1、Import and EDA
import os iskaggle = os.environ.get('KAGGLE_KERNEL_RUN_TYPE', '') from pathlib import Path if iskaggle: path = Path('/kaggle/input/us-patent-phrase-to-phrase-matching')
import pandas as pd df = pd.read_csv(path/'train.csv') df['input'] = 'TEXT1: ' + df.context + '; TEXT2: ' + df.target + '; ANC1: ' + df.anchor df.input.head()
2、Tokenization
from datasets import Dataset, DatasetDict ds = Dataset.from_pandas(df) import warnings,logging,torch warnings.simplefilter('ignore') logging.disable(logging.WARNING) model_nm = 'anferico/bert-for-patents' # Load model directly from transformers import AutoModelForSequenceClassification, AutoTokenizer model = AutoModelForSequenceClassification.from_pretrained(model_nm, num_labels=1) tokenizer = AutoTokenizer.from_pretrained('anferico/bert-for-patents')
def tok_func(x): return tokenizer(x['input']) # Tokenize all the sentences using the tokenizer tok_ds = ds.map(tok_func, batched=True) tok_ds = tok_ds.rename_columns({'score':'labels'})
3、Test and Validation sets
eval_df = pd.read_csv(path/'test.csv') dds = tok_ds.train_test_split(0.25, seed=42) eval_df['input'] = 'TEXT1: ' + eval_df.context + '; TEXT2: ' + eval_df.target + '; ANC1: ' + eval_df.anchor eval_ds = Dataset.from_pandas(eval_df).map(tok_func, batched=True)
4、Metrics and Correlation
import numpy as np def corr(x,y): ## change the 2-d array into 1-d array return np.corrcoef(x.flatten(), y)[0,1] def corr_d(eval_pred): return {'pearson': corr(*eval_pred)}
5、Training our model
from transformers import TrainingArguments,Trainer bs = 128 epochs = 4 lr = 8e-5 args = TrainingArguments('outputs', learning_rate=lr, warmup_ratio=0.1, lr_scheduler_type='cosine', fp16=True, evaluation_strategy="epoch", per_device_train_batch_size=bs, per_device_eval_batch_size=bs*2, num_train_epochs=epochs, weight_decay=0.01, report_to='none') trainer = Trainer(model, args, train_dataset=dds['train'], eval_dataset=dds['test'], tokenizer=tokenizer, compute_metrics=corr_d) trainer.train()
6、Get the predictions on the test set
preds = trainer.predict(eval_ds).predictions.astype(float) preds = np.clip(preds, 0, 1) import datasets submission = datasets.Dataset.from_dict({ 'id': eval_ds['id'], 'score': preds }) submission.to_csv('submission.csv', index=False)
The above is the detailed content of Getting started with NLP using Bert on Kaggle. For more information, please follow other related articles on the PHP Chinese website!
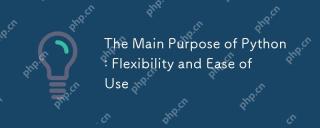
Python's flexibility is reflected in multi-paradigm support and dynamic type systems, while ease of use comes from a simple syntax and rich standard library. 1. Flexibility: Supports object-oriented, functional and procedural programming, and dynamic type systems improve development efficiency. 2. Ease of use: The grammar is close to natural language, the standard library covers a wide range of functions, and simplifies the development process.
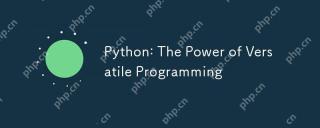
Python is highly favored for its simplicity and power, suitable for all needs from beginners to advanced developers. Its versatility is reflected in: 1) Easy to learn and use, simple syntax; 2) Rich libraries and frameworks, such as NumPy, Pandas, etc.; 3) Cross-platform support, which can be run on a variety of operating systems; 4) Suitable for scripting and automation tasks to improve work efficiency.
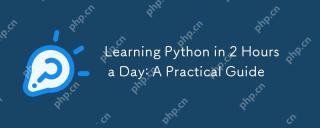
Yes, learn Python in two hours a day. 1. Develop a reasonable study plan, 2. Select the right learning resources, 3. Consolidate the knowledge learned through practice. These steps can help you master Python in a short time.
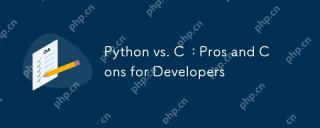
Python is suitable for rapid development and data processing, while C is suitable for high performance and underlying control. 1) Python is easy to use, with concise syntax, and is suitable for data science and web development. 2) C has high performance and accurate control, and is often used in gaming and system programming.
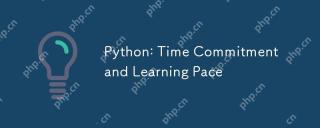
The time required to learn Python varies from person to person, mainly influenced by previous programming experience, learning motivation, learning resources and methods, and learning rhythm. Set realistic learning goals and learn best through practical projects.
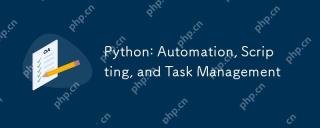
Python excels in automation, scripting, and task management. 1) Automation: File backup is realized through standard libraries such as os and shutil. 2) Script writing: Use the psutil library to monitor system resources. 3) Task management: Use the schedule library to schedule tasks. Python's ease of use and rich library support makes it the preferred tool in these areas.
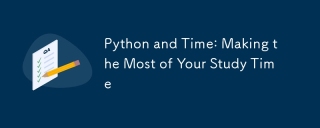
To maximize the efficiency of learning Python in a limited time, you can use Python's datetime, time, and schedule modules. 1. The datetime module is used to record and plan learning time. 2. The time module helps to set study and rest time. 3. The schedule module automatically arranges weekly learning tasks.
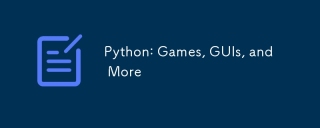
Python excels in gaming and GUI development. 1) Game development uses Pygame, providing drawing, audio and other functions, which are suitable for creating 2D games. 2) GUI development can choose Tkinter or PyQt. Tkinter is simple and easy to use, PyQt has rich functions and is suitable for professional development.


Hot AI Tools

Undresser.AI Undress
AI-powered app for creating realistic nude photos

AI Clothes Remover
Online AI tool for removing clothes from photos.

Undress AI Tool
Undress images for free

Clothoff.io
AI clothes remover

AI Hentai Generator
Generate AI Hentai for free.

Hot Article

Hot Tools

Safe Exam Browser
Safe Exam Browser is a secure browser environment for taking online exams securely. This software turns any computer into a secure workstation. It controls access to any utility and prevents students from using unauthorized resources.

Notepad++7.3.1
Easy-to-use and free code editor

MinGW - Minimalist GNU for Windows
This project is in the process of being migrated to osdn.net/projects/mingw, you can continue to follow us there. MinGW: A native Windows port of the GNU Compiler Collection (GCC), freely distributable import libraries and header files for building native Windows applications; includes extensions to the MSVC runtime to support C99 functionality. All MinGW software can run on 64-bit Windows platforms.

DVWA
Damn Vulnerable Web App (DVWA) is a PHP/MySQL web application that is very vulnerable. Its main goals are to be an aid for security professionals to test their skills and tools in a legal environment, to help web developers better understand the process of securing web applications, and to help teachers/students teach/learn in a classroom environment Web application security. The goal of DVWA is to practice some of the most common web vulnerabilities through a simple and straightforward interface, with varying degrees of difficulty. Please note that this software

PhpStorm Mac version
The latest (2018.2.1) professional PHP integrated development tool