Today's challenge tackles Day 10's puzzle, a 2D grid similar to Day 6, but requiring exploration of multiple paths. This puzzle elegantly showcases the power of depth-first search (DFS).
An AI-generated illustration of the puzzle
The map is represented as a dictionary; keys are (x, y) coordinates, and values are single-digit integers (0-9) indicating height, with 9 representing the peak. The parsing function efficiently handles this data structure:
def parse(input: str) -> dict[tuple[int, int], int | None]: return { (x, y): int(item) if item.isdigit() else None for y, row in enumerate(input.strip().splitlines()) for x, item in enumerate(row) }
Trails ascend from trailheads (height 0) to the peak (height 9), increasing height by exactly 1 per step. The next_step
function identifies valid next steps:
TRAIL_MAX = 9 def next_step( topo_map: dict[tuple[int, int], int | None], x: int, y: int ) -> tuple[tuple[int, int], ...]: assert topo_map[(x, y)] != TRAIL_MAX return tuple( incoming for incoming in ( (x + 1, y), (x, y + 1), (x - 1, y), (x, y - 1), ) if ( isinstance(topo_map.get(incoming), int) and isinstance(topo_map.get((x, y)), int) and (topo_map[incoming] - topo_map[(x, y)] == 1) ) )
Trailheads (height 0) are located using find_trailheads
:
TRAILHEAD = 0 def find_trailheads( topo_map: dict[tuple[int, int], int | None], ) -> tuple[tuple[int, int], ...]: return tuple(key for key, value in topo_map.items() if value == TRAILHEAD)
The core of the solution is the climb
function, which implements a depth-first search. Following Wikipedia's definition of DFS, we explore each branch fully before backtracking.
A visual representation of depth-first search
Map points are our "nodes," and we ascend one height level at a time. The climb
function manages the DFS process:
def climb( topo_map: dict[tuple[int, int], int | None], trailheads: tuple[tuple[int, int], ...] ) -> dict[ tuple[tuple[int, int], tuple[int, int]], tuple[tuple[tuple[int, int], ...], ...] ]: candidates: list[tuple[tuple[int, int], ...]] = [(head,) for head in trailheads] result = {} while candidates: current = candidates.pop() while True: if topo_map[current[-1]] == TRAIL_MAX: result[(current[0], current[-1])] = result.get( (current[0], current[-1]), () ) + (current,) break elif steps := next_step(topo_map, *current[-1]): incoming, *rest = steps candidates.extend([current + (step,) for step in rest]) current = current + (incoming,) else: break return result
The else
clause's break
handles dead ends, preventing infinite loops. The function returns all paths from each trailhead to the peak.
Part 1 counts the unique peak destinations:
def part1(input: str) -> int: topo_map = parse(input) return len(climb(topo_map, find_trailheads(topo_map)))
Part 2 counts all unique paths:
def part2(input: str) -> int: topo_map = parse(input) return sum( len(routes) for routes in climb(topo_map, find_trailheads(topo_map)).values() )
While alternative approaches exist (e.g., integrating trailhead detection into parsing), this solution's performance is acceptable. Recent job search setbacks haven't dampened my spirits; I remain hopeful. If you're seeking a mid-senior Python developer, please reach out. Until next week!
The above is the detailed content of Climbing a depth-first search hill, Advent of Code day 10. For more information, please follow other related articles on the PHP Chinese website!
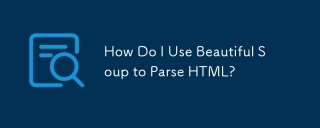
This article explains how to use Beautiful Soup, a Python library, to parse HTML. It details common methods like find(), find_all(), select(), and get_text() for data extraction, handling of diverse HTML structures and errors, and alternatives (Sel
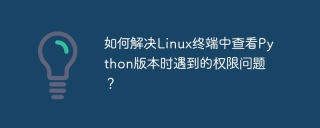
Solution to permission issues when viewing Python version in Linux terminal When you try to view Python version in Linux terminal, enter python...
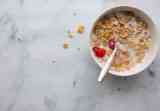
Serialization and deserialization of Python objects are key aspects of any non-trivial program. If you save something to a Python file, you do object serialization and deserialization if you read the configuration file, or if you respond to an HTTP request. In a sense, serialization and deserialization are the most boring things in the world. Who cares about all these formats and protocols? You want to persist or stream some Python objects and retrieve them in full at a later time. This is a great way to see the world on a conceptual level. However, on a practical level, the serialization scheme, format or protocol you choose may determine the speed, security, freedom of maintenance status, and other aspects of the program
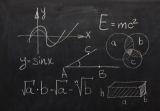
Python's statistics module provides powerful data statistical analysis capabilities to help us quickly understand the overall characteristics of data, such as biostatistics and business analysis. Instead of looking at data points one by one, just look at statistics such as mean or variance to discover trends and features in the original data that may be ignored, and compare large datasets more easily and effectively. This tutorial will explain how to calculate the mean and measure the degree of dispersion of the dataset. Unless otherwise stated, all functions in this module support the calculation of the mean() function instead of simply summing the average. Floating point numbers can also be used. import random import statistics from fracti
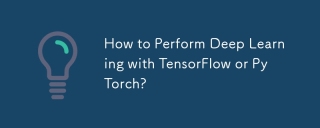
This article compares TensorFlow and PyTorch for deep learning. It details the steps involved: data preparation, model building, training, evaluation, and deployment. Key differences between the frameworks, particularly regarding computational grap
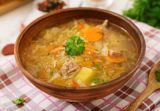
This tutorial builds upon the previous introduction to Beautiful Soup, focusing on DOM manipulation beyond simple tree navigation. We'll explore efficient search methods and techniques for modifying HTML structure. One common DOM search method is ex
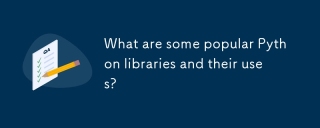
The article discusses popular Python libraries like NumPy, Pandas, Matplotlib, Scikit-learn, TensorFlow, Django, Flask, and Requests, detailing their uses in scientific computing, data analysis, visualization, machine learning, web development, and H
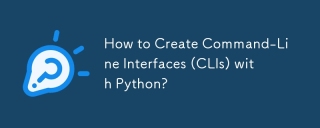
This article guides Python developers on building command-line interfaces (CLIs). It details using libraries like typer, click, and argparse, emphasizing input/output handling, and promoting user-friendly design patterns for improved CLI usability.


Hot AI Tools

Undresser.AI Undress
AI-powered app for creating realistic nude photos

AI Clothes Remover
Online AI tool for removing clothes from photos.

Undress AI Tool
Undress images for free

Clothoff.io
AI clothes remover

AI Hentai Generator
Generate AI Hentai for free.

Hot Article

Hot Tools

SublimeText3 English version
Recommended: Win version, supports code prompts!

MinGW - Minimalist GNU for Windows
This project is in the process of being migrated to osdn.net/projects/mingw, you can continue to follow us there. MinGW: A native Windows port of the GNU Compiler Collection (GCC), freely distributable import libraries and header files for building native Windows applications; includes extensions to the MSVC runtime to support C99 functionality. All MinGW software can run on 64-bit Windows platforms.

Notepad++7.3.1
Easy-to-use and free code editor

PhpStorm Mac version
The latest (2018.2.1) professional PHP integrated development tool
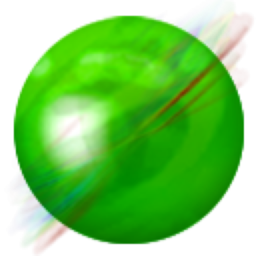
ZendStudio 13.5.1 Mac
Powerful PHP integrated development environment