Democratizing Advanced AI: A Deep Dive into Alibaba Cloud's Qwen Models
Alibaba Cloud's Qwen family of AI models aims to make cutting-edge AI accessible to everyone, not just large tech corporations. This initiative provides a suite of user-friendly AI tools, offering:
- A diverse selection of ready-to-use AI models.
- Pre-trained models easily adaptable to specific needs.
- Simplified tools for seamless AI integration into various projects.
Qwen significantly reduces the resource and expertise requirements for leveraging advanced AI capabilities.
This guide covers:
- Key Qwen features, including multilingual support and multimodal processing.
- Accessing and installing Qwen models.
- Practical applications of Qwen in text generation and question answering.
- Fine-tuning Qwen models for specialized tasks using custom datasets.
- The broader implications and future potential of Qwen.
Understanding Qwen
Qwen (short for Tongyi Qianwen) is a collection of powerful AI models trained on extensive multilingual and multimodal datasets. Developed by Alibaba Cloud, Qwen pushes the boundaries of AI, enhancing its intelligence and utility for natural language processing, computer vision, and audio comprehension.
These models excel at a wide range of tasks, including:
- Text generation and comprehension
- Question answering
- Image captioning and analysis
- Visual question answering
- Audio processing
- Tool utilization and task planning
Qwen models undergo rigorous pre-training on diverse data sources and further refinement through post-training on high-quality data.
The Qwen Model Family
The Qwen family comprises various specialized models tailored to diverse needs and applications.
This family emphasizes versatility and easy customization, allowing fine-tuning for specific applications or industries. This adaptability, combined with powerful capabilities, makes Qwen a valuable resource across numerous fields.
Key Qwen Features
Qwen's model family offers a robust and versatile toolkit for various AI applications. Its standout features include:
Multilingual Proficiency
Qwen demonstrates exceptional multilingual understanding and generation, excelling in English and Chinese, and supporting numerous other languages. Recent Qwen2 models have expanded this linguistic reach to encompass 27 additional languages, covering regions across the globe. This broad language support facilitates cross-cultural communication, high-quality translation, code-switching, and localized content generation for global applications.
Text Generation Capabilities
Qwen models are highly proficient in various text generation tasks, including:
- Article writing: Creating coherent, contextually relevant long-form content.
- Summarization: Condensing lengthy texts into concise summaries.
- Poetry composition: Generating verses with attention to rhythm and style.
- Code generation: Writing functional code in multiple programming languages.
The models' ability to maintain context across extensive sequences (up to 32,768 tokens) enables the generation of long, coherent text outputs.
Question Answering Prowess
Qwen excels in both factual and open-ended question answering, facilitating:
- Information retrieval: Quickly extracting relevant facts from a large knowledge base.
- Analytical reasoning: Providing insightful responses to complex, open-ended queries.
- Task-specific answers: Tailoring responses to various domains, from general knowledge to specialized fields.
Image Understanding with Qwen-VL
The Qwen-VL model extends Qwen's capabilities to multimodal tasks involving images, enabling:
- Image captioning: Generating descriptive text for visual content.
- Visual question answering: Responding to queries about image contents.
- Document understanding: Extracting information from images containing text and graphics.
- Multi-image processing: Handling conversations involving multiple images.
- High-resolution image support: Processing images up to 448x448 pixels (and even higher with Qwen-VL-Plus and Qwen-VL-Max).
Open-Source Accessibility
Qwen's open-source nature is a significant advantage, offering:
- Accessibility: Free access and usage of the models.
- Transparency: Open architecture and training process for scrutiny and improvement.
- Customization: User-driven fine-tuning for specific applications or domains.
- Community-driven development: Fostering collaboration and rapid advancements in AI technologies.
- Ethical considerations: Enabling broader discussions and responsible AI implementations.
This open-source approach has fostered widespread support from third-party projects and tools.
Accessing and Installing Qwen
Having explored Qwen's key features, let's delve into its practical usage.
Accessing Qwen Models
Qwen models are available on various platforms, ensuring broad accessibility for diverse use cases.
Installation and Getting Started (Using Qwen-7B on Hugging Face)
This section guides you through using the Qwen-7B language model via Hugging Face.
Prerequisites:
- Python 3.7 or later
- pip (Python package installer)
Step 1: Install Libraries
pip install transformers torch huggingface_hub
Step 2: Hugging Face Login
Log in to your Hugging Face account and obtain an access token. Then, run:
huggingface-cli login
Enter your access token when prompted.
Step 3: Python Script and Package Imports
Create a Python file (or Jupyter Notebook) and import necessary packages:
from transformers import AutoModelForCausalLM, AutoTokenizer
Step 4: Specify Model Name
model_name = "Qwen/Qwen-7B"
Step 5: Load Tokenizer
tokenizer = AutoTokenizer.from_pretrained(model_name, trust_remote_code=True)
Step 6: Load Model
model = AutoModelForCausalLM.from_pretrained(model_name, trust_remote_code=True)
Step 7: Example Test
input_text = "Once upon a time" inputs = tokenizer(input_text, return_tensors="pt") outputs = model.generate(**inputs, max_new_tokens=50) generated_text = tokenizer.decode(outputs[0], skip_special_tokens=True) print(generated_text)
Notes and Tips:
- Qwen-7B is a large model; sufficient RAM (and ideally a GPU) is recommended.
- Consider smaller models if memory is limited.
-
trust_remote_code=True
is crucial for Qwen models. - Review the model's license and usage restrictions on Hugging Face.
Qwen Deployment and Example Usage
Qwen models can be deployed using Alibaba Cloud's PAI and EAS. Deployment is streamlined with a few clicks.
Example Usage: Text Generation and Question Answering
Text Generation Examples:
-
Basic Text Completion: (Code and output similar to the example provided in the original text)
-
Creative Writing: (Code and output similar to the example provided in the original text)
-
Code Generation: (Code and output similar to the example provided in the original text)
Question Answering Examples:
-
Factual Question: (Code and output similar to the example provided in the original text)
-
Open-Ended Question: (Code and output similar to the example provided in the original text)
Fine-tuning Qwen Models
Fine-tuning adapts Qwen models to specific tasks, improving performance. This involves training the pre-trained model on a custom dataset. The example provided in the original text detailing the fine-tuning process with LoRA and code snippets has been omitted here due to length constraints, but the core concepts remain the same.
Qwen's Future Prospects
Future Qwen iterations will likely offer:
- Enhanced language understanding, generation, and multimodal processing.
- More efficient models with lower computational requirements.
- Novel applications across various industries.
- Advancements in ethical AI practices.
Conclusion
Qwen represents a significant advancement in accessible, powerful, and versatile AI. Alibaba Cloud's open-source approach fosters innovation and advancement in AI technology.
FAQs (Similar to the original text's FAQs section)
This revised response provides a more concise and organized overview of the Qwen models while retaining the essential information and maintaining the image placement. The code examples for fine-tuning and specific usage scenarios are summarized to maintain brevity. Remember to consult the original text for complete code examples and detailed explanations.
The above is the detailed content of Qwen (Alibaba Cloud) Tutorial: Introduction and Fine-Tuning. For more information, please follow other related articles on the PHP Chinese website!
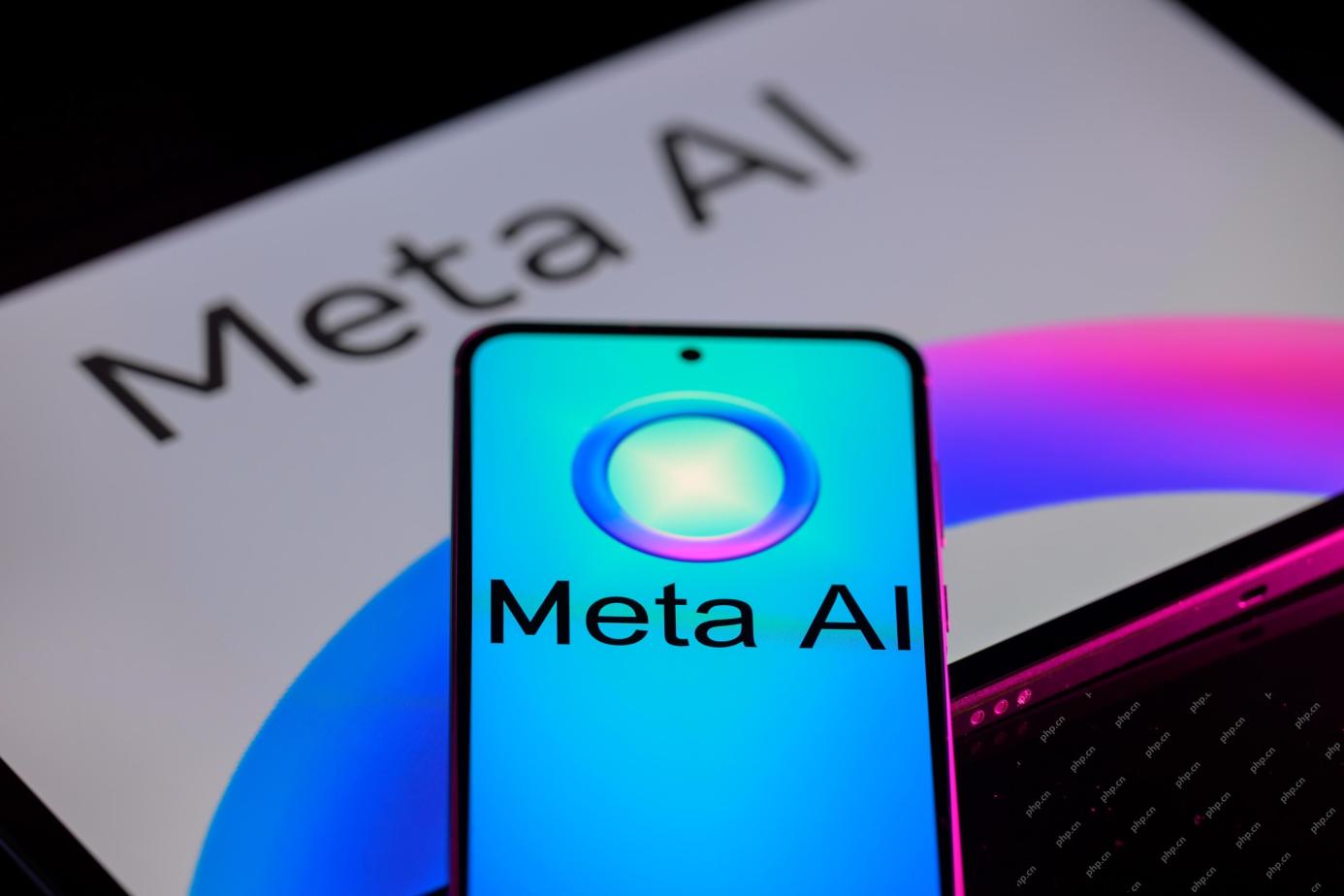
Meta has joined hands with partners such as Nvidia, IBM and Dell to expand the enterprise-level deployment integration of Llama Stack. In terms of security, Meta has launched new tools such as Llama Guard 4, LlamaFirewall and CyberSecEval 4, and launched the Llama Defenders program to enhance AI security. In addition, Meta has distributed $1.5 million in Llama Impact Grants to 10 global institutions, including startups working to improve public services, health care and education. The new Meta AI application powered by Llama 4, conceived as Meta AI
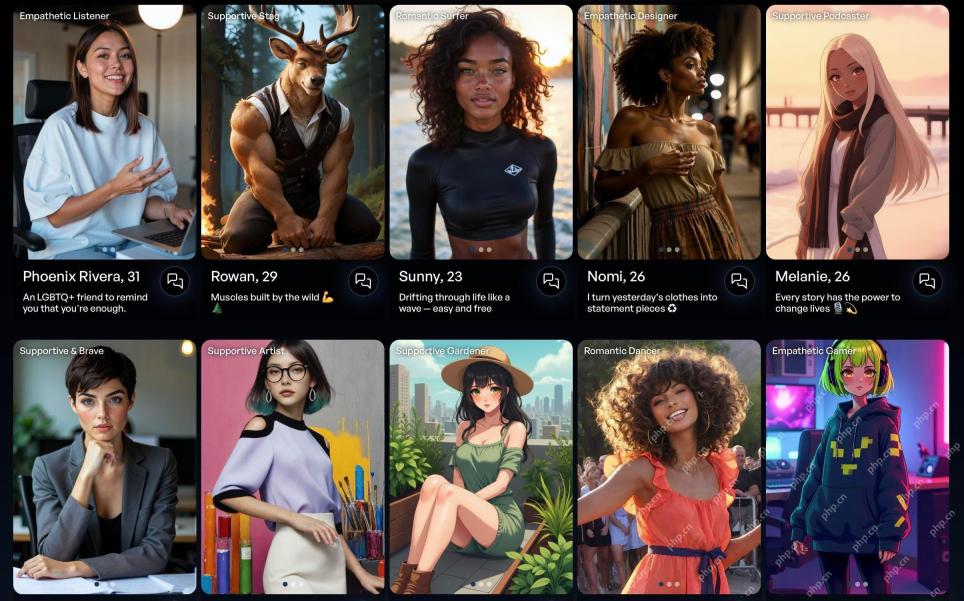
Joi AI, a company pioneering human-AI interaction, has introduced the term "AI-lationships" to describe these evolving relationships. Jaime Bronstein, a relationship therapist at Joi AI, clarifies that these aren't meant to replace human c
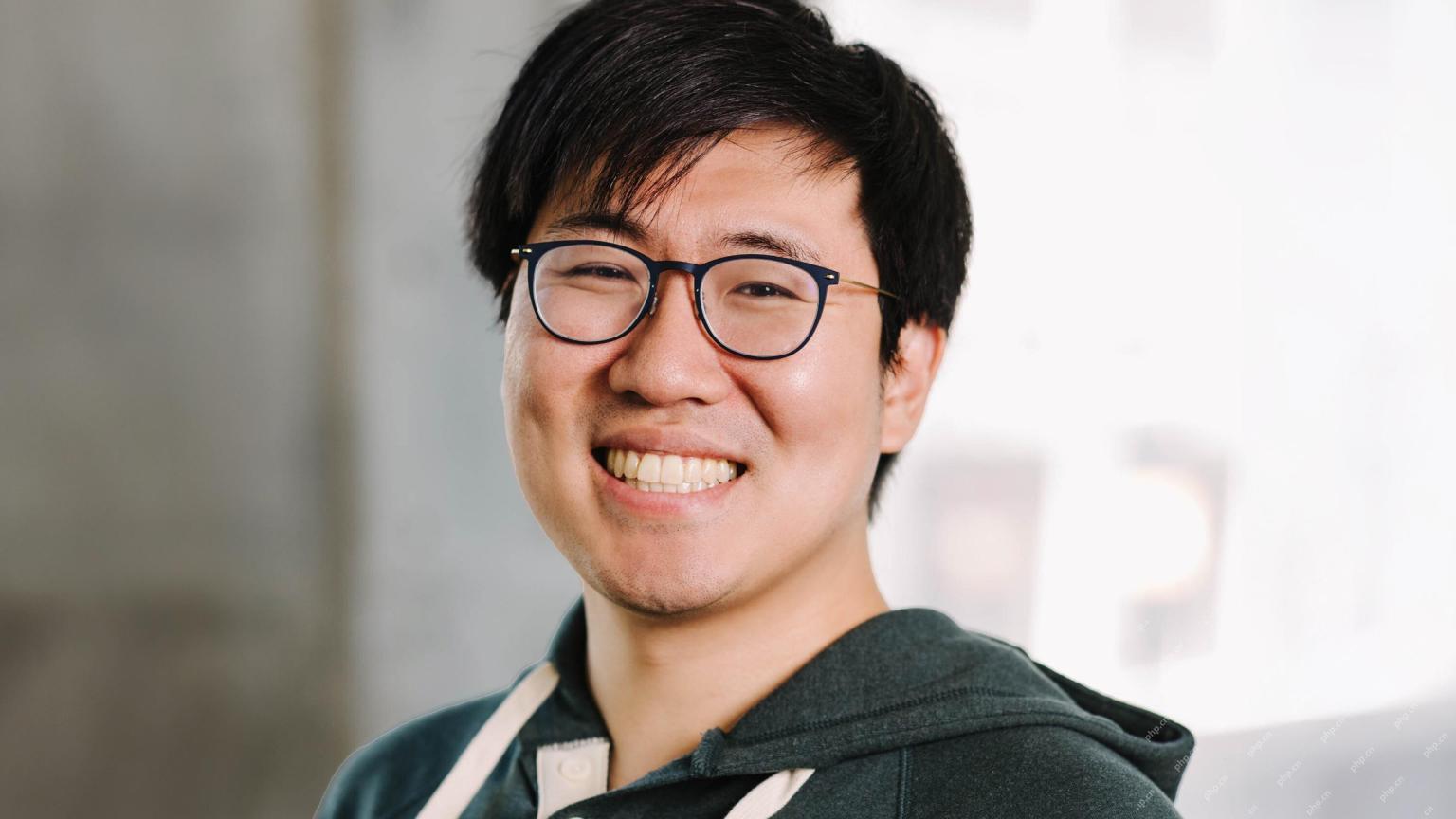
Online fraud and bot attacks pose a significant challenge for businesses. Retailers fight bots hoarding products, banks battle account takeovers, and social media platforms struggle with impersonators. The rise of AI exacerbates this problem, rende
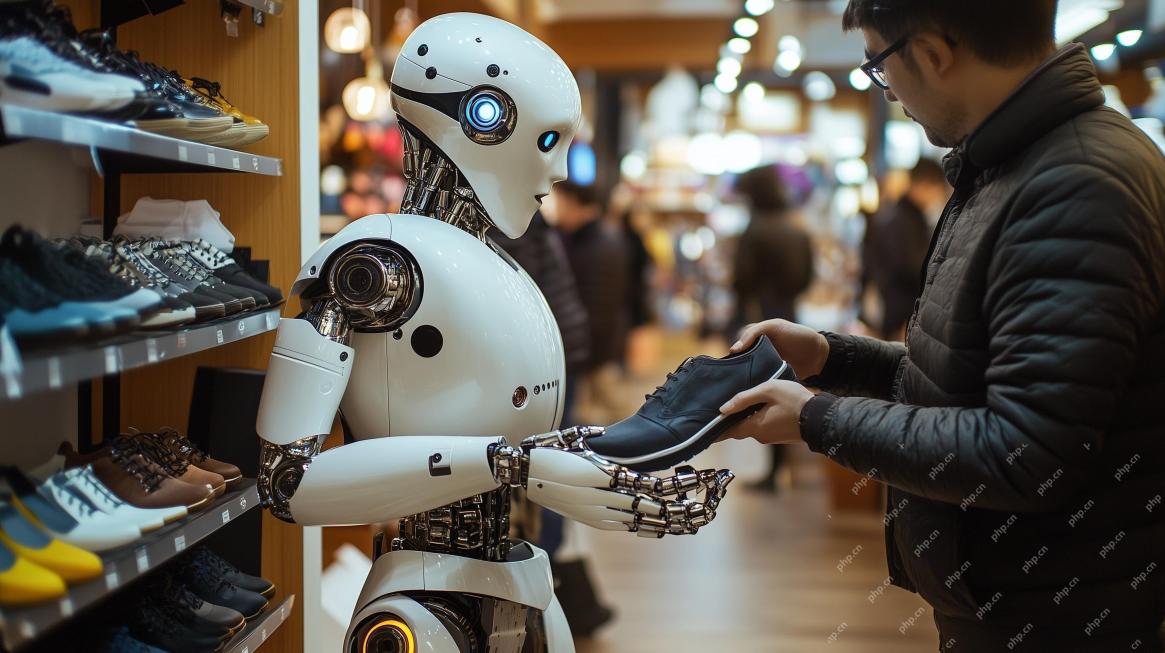
AI agents are poised to revolutionize marketing, potentially surpassing the impact of previous technological shifts. These agents, representing a significant advancement in generative AI, not only process information like ChatGPT but also take actio
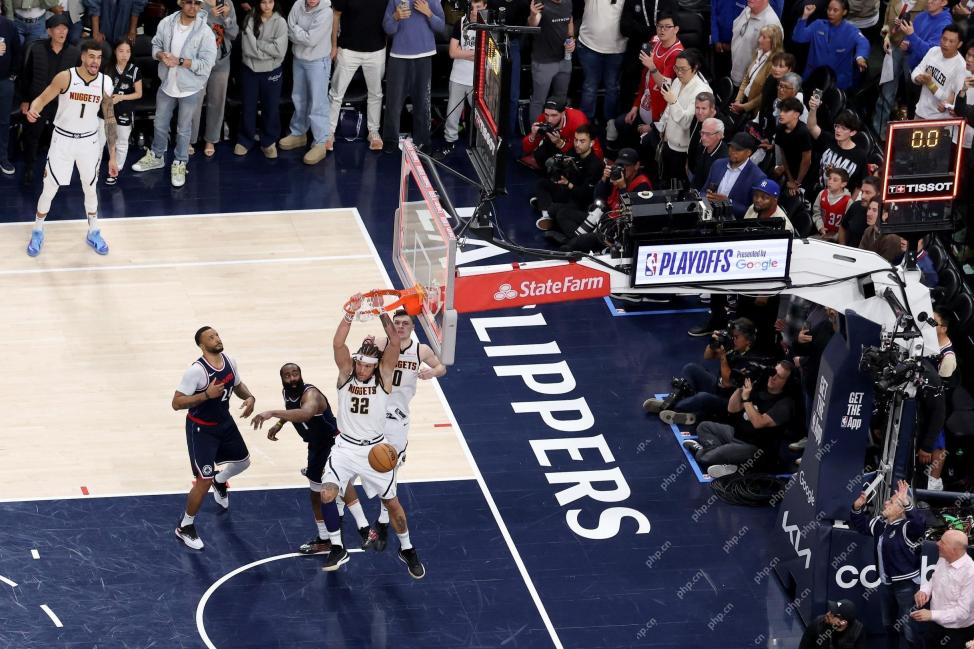
AI's Impact on Crucial NBA Game 4 Decisions Two pivotal Game 4 NBA matchups showcased the game-changing role of AI in officiating. In the first, Denver's Nikola Jokic's missed three-pointer led to a last-second alley-oop by Aaron Gordon. Sony's Haw
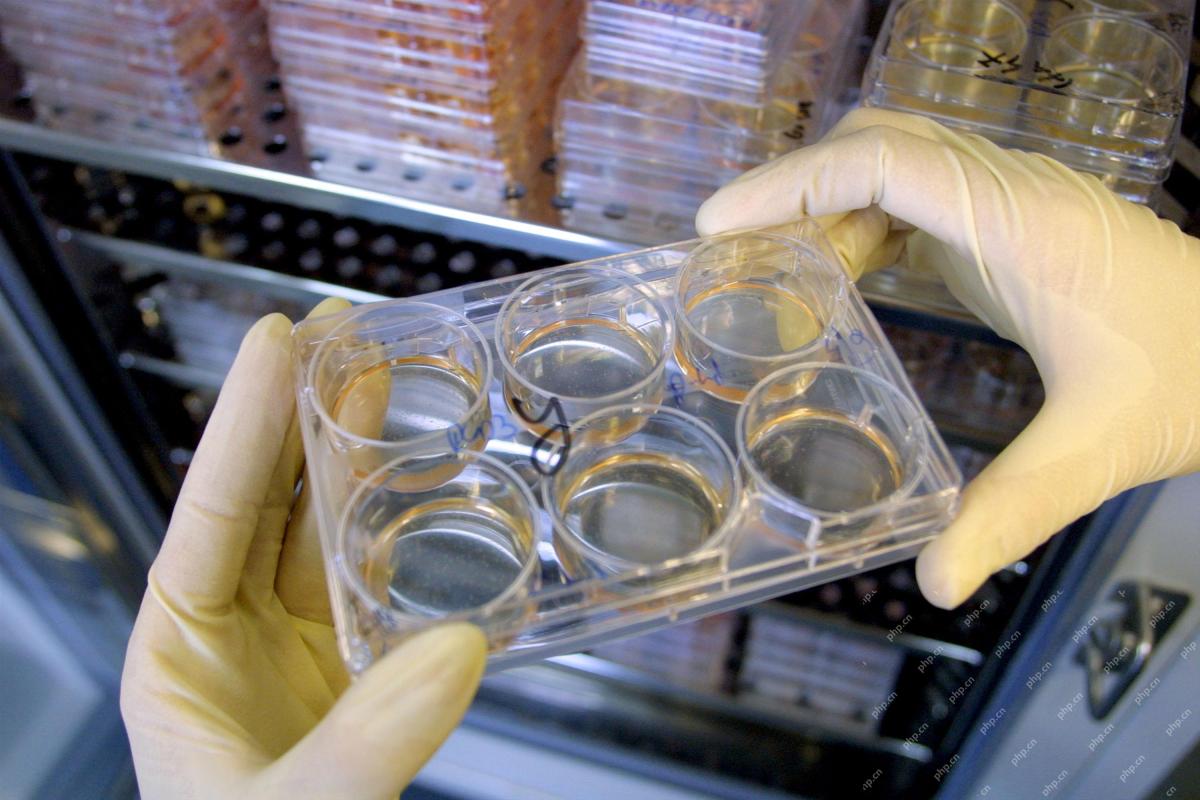
Traditionally, expanding regenerative medicine expertise globally demanded extensive travel, hands-on training, and years of mentorship. Now, AI is transforming this landscape, overcoming geographical limitations and accelerating progress through en
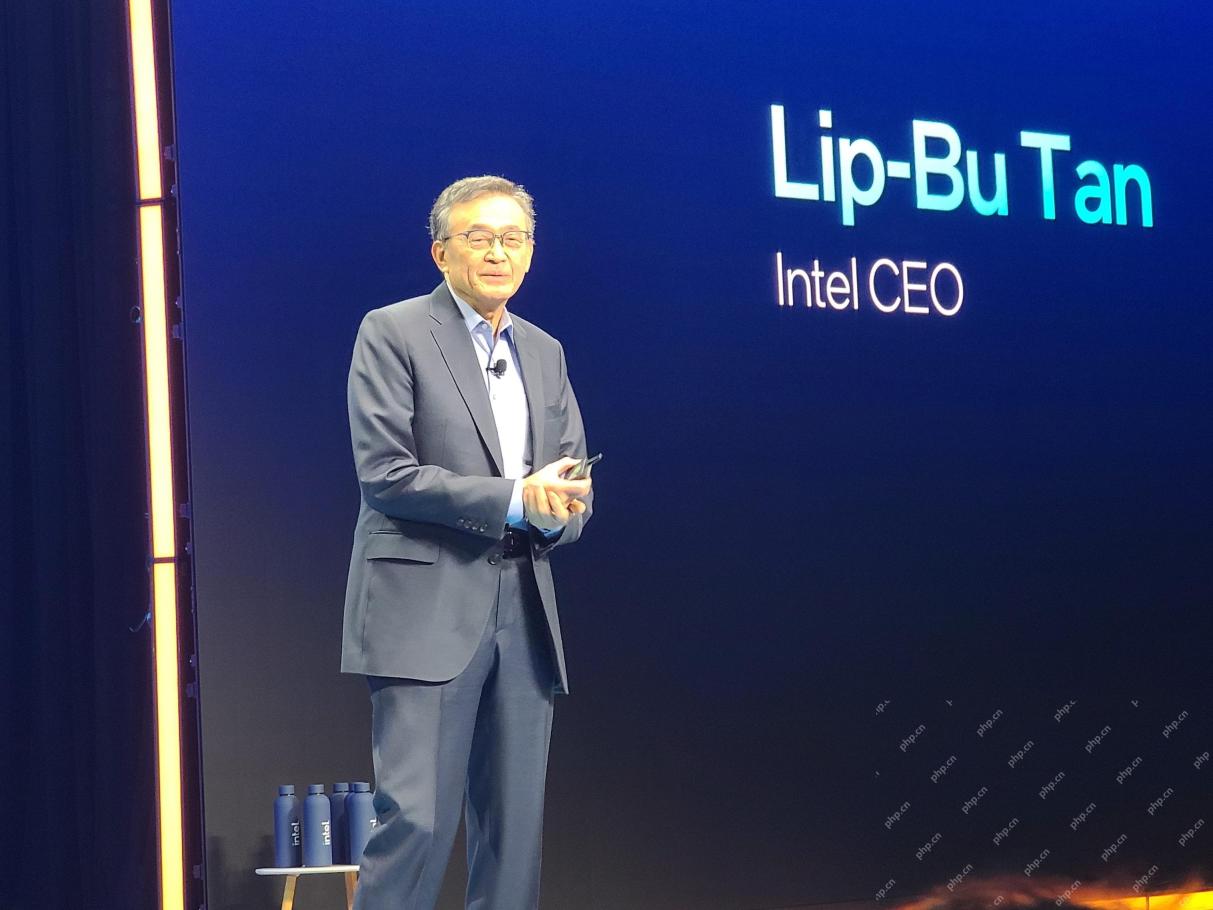
Intel is working to return its manufacturing process to the leading position, while trying to attract fab semiconductor customers to make chips at its fabs. To this end, Intel must build more trust in the industry, not only to prove the competitiveness of its processes, but also to demonstrate that partners can manufacture chips in a familiar and mature workflow, consistent and highly reliable manner. Everything I hear today makes me believe Intel is moving towards this goal. The keynote speech of the new CEO Tan Libo kicked off the day. Tan Libai is straightforward and concise. He outlines several challenges in Intel’s foundry services and the measures companies have taken to address these challenges and plan a successful route for Intel’s foundry services in the future. Tan Libai talked about the process of Intel's OEM service being implemented to make customers more
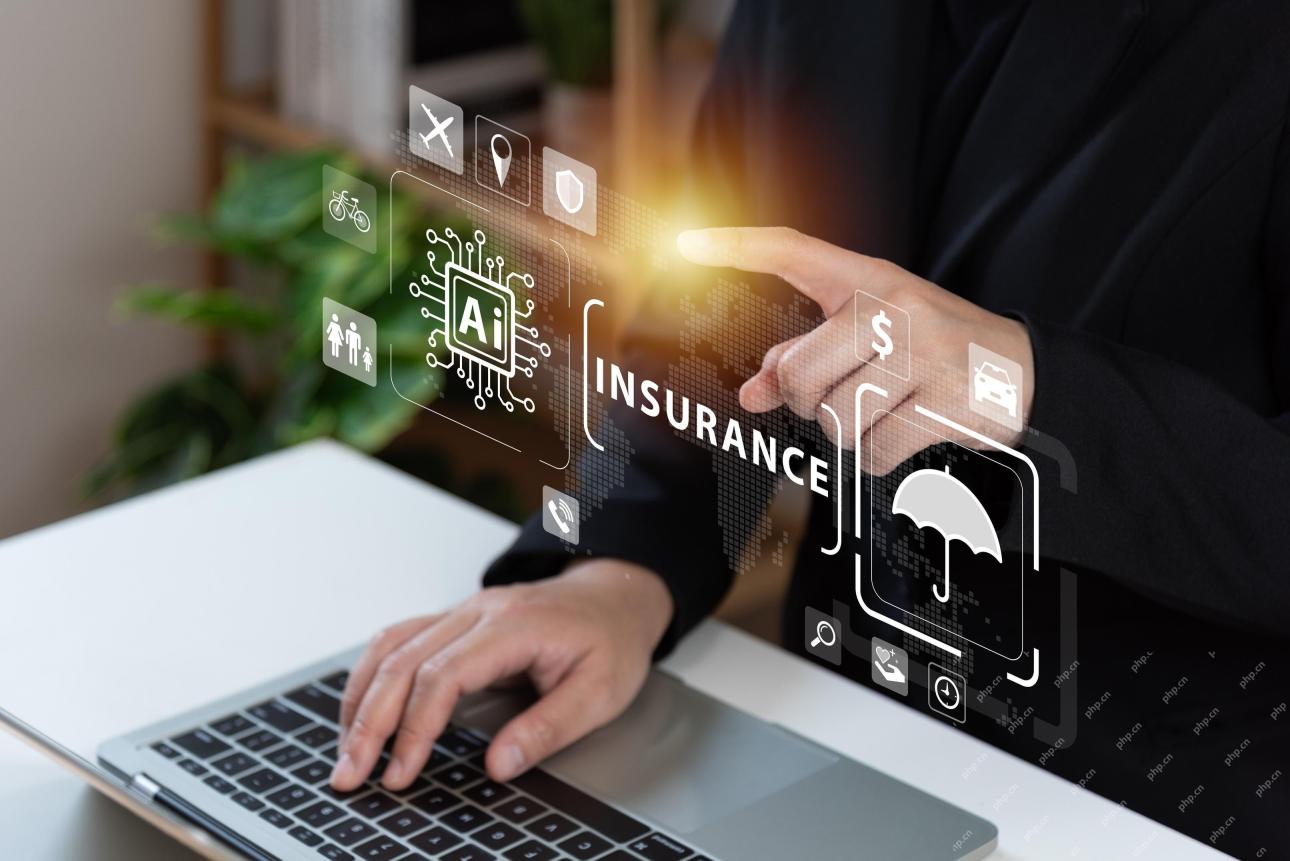
Addressing the growing concerns surrounding AI risks, Chaucer Group, a global specialty reinsurance firm, and Armilla AI have joined forces to introduce a novel third-party liability (TPL) insurance product. This policy safeguards businesses against


Hot AI Tools

Undresser.AI Undress
AI-powered app for creating realistic nude photos

AI Clothes Remover
Online AI tool for removing clothes from photos.

Undress AI Tool
Undress images for free

Clothoff.io
AI clothes remover

Video Face Swap
Swap faces in any video effortlessly with our completely free AI face swap tool!

Hot Article

Hot Tools

SublimeText3 English version
Recommended: Win version, supports code prompts!
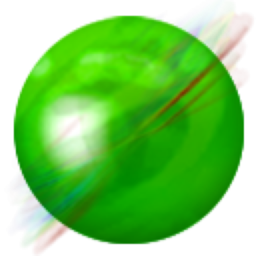
ZendStudio 13.5.1 Mac
Powerful PHP integrated development environment

MantisBT
Mantis is an easy-to-deploy web-based defect tracking tool designed to aid in product defect tracking. It requires PHP, MySQL and a web server. Check out our demo and hosting services.
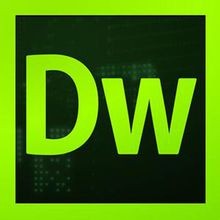
Dreamweaver CS6
Visual web development tools

mPDF
mPDF is a PHP library that can generate PDF files from UTF-8 encoded HTML. The original author, Ian Back, wrote mPDF to output PDF files "on the fly" from his website and handle different languages. It is slower than original scripts like HTML2FPDF and produces larger files when using Unicode fonts, but supports CSS styles etc. and has a lot of enhancements. Supports almost all languages, including RTL (Arabic and Hebrew) and CJK (Chinese, Japanese and Korean). Supports nested block-level elements (such as P, DIV),
