Understanding Big O Notation: A Developer's Guide to Algorithm Efficiency
As a software developer, grasping Big O notation is essential, regardless of whether you're building web, mobile applications, or handling data processing. It's the key to evaluating algorithm efficiency, directly impacting application performance and scalability. The more you understand Big O, the better you'll be at code optimization.
This guide offers a thorough explanation of Big O notation, its significance, and how to analyze algorithms based on time and space complexity. We'll cover coding examples, real-world applications, and advanced concepts to provide a complete understanding.
Table of Contents
- What is Big O Notation?
- Why is Big O Notation Important?
- Key Big O Notations
- Advanced Big O Concepts
- Real-World Applications of Big O Notation
- Algorithm Optimization: Practical Solutions
- Conclusion
- Frequently Asked Questions (FAQs)
What is Big O Notation?
Big O notation is a mathematical tool for describing an algorithm's performance or complexity. Specifically, it shows how the algorithm's runtime or memory usage scales as the input size grows. Understanding Big O lets you predict how an algorithm will behave with large datasets.
Why is Big O Notation Important?
Consider a social media platform needing to handle millions of users and posts. Without optimized algorithms (analyzed using Big O), the platform could become slow or crash as user numbers increase. Big O helps you anticipate your code's performance with increasing input size (e.g., users or posts).
- Without Big O, you'd lack direction in code optimization.
- With Big O, you can design scalable, efficient algorithms even for massive datasets.
Key Big O Notations
-
Constant Time: O(1)
An O(1) algorithm performs a fixed number of operations regardless of input size. Its execution time remains constant as input grows.
Example: A function retrieving the first array element:
function getFirstElement(arr) { return arr[0]; }
The runtime is constant, regardless of array size – O(1).
Real-World Scenario: A vending machine dispensing a snack takes the same time regardless of the number of snacks available.
-
Logarithmic Time: O(log n)
Logarithmic time complexity arises when an algorithm halves the problem size with each iteration. This leads to O(log n) complexity, meaning runtime grows logarithmically with input size.
Example: Binary search is a classic example:
function getFirstElement(arr) { return arr[0]; }
Each iteration halves the search space, resulting in O(log n).
Real-World Scenario: Finding a name in a sorted phone book.
-
Linear Time: O(n)
O(n) complexity means runtime grows directly proportional to input size. Adding one element increases runtime by a constant amount.
Example: Finding the maximum element in an array:
function binarySearch(arr, target) { let low = 0; let high = arr.length - 1; while (low <= high) { let mid = Math.floor((low + high) / 2); if (arr[mid] === target) { return mid; } else if (arr[mid] < target) { low = mid + 1; } else { high = mid - 1; } } return -1; // Target not found }
The algorithm iterates through each element once – O(n).
Real-World Scenario: Processing a queue of people one by one.
-
Linearithmic Time: O(n log n)
O(n log n) is common in efficient sorting algorithms like Merge Sort and Quick Sort. They divide the input into smaller parts and process them efficiently.
Example: Merge Sort (implementation omitted for brevity). It recursively divides the array (log n) and merges (O(n)), resulting in O(n log n).
Real-World Scenario: Sorting a large group of people by height.
-
Quadratic Time: O(n²)
O(n²) algorithms usually have nested loops where each element in one loop is compared to every element in another.
Example: Bubble Sort (implementation omitted for brevity). The nested loops lead to O(n²).
Real-World Scenario: Comparing everyone's height to everyone else's in a group.
-
Cubic Time: O(n³)
Algorithms with three nested loops often have O(n³) complexity. This is common in algorithms working with multidimensional data structures like matrices.
Example: Simple matrix multiplication (implementation omitted for brevity) with three nested loops results in O(n³).
Real-World Scenario: Processing a 3D object in a graphics program.
Advanced Big O Concepts
-
Amortized Time Complexity: An algorithm might have occasional expensive operations, but the average cost over many operations is lower (e.g., dynamic array resizing).
-
Best, Worst, and Average Case: Big O often represents the worst-case scenario. However, best-case (Ω), worst-case (O), and average-case (Θ) complexities provide a more complete picture.
-
Space Complexity: Big O also analyzes an algorithm's memory usage (space complexity). Understanding both time and space complexity is crucial for optimization.
Conclusion
This guide covered Big O notation from basic to advanced concepts. By understanding and applying Big O analysis, you can write more efficient and scalable code. Continuously practicing this will make you a more proficient developer.
Frequently Asked Questions (FAQs)
- What is Big O notation? A mathematical description of algorithm performance (time and space) as input size grows.
- Why is Big O important? It helps optimize code for scalability and efficiency.
- Best, worst, average case differences? Best is the fastest, worst is the slowest, average is the expected performance.
- Time vs. space complexity? Time measures execution time; space measures memory usage.
- How to optimize using Big O? Analyze complexity and use techniques like caching or divide and conquer.
- Best sorting algorithm? Merge Sort and Quick Sort (O(n log n)) are efficient for large datasets.
- Can Big O be used for both time and space? Yes.
(Note: The images are assumed to be present and correctly linked as per the original input. The code examples are simplified for clarity. More robust implementations may exist.)
The above is the detailed content of Big-O Notation Simplified: Guide to Algorithm Efficiency | Mbloging. For more information, please follow other related articles on the PHP Chinese website!
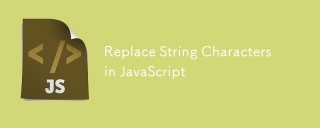
Detailed explanation of JavaScript string replacement method and FAQ This article will explore two ways to replace string characters in JavaScript: internal JavaScript code and internal HTML for web pages. Replace string inside JavaScript code The most direct way is to use the replace() method: str = str.replace("find","replace"); This method replaces only the first match. To replace all matches, use a regular expression and add the global flag g: str = str.replace(/fi
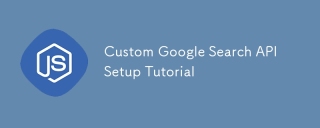
This tutorial shows you how to integrate a custom Google Search API into your blog or website, offering a more refined search experience than standard WordPress theme search functions. It's surprisingly easy! You'll be able to restrict searches to y
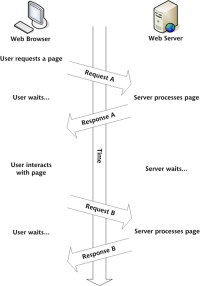
So here you are, ready to learn all about this thing called AJAX. But, what exactly is it? The term AJAX refers to a loose grouping of technologies that are used to create dynamic, interactive web content. The term AJAX, originally coined by Jesse J
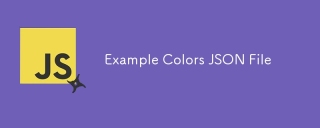
This article series was rewritten in mid 2017 with up-to-date information and fresh examples. In this JSON example, we will look at how we can store simple values in a file using JSON format. Using the key-value pair notation, we can store any kind
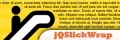
Leverage jQuery for Effortless Web Page Layouts: 8 Essential Plugins jQuery simplifies web page layout significantly. This article highlights eight powerful jQuery plugins that streamline the process, particularly useful for manual website creation
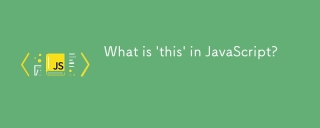
Core points This in JavaScript usually refers to an object that "owns" the method, but it depends on how the function is called. When there is no current object, this refers to the global object. In a web browser, it is represented by window. When calling a function, this maintains the global object; but when calling an object constructor or any of its methods, this refers to an instance of the object. You can change the context of this using methods such as call(), apply(), and bind(). These methods call the function using the given this value and parameters. JavaScript is an excellent programming language. A few years ago, this sentence was

jQuery is a great JavaScript framework. However, as with any library, sometimes it’s necessary to get under the hood to discover what’s going on. Perhaps it’s because you’re tracing a bug or are just curious about how jQuery achieves a particular UI
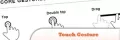
This post compiles helpful cheat sheets, reference guides, quick recipes, and code snippets for Android, Blackberry, and iPhone app development. No developer should be without them! Touch Gesture Reference Guide (PDF) A valuable resource for desig


Hot AI Tools

Undresser.AI Undress
AI-powered app for creating realistic nude photos

AI Clothes Remover
Online AI tool for removing clothes from photos.

Undress AI Tool
Undress images for free

Clothoff.io
AI clothes remover

AI Hentai Generator
Generate AI Hentai for free.

Hot Article

Hot Tools

SublimeText3 Chinese version
Chinese version, very easy to use

mPDF
mPDF is a PHP library that can generate PDF files from UTF-8 encoded HTML. The original author, Ian Back, wrote mPDF to output PDF files "on the fly" from his website and handle different languages. It is slower than original scripts like HTML2FPDF and produces larger files when using Unicode fonts, but supports CSS styles etc. and has a lot of enhancements. Supports almost all languages, including RTL (Arabic and Hebrew) and CJK (Chinese, Japanese and Korean). Supports nested block-level elements (such as P, DIV),

SublimeText3 Linux new version
SublimeText3 Linux latest version

MantisBT
Mantis is an easy-to-deploy web-based defect tracking tool designed to aid in product defect tracking. It requires PHP, MySQL and a web server. Check out our demo and hosting services.

SAP NetWeaver Server Adapter for Eclipse
Integrate Eclipse with SAP NetWeaver application server.
