Introduction to RAG and GraphRAG
What is RAG?
RAG, or Retrieval-Augmented Generation, is a technology that combines information retrieval and text generation to generate more accurate and contextual responses. It works by retrieving relevant information from a knowledge base and then using this information to enhance the input to a large language model (LLM).
What is GraphRAG?
GraphRAG is an extension of the RAG framework, which combines knowledge of graph structures. GraphRAG leverages graph databases to represent and query complex relationships between entities and concepts, rather than using flat document-based retrieval systems.
Applications of RAG and GraphRAG
RAG App:
- Question and Answer System
- Chatbots and Virtual Assistants
- Content summary
- Fact checking and information verification
- Personalized content generation
GraphRAG application:
- Q&A based on knowledge graph
- Complex reasoning tasks
- Recommendation system
- Fraud Detection and Financial Analysis
- Scientific research and literature review
Advantages and Disadvantages of RAG
Advantages of RAG:
- Improved accuracy: By retrieving relevant information, RAG can provide more accurate and up-to-date responses.
- Reduce hallucinations: The retrieval step helps to base the model’s responses on factual information.
- Scalability: Easily update the knowledge base without retraining the entire model.
- Transparency: The retrieved documents can be used to explain the model’s reasoning process.
- Customizability: Can be customized for specific domains or use cases.
RAG Disadvantages:
- Latency: The retrieval step may introduce additional latency compared to purely generative models.
- Complexity: Implementing and maintaining a RAG system can be more complex than using a standalone LLM.
- Quality Dependence: The performance of the system largely depends on the quality and coverage of the knowledge base.
- May retrieve irrelevant information: If the retrieval system is not well tuned, it may retrieve irrelevant information.
- Storage requirements: Maintaining a large knowledge base can require significant resources.
Advantages and Disadvantages of GraphRAG
Advantages of GraphRAG:
- Complex relationship modeling: can represent and query intricate relationships between entities.
- Improving contextual understanding: Graph structures allow for better capture of contextual information.
- Multi-hop reasoning: Able to answer questions that require following multiple steps or connections.
- Flexibility: Various types of information and relationships can be combined in a unified framework.
- Efficient queries: Compared to traditional databases, graph databases may be more efficient for certain types of queries.
Disadvantages of GraphRAG:
- Increased complexity: Building and maintaining knowledge graphs is more complex than document-based systems.
- Higher computational requirements: Graph operations may require more computing resources.
- Data preparation challenges: Converting unstructured data into graph format can be time-consuming and error-prone.
- Possible overfitting: If the graph structure is too specific, it may not generalize well to new queries.
- Scalability issues: As a graph grows, it can become challenging to manage and query it efficiently.
Comparison of RAG and GraphRAG
When to use RAG:
- For general question answering system
- When processing mainly text information
- In scenarios where fast implementation and simplicity are required
- For applications that do not require complex relationship modeling
When to use GraphRAG:
- For domain-specific applications with complex relationships (e.g., scientific research, financial analysis)
- When multi-hop reasoning is critical
- In scenarios where understanding context and relationships is more important than raw text retrieval
- For applications that can benefit from structured knowledge representation
Future development direction and challenges
RAG’s progress:
- Improved search algorithm
- Better integration with LLM
- Real-time knowledge base updates
- Multi-modal RAG (combining images, audio, etc.)
Progress in GraphRAG:
- More efficient graph embedding technology
- Integrate with other AI technologies (e.g., reinforcement learning)
- Automated graph construction and maintenance
- Realizing explainable AI through graph structures
Common challenges:
- Guarantee data privacy and security
- Handling deviations in the knowledge base
- Improve calculation efficiency
- Enhance the interpretability of results
Conclusion
Both RAG and GraphRAG represent significant advances in enhancing language models with external knowledge. While RAG provides a more straightforward approach suitable for many general-purpose applications, GraphRAG provides a powerful framework for dealing with complex, relationship-rich domains. The choice between the two depends on the specific requirements of the application, the nature of the data, and the complexity of the inference tasks involved. As these technologies continue to develop, we can expect to see more sophisticated and efficient methods of combining retrieval, reasoning, and generation in AI systems.
The above is the detailed content of RAG vs GraphRAG. For more information, please follow other related articles on the PHP Chinese website!
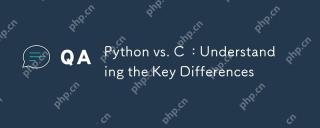
Python and C each have their own advantages, and the choice should be based on project requirements. 1) Python is suitable for rapid development and data processing due to its concise syntax and dynamic typing. 2)C is suitable for high performance and system programming due to its static typing and manual memory management.
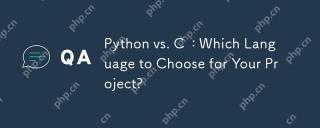
Choosing Python or C depends on project requirements: 1) If you need rapid development, data processing and prototype design, choose Python; 2) If you need high performance, low latency and close hardware control, choose C.
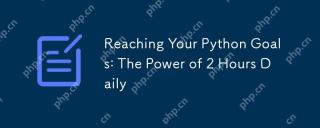
By investing 2 hours of Python learning every day, you can effectively improve your programming skills. 1. Learn new knowledge: read documents or watch tutorials. 2. Practice: Write code and complete exercises. 3. Review: Consolidate the content you have learned. 4. Project practice: Apply what you have learned in actual projects. Such a structured learning plan can help you systematically master Python and achieve career goals.
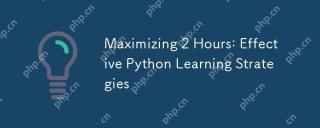
Methods to learn Python efficiently within two hours include: 1. Review the basic knowledge and ensure that you are familiar with Python installation and basic syntax; 2. Understand the core concepts of Python, such as variables, lists, functions, etc.; 3. Master basic and advanced usage by using examples; 4. Learn common errors and debugging techniques; 5. Apply performance optimization and best practices, such as using list comprehensions and following the PEP8 style guide.
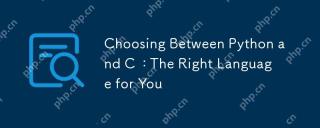
Python is suitable for beginners and data science, and C is suitable for system programming and game development. 1. Python is simple and easy to use, suitable for data science and web development. 2.C provides high performance and control, suitable for game development and system programming. The choice should be based on project needs and personal interests.
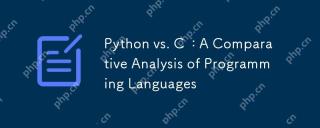
Python is more suitable for data science and rapid development, while C is more suitable for high performance and system programming. 1. Python syntax is concise and easy to learn, suitable for data processing and scientific computing. 2.C has complex syntax but excellent performance and is often used in game development and system programming.
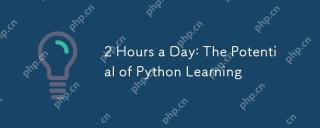
It is feasible to invest two hours a day to learn Python. 1. Learn new knowledge: Learn new concepts in one hour, such as lists and dictionaries. 2. Practice and exercises: Use one hour to perform programming exercises, such as writing small programs. Through reasonable planning and perseverance, you can master the core concepts of Python in a short time.
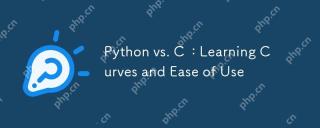
Python is easier to learn and use, while C is more powerful but complex. 1. Python syntax is concise and suitable for beginners. Dynamic typing and automatic memory management make it easy to use, but may cause runtime errors. 2.C provides low-level control and advanced features, suitable for high-performance applications, but has a high learning threshold and requires manual memory and type safety management.


Hot AI Tools

Undresser.AI Undress
AI-powered app for creating realistic nude photos

AI Clothes Remover
Online AI tool for removing clothes from photos.

Undress AI Tool
Undress images for free

Clothoff.io
AI clothes remover

Video Face Swap
Swap faces in any video effortlessly with our completely free AI face swap tool!

Hot Article

Hot Tools

MantisBT
Mantis is an easy-to-deploy web-based defect tracking tool designed to aid in product defect tracking. It requires PHP, MySQL and a web server. Check out our demo and hosting services.

mPDF
mPDF is a PHP library that can generate PDF files from UTF-8 encoded HTML. The original author, Ian Back, wrote mPDF to output PDF files "on the fly" from his website and handle different languages. It is slower than original scripts like HTML2FPDF and produces larger files when using Unicode fonts, but supports CSS styles etc. and has a lot of enhancements. Supports almost all languages, including RTL (Arabic and Hebrew) and CJK (Chinese, Japanese and Korean). Supports nested block-level elements (such as P, DIV),
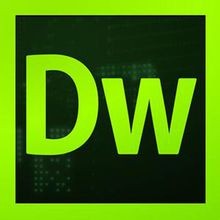
Dreamweaver CS6
Visual web development tools

DVWA
Damn Vulnerable Web App (DVWA) is a PHP/MySQL web application that is very vulnerable. Its main goals are to be an aid for security professionals to test their skills and tools in a legal environment, to help web developers better understand the process of securing web applications, and to help teachers/students teach/learn in a classroom environment Web application security. The goal of DVWA is to practice some of the most common web vulnerabilities through a simple and straightforward interface, with varying degrees of difficulty. Please note that this software
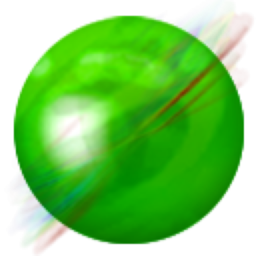
ZendStudio 13.5.1 Mac
Powerful PHP integrated development environment