customizable nature allows for tailoring its behavior to diverse applications, making it a powerful tool for various tasks requiring high factual accuracy. The provided LangChain and LangGraph implementation offers a practical guide for building and deploying Self-RAG systems.
Frequently Asked Questions (FAQs) (The FAQs section from the original text is retained here.)
Q1. What is Self-RAG? A. Self-RAG (Self-Reflective Retrieval-Augmented Generation) is a framework that improves LLM performance by combining on-demand retrieval with self-reflection to enhance factual accuracy and relevance.
Q2. How does Self-RAG differ from standard RAG? A. Unlike standard RAG, Self-RAG retrieves passages only when needed, uses reflection tokens to critique its outputs, and adapts its behavior based on task requirements.
Q3. What are reflection tokens? A. Reflection tokens (ISREL, ISSUP, ISUSE) evaluate retrieval relevance, support for generated text, and overall utility, enabling self-assessment and better outputs.
Q4. What are the main advantages of Self-RAG? A. Self-RAG improves accuracy, reduces factual errors, offers better citations, and allows task-specific customization during inference.
Q5. Can Self-RAG completely eliminate factual inaccuracies? A. No, while Self-RAG reduces inaccuracies significantly, it is still prone to occasional factual errors like any LLM.
(Note: The image remains in its original format and location.)
The above is the detailed content of Self-RAG: AI That Knows When to Double-Check. For more information, please follow other related articles on the PHP Chinese website!
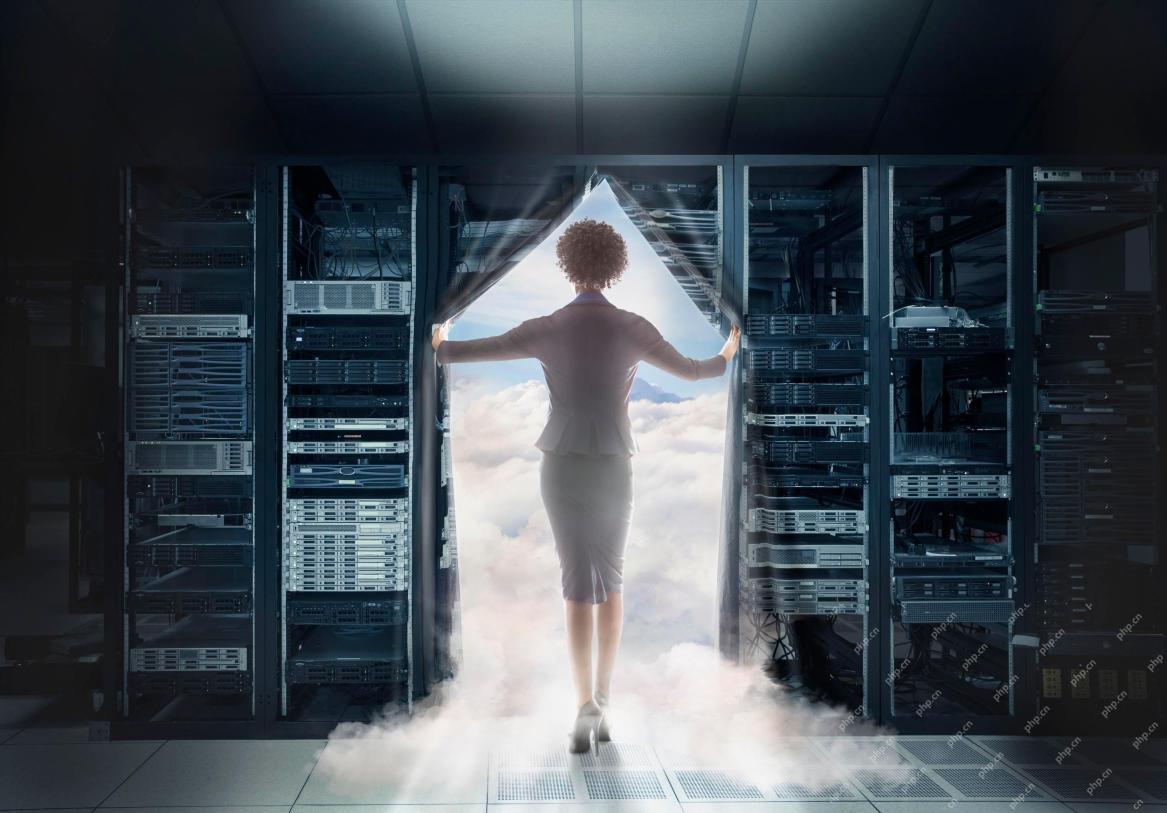
In John Rawls' seminal 1971 book The Theory of Justice, he proposed a thought experiment that we should take as the core of today's AI design and use decision-making: the veil of ignorance. This philosophy provides a simple tool for understanding equity and also provides a blueprint for leaders to use this understanding to design and implement AI equitably. Imagine that you are making rules for a new society. But there is a premise: you don’t know in advance what role you will play in this society. You may end up being rich or poor, healthy or disabled, belonging to a majority or marginal minority. Operating under this "veil of ignorance" prevents rule makers from making decisions that benefit themselves. On the contrary, people will be more motivated to formulate public
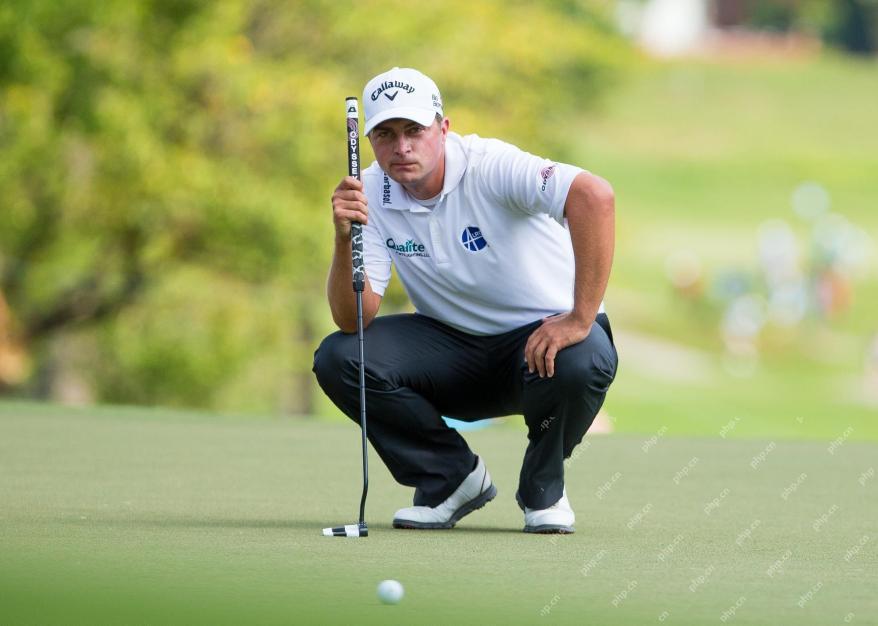
Numerous companies specialize in robotic process automation (RPA), offering bots to automate repetitive tasks—UiPath, Automation Anywhere, Blue Prism, and others. Meanwhile, process mining, orchestration, and intelligent document processing speciali
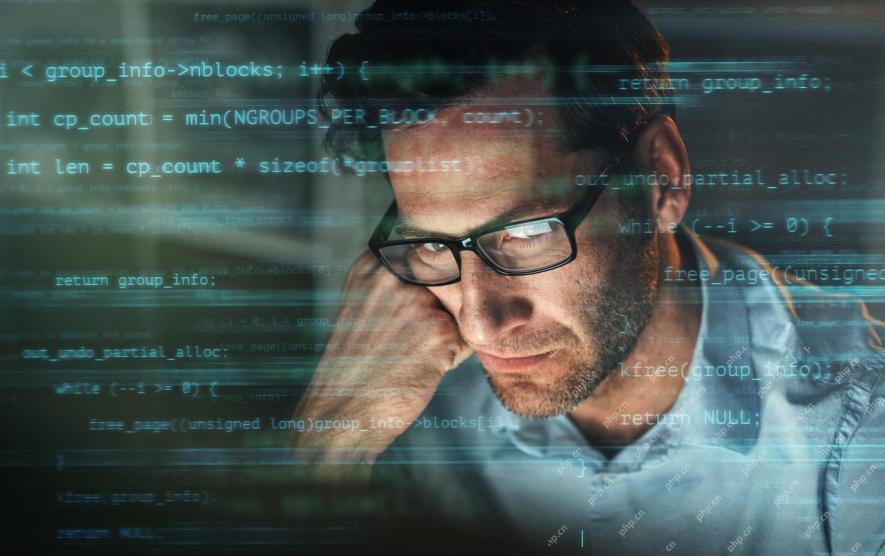
The future of AI is moving beyond simple word prediction and conversational simulation; AI agents are emerging, capable of independent action and task completion. This shift is already evident in tools like Anthropic's Claude. AI Agents: Research a
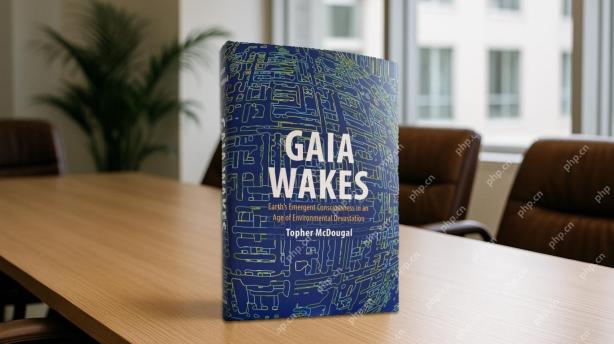
Rapid technological advancements necessitate a forward-looking perspective on the future of work. What happens when AI transcends mere productivity enhancement and begins shaping our societal structures? Topher McDougal's upcoming book, Gaia Wakes:
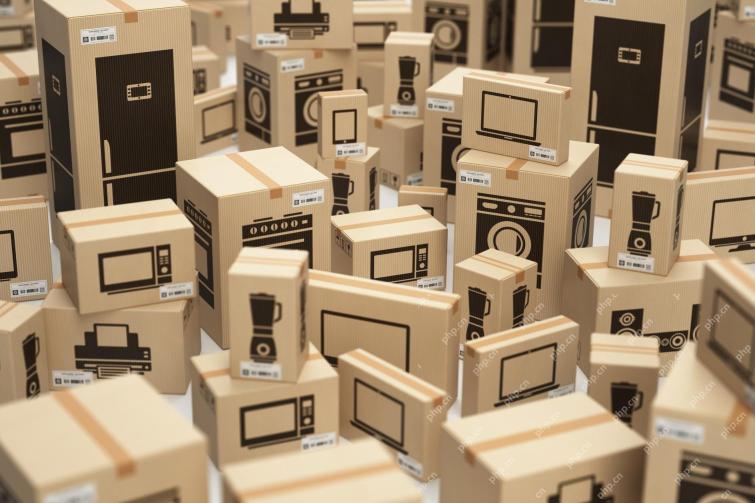
Product classification, often involving complex codes like "HS 8471.30" from systems such as the Harmonized System (HS), is crucial for international trade and domestic sales. These codes ensure correct tax application, impacting every inv
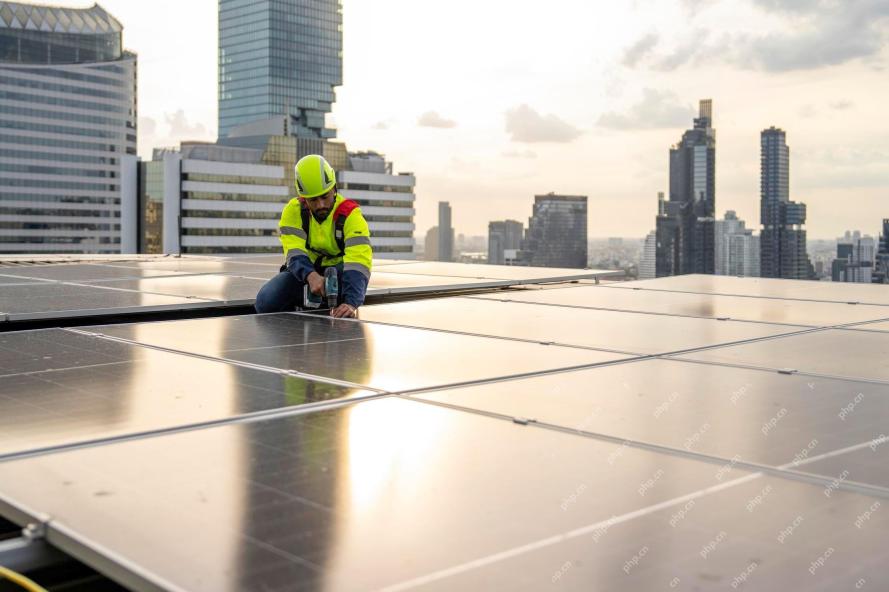
The future of energy consumption in data centers and climate technology investment This article explores the surge in energy consumption in AI-driven data centers and its impact on climate change, and analyzes innovative solutions and policy recommendations to address this challenge. Challenges of energy demand: Large and ultra-large-scale data centers consume huge power, comparable to the sum of hundreds of thousands of ordinary North American families, and emerging AI ultra-large-scale centers consume dozens of times more power than this. In the first eight months of 2024, Microsoft, Meta, Google and Amazon have invested approximately US$125 billion in the construction and operation of AI data centers (JP Morgan, 2024) (Table 1). Growing energy demand is both a challenge and an opportunity. According to Canary Media, the looming electricity
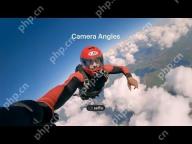
Generative AI is revolutionizing film and television production. Luma's Ray 2 model, as well as Runway's Gen-4, OpenAI's Sora, Google's Veo and other new models, are improving the quality of generated videos at an unprecedented speed. These models can easily create complex special effects and realistic scenes, even short video clips and camera-perceived motion effects have been achieved. While the manipulation and consistency of these tools still need to be improved, the speed of progress is amazing. Generative video is becoming an independent medium. Some models are good at animation production, while others are good at live-action images. It is worth noting that Adobe's Firefly and Moonvalley's Ma
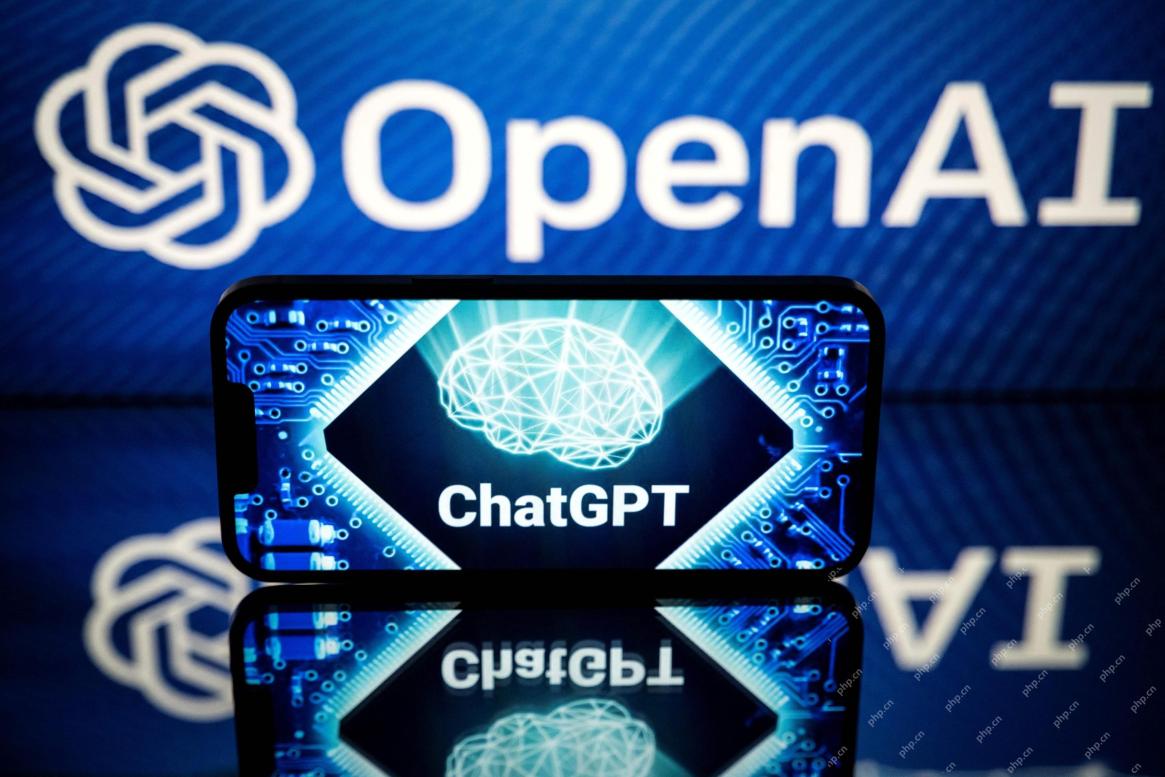
ChatGPT user experience declines: is it a model degradation or user expectations? Recently, a large number of ChatGPT paid users have complained about their performance degradation, which has attracted widespread attention. Users reported slower responses to models, shorter answers, lack of help, and even more hallucinations. Some users expressed dissatisfaction on social media, pointing out that ChatGPT has become “too flattering” and tends to verify user views rather than provide critical feedback. This not only affects the user experience, but also brings actual losses to corporate customers, such as reduced productivity and waste of computing resources. Evidence of performance degradation Many users have reported significant degradation in ChatGPT performance, especially in older models such as GPT-4 (which will soon be discontinued from service at the end of this month). this


Hot AI Tools

Undresser.AI Undress
AI-powered app for creating realistic nude photos

AI Clothes Remover
Online AI tool for removing clothes from photos.

Undress AI Tool
Undress images for free

Clothoff.io
AI clothes remover

Video Face Swap
Swap faces in any video effortlessly with our completely free AI face swap tool!

Hot Article

Hot Tools

SAP NetWeaver Server Adapter for Eclipse
Integrate Eclipse with SAP NetWeaver application server.

Zend Studio 13.0.1
Powerful PHP integrated development environment

Atom editor mac version download
The most popular open source editor
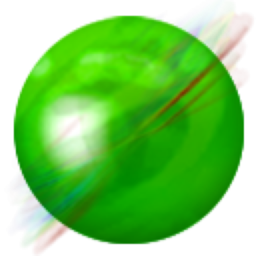
ZendStudio 13.5.1 Mac
Powerful PHP integrated development environment

mPDF
mPDF is a PHP library that can generate PDF files from UTF-8 encoded HTML. The original author, Ian Back, wrote mPDF to output PDF files "on the fly" from his website and handle different languages. It is slower than original scripts like HTML2FPDF and produces larger files when using Unicode fonts, but supports CSS styles etc. and has a lot of enhancements. Supports almost all languages, including RTL (Arabic and Hebrew) and CJK (Chinese, Japanese and Korean). Supports nested block-level elements (such as P, DIV),
