


Advanced Database Query Optimization Techniques: A Practical Approach with Django
In today's fast-paced digital world, rapid data retrieval is paramount for application performance and user experience. Database queries are a critical component of many applications, and optimizing them is essential for scalability, reduced latency, and cost savings. This article explores advanced database query optimization techniques within the Django framework.
Understanding Query Optimization
Query optimization focuses on selecting the most efficient execution plan for a given database query. Just as there are multiple ways to solve a problem, multiple query approaches exist; optimization identifies the fastest and most resource-efficient method.
Why Optimize Queries?
Optimized queries deliver several key benefits:
- Improved Application Speed: Faster queries lead to a more responsive application.
- Reduced Server Load: Efficient queries lessen the strain on database servers.
- Enhanced User Experience: Users experience quicker load times and improved interaction.
- Lower Operating Costs: Optimized queries consume fewer resources, reducing expenses.
Advanced Query Optimization Techniques in Django
Here are several key techniques for optimizing Django database queries:
1. Leverage Database Indexes
Queries against unindexed fields force full table scans, significantly impacting performance. Indexes dramatically speed up queries, especially for large datasets.
Example: Indexed vs. Unindexed Field
# Unindexed field class Book(models.Model): title = models.CharField(max_length=200) # ... other fields # Indexed field class Book(models.Model): title = models.CharField(max_length=200, db_index=True) # ... other fields
2. Utilize select_related
and prefetch_related
These methods effectively address the N 1 query problem when retrieving related objects.
-
select_related
: Efficiently retrieves related data using SQL JOINs for single-valued relationships (ForeignKey, OneToOneField). -
prefetch_related
: Executes separate queries for multi-valued relationships (ManyToManyField, reverse ForeignKey) but caches the results to avoid redundant database hits.
Example: Avoiding N 1 Queries
# Inefficient (N+1 queries) books = Book.objects.all() for book in books: print(book.author.name) # Efficient (select_related) books = Book.objects.select_related('author') for book in books: print(book.author.name)
3. Conquer the N 1 Query Problem
The N 1 problem arises when related data is fetched repeatedly in a loop. prefetch_related
is the solution.
Example: Solving the N 1 Problem
# Inefficient (N+1 queries) books = Book.objects.all() for book in books: reviews = book.review_set.all() # Separate query for each book's reviews # Efficient (prefetch_related) books = Book.objects.prefetch_related('review_set') for book in books: print(book.review_set.all())
4. Filter Early, Retrieve Less Data
Filter data at the database level to minimize the amount of data transferred to your application.
Example: Efficient Filtering
# Unindexed field class Book(models.Model): title = models.CharField(max_length=200) # ... other fields # Indexed field class Book(models.Model): title = models.CharField(max_length=200, db_index=True) # ... other fields
5. Employ defer
and only
for Field Selection
Control which fields are retrieved, reducing data transfer.
-
defer
: Excludes specified fields. -
only
: Includes only specified fields.
Example: Selective Field Retrieval
# Inefficient (N+1 queries) books = Book.objects.all() for book in books: print(book.author.name) # Efficient (select_related) books = Book.objects.select_related('author') for book in books: print(book.author.name)
6. Implement Pagination for Large Datasets
Break down large datasets into smaller pages to improve performance and reduce memory consumption.
Example: Pagination
# Inefficient (N+1 queries) books = Book.objects.all() for book in books: reviews = book.review_set.all() # Separate query for each book's reviews # Efficient (prefetch_related) books = Book.objects.prefetch_related('review_set') for book in books: print(book.review_set.all())
7. Cache Frequently Accessed Queries
Store frequently used query results in a cache (like Redis or Memcached) to avoid repeated database hits.
8. Optimize Aggregations
Use Django's aggregation functions (e.g., Sum
, Avg
, Count
) for efficient database-level calculations.
9. Monitor and Profile Queries
Use Django's connection.queries
or a profiling tool (like Django Debug Toolbar) to identify performance bottlenecks.
10. Utilize Q Objects for Complex Queries
Improve readability and potentially efficiency for complex queries using Django's Q objects.
Conclusion
Database query optimization is crucial for maintaining the performance and scalability of Django applications. By consistently applying these techniques and monitoring query performance, developers can create highly responsive and efficient web applications.
The above is the detailed content of Advanced Database Query Optimization Techniques: A Practical Approach with Django. For more information, please follow other related articles on the PHP Chinese website!
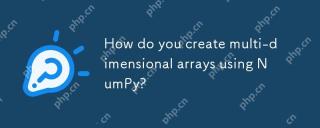
Create multi-dimensional arrays with NumPy can be achieved through the following steps: 1) Use the numpy.array() function to create an array, such as np.array([[1,2,3],[4,5,6]]) to create a 2D array; 2) Use np.zeros(), np.ones(), np.random.random() and other functions to create an array filled with specific values; 3) Understand the shape and size properties of the array to ensure that the length of the sub-array is consistent and avoid errors; 4) Use the np.reshape() function to change the shape of the array; 5) Pay attention to memory usage to ensure that the code is clear and efficient.
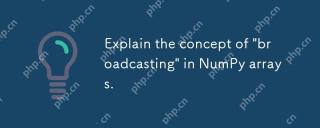
BroadcastinginNumPyisamethodtoperformoperationsonarraysofdifferentshapesbyautomaticallyaligningthem.Itsimplifiescode,enhancesreadability,andboostsperformance.Here'showitworks:1)Smallerarraysarepaddedwithonestomatchdimensions.2)Compatibledimensionsare
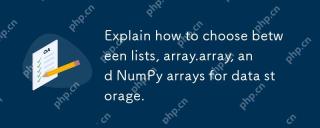
ForPythondatastorage,chooselistsforflexibilitywithmixeddatatypes,array.arrayformemory-efficienthomogeneousnumericaldata,andNumPyarraysforadvancednumericalcomputing.Listsareversatilebutlessefficientforlargenumericaldatasets;array.arrayoffersamiddlegro
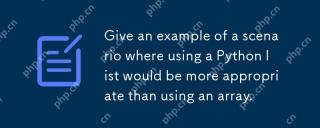
Pythonlistsarebetterthanarraysformanagingdiversedatatypes.1)Listscanholdelementsofdifferenttypes,2)theyaredynamic,allowingeasyadditionsandremovals,3)theyofferintuitiveoperationslikeslicing,but4)theyarelessmemory-efficientandslowerforlargedatasets.
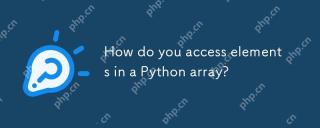
ToaccesselementsinaPythonarray,useindexing:my_array[2]accessesthethirdelement,returning3.Pythonuseszero-basedindexing.1)Usepositiveandnegativeindexing:my_list[0]forthefirstelement,my_list[-1]forthelast.2)Useslicingforarange:my_list[1:5]extractselemen
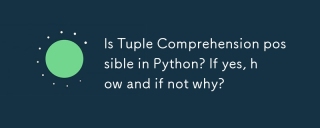
Article discusses impossibility of tuple comprehension in Python due to syntax ambiguity. Alternatives like using tuple() with generator expressions are suggested for creating tuples efficiently.(159 characters)
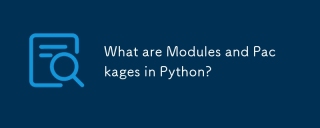
The article explains modules and packages in Python, their differences, and usage. Modules are single files, while packages are directories with an __init__.py file, organizing related modules hierarchically.
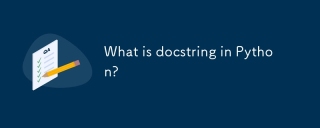
Article discusses docstrings in Python, their usage, and benefits. Main issue: importance of docstrings for code documentation and accessibility.


Hot AI Tools

Undresser.AI Undress
AI-powered app for creating realistic nude photos

AI Clothes Remover
Online AI tool for removing clothes from photos.

Undress AI Tool
Undress images for free

Clothoff.io
AI clothes remover

Video Face Swap
Swap faces in any video effortlessly with our completely free AI face swap tool!

Hot Article

Hot Tools
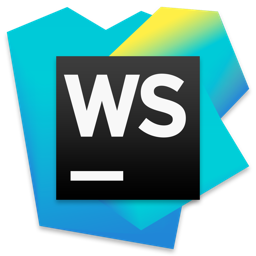
WebStorm Mac version
Useful JavaScript development tools

mPDF
mPDF is a PHP library that can generate PDF files from UTF-8 encoded HTML. The original author, Ian Back, wrote mPDF to output PDF files "on the fly" from his website and handle different languages. It is slower than original scripts like HTML2FPDF and produces larger files when using Unicode fonts, but supports CSS styles etc. and has a lot of enhancements. Supports almost all languages, including RTL (Arabic and Hebrew) and CJK (Chinese, Japanese and Korean). Supports nested block-level elements (such as P, DIV),

SublimeText3 English version
Recommended: Win version, supports code prompts!

SecLists
SecLists is the ultimate security tester's companion. It is a collection of various types of lists that are frequently used during security assessments, all in one place. SecLists helps make security testing more efficient and productive by conveniently providing all the lists a security tester might need. List types include usernames, passwords, URLs, fuzzing payloads, sensitive data patterns, web shells, and more. The tester can simply pull this repository onto a new test machine and he will have access to every type of list he needs.

Notepad++7.3.1
Easy-to-use and free code editor
