Report generation using Anthropic’s Claude 3.5 Sonnet: Comparison of two methods
Hello everyone! I'm Raphael, co-founder and CTO of Brazilian real estate company Pilar. Pilar provides software and services to real estate agents and brokerage firms, using a low success fee model. Instead of charging high upfront fees, we take a small commission from each successful transaction, tying our success directly to our clients' success. Our team of 20 technologists are constantly innovating and the latest product is Pilar Homes, a new real estate portal designed to provide the best experience for homebuyers and real estate agents.
In this post, I will share our experience using artificial intelligence to generate reports, specifically Anthropic’s Claude 3.5 Sonnet, and compare two different methods.
Our philosophy for handling tasks will be detailed in a future article (stay tuned!), but in short, these tasks end up on the "Tech Help Desk" board as Jira tickets. Generating reports is one such task, with most tasks taking engineers about 30 minutes to solve, with complex reports rarely taking more than a few hours. But things are changing. The boutique brands we started out with one or two partners are expanding into larger agencies, and we're signing more contracts with established players in the industry. While increasing engineer hours could address the growing reporting needs, I saw an opportunity to explore AI agents and learn architectural patterns in real-world environments.
Method 1: Let AI fully handle and reach the max_tokens limit
In our initial approach, we exposed the tool to Claude's 3.5 Sonnet model, enabling it to perform database queries, convert retrieved documents to CSV, and write its results to a .csv file.
Here is our structure, heavily inspired by the blog post above:
<code># 每个collection对象描述一个MongoDB集合及其字段 # 这有助于Claude理解我们的数据模式 COLLECTIONS = [ { 'name': 'companies', 'description': 'Companies are the real estate brokerages. If the user provides a code to filter the data, it will be a company code. The _id may be retrieved by querying the company with the given code. Company codes are not used to join data.', 'fields': { '_id': 'The ObjectId is the MongoDB id that uniquely identifies a company document. Its JSON representation is \"{"$oid": "the id"}\"', 'code': 'The company code is a short and human friendly string that uniquely identifies the company. Never use it for joining data.', 'name': 'A string representing the company name', } }, # 此处之后描述了更多集合,但思路相同... ] # 这是client.messages.create的“system”参数 ROLE_PROMPT = "You are an engineer responsible for generating reports in CSV based on a user's description of the report content" # 这是“user”消息 task_prompt = f"{report_description}.\nAvailable collections: {COLLECTIONS}\nCompany codes: {company_codes}\n.Always demand a company code from the user to filter the data -- the user may use the terms imobiliária, marca, brand or company to reference a company. If the user wants a field that does not exist in a collection, don't add it to the report and don't ask the user for the field." </code>
report_description is just a command line argument read via argparse, company_codes is retrieved from the database and exposed to the model so that it knows which companies exist and what company codes are in the user input. Examples: (MO - Mosaic Homes, NV - Nova Real Estate, etc.).
Tools available for models include: find and docs2csv.
<code>def find(collection: str, query: str, fields: list[str]) -> Cursor: """Find documents in a collection filtering by "query" and retrieving fields via projection""" return db.get_collection(collection).find(query, projection={field: 1 for field in fields}) def docs2csv(documents: list[dict]) -> list[str]: """ Convert a dictionary to a CSV string. """ print(f"Converting {len(documents)} documents to CSV") with open('report.csv', mode='w', encoding='utf-8') as file: writer = csv.DictWriter(file, fieldnames=documents[0].keys()) writer.writeheader() writer.writerows(documents) return "report.csv"</code>
Claude was able to call the find function to perform well-structured queries and projections against our database and generate small CSV reports (less than 500 rows) using the docs2csv tool. However, larger reports trigger max_tokens errors.
After analyzing our token usage patterns, we realized that most of the token consumption comes from processing individual records through the model. This prompted us to explore another approach: letting Claude generate processing code instead of processing the data directly.
Method 2: Python code generation as a solution
While solving the max_tokens limit is not technically difficult, it requires us to rethink our approach to solving the problem.
Solution? Let Claude generate Python code that will run on our CPUs instead of processing each document through AI.
I had to modify the character and quest prompts and remove the tools.
The following is the gist of the report generation code.
The command to generate the report is:
<code># 每个collection对象描述一个MongoDB集合及其字段 # 这有助于Claude理解我们的数据模式 COLLECTIONS = [ { 'name': 'companies', 'description': 'Companies are the real estate brokerages. If the user provides a code to filter the data, it will be a company code. The _id may be retrieved by querying the company with the given code. Company codes are not used to join data.', 'fields': { '_id': 'The ObjectId is the MongoDB id that uniquely identifies a company document. Its JSON representation is \"{"$oid": "the id"}\"', 'code': 'The company code is a short and human friendly string that uniquely identifies the company. Never use it for joining data.', 'name': 'A string representing the company name', } }, # 此处之后描述了更多集合,但思路相同... ] # 这是client.messages.create的“system”参数 ROLE_PROMPT = "You are an engineer responsible for generating reports in CSV based on a user's description of the report content" # 这是“user”消息 task_prompt = f"{report_description}.\nAvailable collections: {COLLECTIONS}\nCompany codes: {company_codes}\n.Always demand a company code from the user to filter the data -- the user may use the terms imobiliária, marca, brand or company to reference a company. If the user wants a field that does not exist in a collection, don't add it to the report and don't ask the user for the field." </code>
Claude-generated Python content (working well):
<code>def find(collection: str, query: str, fields: list[str]) -> Cursor: """Find documents in a collection filtering by "query" and retrieving fields via projection""" return db.get_collection(collection).find(query, projection={field: 1 for field in fields}) def docs2csv(documents: list[dict]) -> list[str]: """ Convert a dictionary to a CSV string. """ print(f"Converting {len(documents)} documents to CSV") with open('report.csv', mode='w', encoding='utf-8') as file: writer = csv.DictWriter(file, fieldnames=documents[0].keys()) writer.writeheader() writer.writerows(documents) return "report.csv"</code>
Conclusion
Our journey with Claude 3.5 Sonnet shows that AI can significantly improve operational efficiency, but the key to success lies in choosing the right architecture. The code generation approach proved more powerful than direct AI processing while maintaining the benefits of automation.
In addition to building reports correctly, the code generation method also allows engineers to review the work of the AI, which is a very good thing.
To fully automate the process, eliminate human involvement and handle larger volumes of reports, distributing work across multiple agent instances - each handling fewer tokens - would be a natural evolution of the system. For architectural challenges in such distributed AI systems, I highly recommend Phil Calçado’s latest article on building AI products.
Key lessons learned from this implementation:
- Direct AI processing works for smaller data sets
- Code generation provides better scalability and maintainability
- Human review increases reliability
References
- Anthropic Documentation
- Thomas Taylor’s Anthropic Claude with tools using the Python SDK
- Building AI Products - Part 1: Backend Architecture by Phil Calçado
The above is the detailed content of Using Anthropics Claude Sonnet for Generating Reports. For more information, please follow other related articles on the PHP Chinese website!
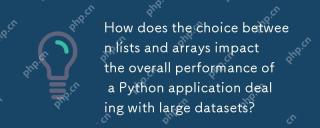
ForhandlinglargedatasetsinPython,useNumPyarraysforbetterperformance.1)NumPyarraysarememory-efficientandfasterfornumericaloperations.2)Avoidunnecessarytypeconversions.3)Leveragevectorizationforreducedtimecomplexity.4)Managememoryusagewithefficientdata
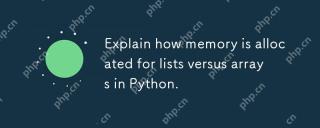
InPython,listsusedynamicmemoryallocationwithover-allocation,whileNumPyarraysallocatefixedmemory.1)Listsallocatemorememorythanneededinitially,resizingwhennecessary.2)NumPyarraysallocateexactmemoryforelements,offeringpredictableusagebutlessflexibility.
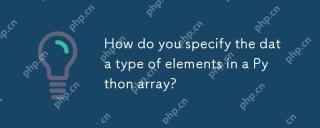
InPython, YouCansSpectHedatatYPeyFeLeMeReModelerErnSpAnT.1) UsenPyNeRnRump.1) UsenPyNeRp.DLOATP.PLOATM64, Formor PrecisconTrolatatypes.
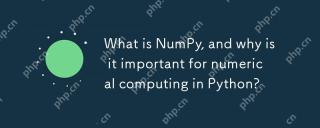
NumPyisessentialfornumericalcomputinginPythonduetoitsspeed,memoryefficiency,andcomprehensivemathematicalfunctions.1)It'sfastbecauseitperformsoperationsinC.2)NumPyarraysaremorememory-efficientthanPythonlists.3)Itoffersawiderangeofmathematicaloperation
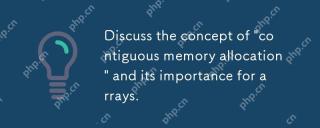
Contiguousmemoryallocationiscrucialforarraysbecauseitallowsforefficientandfastelementaccess.1)Itenablesconstanttimeaccess,O(1),duetodirectaddresscalculation.2)Itimprovescacheefficiencybyallowingmultipleelementfetchespercacheline.3)Itsimplifiesmemorym
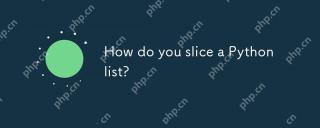
SlicingaPythonlistisdoneusingthesyntaxlist[start:stop:step].Here'showitworks:1)Startistheindexofthefirstelementtoinclude.2)Stopistheindexofthefirstelementtoexclude.3)Stepistheincrementbetweenelements.It'susefulforextractingportionsoflistsandcanuseneg
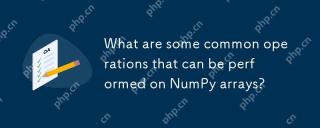
NumPyallowsforvariousoperationsonarrays:1)Basicarithmeticlikeaddition,subtraction,multiplication,anddivision;2)Advancedoperationssuchasmatrixmultiplication;3)Element-wiseoperationswithoutexplicitloops;4)Arrayindexingandslicingfordatamanipulation;5)Ag
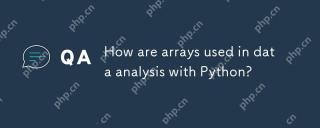
ArraysinPython,particularlythroughNumPyandPandas,areessentialfordataanalysis,offeringspeedandefficiency.1)NumPyarraysenableefficienthandlingoflargedatasetsandcomplexoperationslikemovingaverages.2)PandasextendsNumPy'scapabilitieswithDataFramesforstruc


Hot AI Tools

Undresser.AI Undress
AI-powered app for creating realistic nude photos

AI Clothes Remover
Online AI tool for removing clothes from photos.

Undress AI Tool
Undress images for free

Clothoff.io
AI clothes remover

Video Face Swap
Swap faces in any video effortlessly with our completely free AI face swap tool!

Hot Article

Hot Tools

SublimeText3 Chinese version
Chinese version, very easy to use

MinGW - Minimalist GNU for Windows
This project is in the process of being migrated to osdn.net/projects/mingw, you can continue to follow us there. MinGW: A native Windows port of the GNU Compiler Collection (GCC), freely distributable import libraries and header files for building native Windows applications; includes extensions to the MSVC runtime to support C99 functionality. All MinGW software can run on 64-bit Windows platforms.

Safe Exam Browser
Safe Exam Browser is a secure browser environment for taking online exams securely. This software turns any computer into a secure workstation. It controls access to any utility and prevents students from using unauthorized resources.

SecLists
SecLists is the ultimate security tester's companion. It is a collection of various types of lists that are frequently used during security assessments, all in one place. SecLists helps make security testing more efficient and productive by conveniently providing all the lists a security tester might need. List types include usernames, passwords, URLs, fuzzing payloads, sensitive data patterns, web shells, and more. The tester can simply pull this repository onto a new test machine and he will have access to every type of list he needs.

SAP NetWeaver Server Adapter for Eclipse
Integrate Eclipse with SAP NetWeaver application server.
