


My HyperGraph project's growth exposed significant technical debt, primarily manifested as crippling circular dependencies. This hindered maintainability and testing, prompting a complete architectural refactoring. This post details the challenges, the implemented solutions, and the resulting improvements.
The Challenges
Rapid initial development led to architectural compromises. As HyperGraph expanded, these issues became increasingly problematic:
- Inter-module circular dependencies.
- Tight coupling between components.
- Intractable testing scenarios.
- Complex and unpredictable initialization sequences.
- Poor separation of concerns.
The breaking point arrived during plugin system implementation. A dependency cycle involving the CLI, plugin system, and state service rendered clean architecture unattainable.
The Solution: A Modern Architectural Approach
My solution incorporated several key design patterns:
1. Interface-Driven Design
Prioritizing interfaces over concrete implementations decoupled modules. A dedicated interfaces
package defines contracts for all core components, eliminating circular dependencies.
2. Dependency Injection (DI)
A robust DI system manages:
- Service registration and resolution.
- Component lifecycle management.
- Configuration injection.
- Lazy loading.
This provides granular control over component initialization and dependencies.
3. Enhanced Lifecycle Management
A comprehensive lifecycle management system addresses:
- Component state transitions.
- Initialization order.
- Resource cleanup.
- Error handling.
4. Refined Package Structure
The restructured codebase features clear separation:
<code>hypergraph/ ├── core/ │ ├── di/ # Dependency Injection │ ├── interfaces/ # Core Interfaces │ ├── lifecycle.py # Lifecycle Management │ └── implementations/ ├── cli/ │ ├── interfaces/ │ └── implementations/</code>
The Results: Addressing Key Issues
The refactoring yielded substantial improvements:
- Eliminated Circular Dependencies: Interface-based dependencies resolved all circular dependencies.
- Simplified Testing: Interface-based mocking significantly eased unit testing.
- Improved Maintainability: Clearer separation of concerns enhanced code maintainability and readability.
- Increased Flexibility: The plugin system is now cleanly implemented.
- Robust Error Handling: Improved lifecycle management ensures more reliable error handling.
Future Possibilities: Unleashing Potential
The refactored architecture unlocks significant potential:
- Mature Plugin Ecosystem: A robust plugin system is now feasible.
- Streamlined Feature Expansion: Adding features is cleaner and more efficient.
- Comprehensive Testing: Thorough testing is now achievable.
- Sophisticated State Management: Advanced state management patterns can be implemented.
Key Learnings
This experience reinforced the long-term value of upfront architectural design. While initially seeming excessive, a clean separation of concerns and robust dependency management proves crucial as projects scale. The importance of lifecycle management in complex systems was also underscored. Well-defined states and transitions improve predictability and debuggability.
Looking Ahead
The new architecture provides a solid foundation for future development, including:
- A fully functional plugin system.
- Advanced state management capabilities.
- A more comprehensive testing framework.
- New CLI functionalities.
The extensive refactoring effort has undeniably paid off, resulting in a more maintainable, testable, and extensible codebase. The focus can now shift to feature development without architectural constraints. Sometimes, strategic regression is necessary for substantial progress.
The above is the detailed content of Solving Circular Dependencies: A Journey to Better Architecture. For more information, please follow other related articles on the PHP Chinese website!
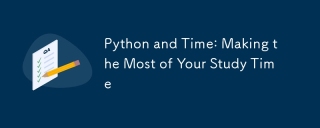
To maximize the efficiency of learning Python in a limited time, you can use Python's datetime, time, and schedule modules. 1. The datetime module is used to record and plan learning time. 2. The time module helps to set study and rest time. 3. The schedule module automatically arranges weekly learning tasks.
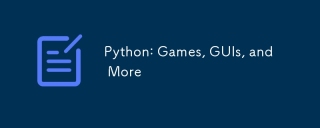
Python excels in gaming and GUI development. 1) Game development uses Pygame, providing drawing, audio and other functions, which are suitable for creating 2D games. 2) GUI development can choose Tkinter or PyQt. Tkinter is simple and easy to use, PyQt has rich functions and is suitable for professional development.
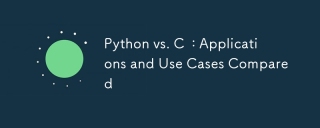
Python is suitable for data science, web development and automation tasks, while C is suitable for system programming, game development and embedded systems. Python is known for its simplicity and powerful ecosystem, while C is known for its high performance and underlying control capabilities.
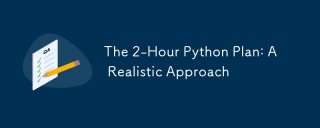
You can learn basic programming concepts and skills of Python within 2 hours. 1. Learn variables and data types, 2. Master control flow (conditional statements and loops), 3. Understand the definition and use of functions, 4. Quickly get started with Python programming through simple examples and code snippets.
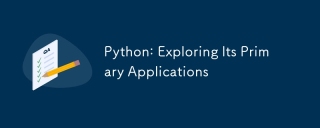
Python is widely used in the fields of web development, data science, machine learning, automation and scripting. 1) In web development, Django and Flask frameworks simplify the development process. 2) In the fields of data science and machine learning, NumPy, Pandas, Scikit-learn and TensorFlow libraries provide strong support. 3) In terms of automation and scripting, Python is suitable for tasks such as automated testing and system management.
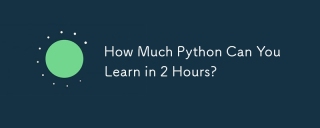
You can learn the basics of Python within two hours. 1. Learn variables and data types, 2. Master control structures such as if statements and loops, 3. Understand the definition and use of functions. These will help you start writing simple Python programs.
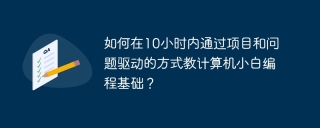
How to teach computer novice programming basics within 10 hours? If you only have 10 hours to teach computer novice some programming knowledge, what would you choose to teach...
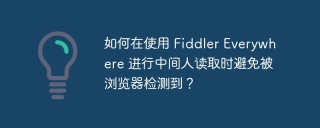
How to avoid being detected when using FiddlerEverywhere for man-in-the-middle readings When you use FiddlerEverywhere...


Hot AI Tools

Undresser.AI Undress
AI-powered app for creating realistic nude photos

AI Clothes Remover
Online AI tool for removing clothes from photos.

Undress AI Tool
Undress images for free

Clothoff.io
AI clothes remover

AI Hentai Generator
Generate AI Hentai for free.

Hot Article

Hot Tools
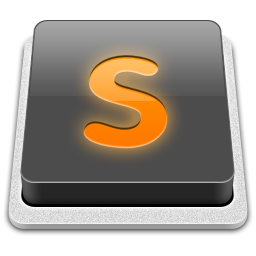
SublimeText3 Mac version
God-level code editing software (SublimeText3)

Safe Exam Browser
Safe Exam Browser is a secure browser environment for taking online exams securely. This software turns any computer into a secure workstation. It controls access to any utility and prevents students from using unauthorized resources.

MantisBT
Mantis is an easy-to-deploy web-based defect tracking tool designed to aid in product defect tracking. It requires PHP, MySQL and a web server. Check out our demo and hosting services.

SecLists
SecLists is the ultimate security tester's companion. It is a collection of various types of lists that are frequently used during security assessments, all in one place. SecLists helps make security testing more efficient and productive by conveniently providing all the lists a security tester might need. List types include usernames, passwords, URLs, fuzzing payloads, sensitive data patterns, web shells, and more. The tester can simply pull this repository onto a new test machine and he will have access to every type of list he needs.
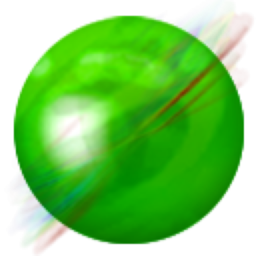
ZendStudio 13.5.1 Mac
Powerful PHP integrated development environment