


This article details building a local, two-way voice-enabled LLM server using Python, the Transformers library, Qwen2-Audio-7B-Instruct, and Bark. This setup allows for personalized voice interactions.
Prerequisites:
Before starting, ensure you have Python 3.9 , PyTorch, Transformers, Accelerate (in some cases), FFmpeg & pydub (audio processing), FastAPI (web server), Uvicorn (FastAPI server), Bark (text-to-speech), Multipart, and SciPy installed. Install FFmpeg using apt install ffmpeg
(Linux) or brew install ffmpeg
(macOS). Python dependencies can be installed via pip install torch transformers accelerate pydub fastapi uvicorn bark python-multipart scipy
.
Steps:
-
Environment Setup: Initialize your Python environment and select the PyTorch device (CUDA for GPU, CPU otherwise, or MPS for Apple Silicon, though MPS support may be limited).
import torch device = 'cuda' if torch.cuda.is_available() else 'cpu'
-
Model Loading: Load the Qwen2-Audio-7B-Instruct model and processor. For cloud GPU instances (Runpod, Vast), set
HF_HOME
andXDG_CACHE_HOME
environment variables to your volume storage before model download. Consider using a faster inference engine like vLLM in production.from transformers import AutoProcessor, Qwen2AudioForConditionalGeneration model_name = "Qwen/Qwen2-Audio-7B-Instruct" processor = AutoProcessor.from_pretrained(model_name) model = Qwen2AudioForConditionalGeneration.from_pretrained(model_name, device_map="auto").to(device)
-
Bark Model Loading: Load the Bark text-to-speech model. Alternatives exist, but proprietary options may be more expensive.
from bark import SAMPLE_RATE, generate_audio, preload_models preload_models()
The combined VRAM usage is approximately 24GB; use a quantized Qwen model if necessary.
-
FastAPI Server Setup: Create a FastAPI server with
/voice
and/text
endpoints for audio and text input respectively.from fastapi import FastAPI, UploadFile, Form from fastapi.responses import StreamingResponse import uvicorn app = FastAPI() # ... (API endpoints defined later) ... if __name__ == "__main__": uvicorn.run(app, host="0.0.0.0", port=8000)
-
Audio Input Processing: Use FFmpeg and pydub to process incoming audio into a format suitable for the Qwen model. Functions
audiosegment_to_float32_array
andload_audio_as_array
handle this conversion. -
Qwen Response Generation: The
generate_response
function takes a conversation (including audio or text) and uses the Qwen model to generate a textual response. It handles both audio and text inputs via the processor's chat template. -
Text-to-Speech Conversion: The
text_to_speech
function uses Bark to convert the generated text into a WAV audio file. -
API Endpoint Integration: The
/voice
and/text
endpoints are completed to handle input, generate a response usinggenerate_response
, and return the synthesized speech usingtext_to_speech
as a StreamingResponse. -
Testing: Use
curl
to test the server:import torch device = 'cuda' if torch.cuda.is_available() else 'cpu'
Complete Code: (The complete code is too long to include here, but it's available in the original prompt. The code snippets above show the key parts.)
Applications: This setup can be used as a foundation for chatbots, phone agents, customer support automation, and legal assistants.
This revised response provides a more structured and concise explanation, making it easier to understand and implement. The code snippets are more focused on the crucial aspects, while still maintaining the integrity of the original information.
The above is the detailed content of Homemade LLM Hosting with Two-Way Voice Support using Python, Transformers, Qwen, and Bark. For more information, please follow other related articles on the PHP Chinese website!
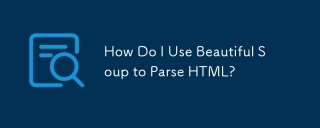
This article explains how to use Beautiful Soup, a Python library, to parse HTML. It details common methods like find(), find_all(), select(), and get_text() for data extraction, handling of diverse HTML structures and errors, and alternatives (Sel
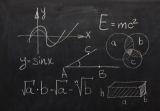
Python's statistics module provides powerful data statistical analysis capabilities to help us quickly understand the overall characteristics of data, such as biostatistics and business analysis. Instead of looking at data points one by one, just look at statistics such as mean or variance to discover trends and features in the original data that may be ignored, and compare large datasets more easily and effectively. This tutorial will explain how to calculate the mean and measure the degree of dispersion of the dataset. Unless otherwise stated, all functions in this module support the calculation of the mean() function instead of simply summing the average. Floating point numbers can also be used. import random import statistics from fracti
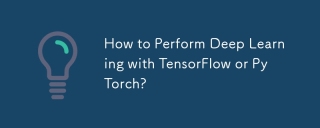
This article compares TensorFlow and PyTorch for deep learning. It details the steps involved: data preparation, model building, training, evaluation, and deployment. Key differences between the frameworks, particularly regarding computational grap
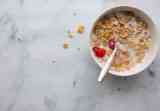
Serialization and deserialization of Python objects are key aspects of any non-trivial program. If you save something to a Python file, you do object serialization and deserialization if you read the configuration file, or if you respond to an HTTP request. In a sense, serialization and deserialization are the most boring things in the world. Who cares about all these formats and protocols? You want to persist or stream some Python objects and retrieve them in full at a later time. This is a great way to see the world on a conceptual level. However, on a practical level, the serialization scheme, format or protocol you choose may determine the speed, security, freedom of maintenance status, and other aspects of the program
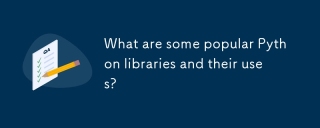
The article discusses popular Python libraries like NumPy, Pandas, Matplotlib, Scikit-learn, TensorFlow, Django, Flask, and Requests, detailing their uses in scientific computing, data analysis, visualization, machine learning, web development, and H
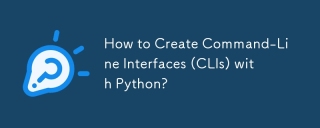
This article guides Python developers on building command-line interfaces (CLIs). It details using libraries like typer, click, and argparse, emphasizing input/output handling, and promoting user-friendly design patterns for improved CLI usability.
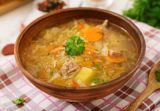
This tutorial builds upon the previous introduction to Beautiful Soup, focusing on DOM manipulation beyond simple tree navigation. We'll explore efficient search methods and techniques for modifying HTML structure. One common DOM search method is ex
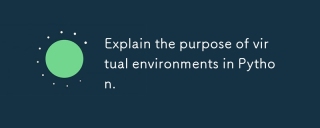
The article discusses the role of virtual environments in Python, focusing on managing project dependencies and avoiding conflicts. It details their creation, activation, and benefits in improving project management and reducing dependency issues.


Hot AI Tools

Undresser.AI Undress
AI-powered app for creating realistic nude photos

AI Clothes Remover
Online AI tool for removing clothes from photos.

Undress AI Tool
Undress images for free

Clothoff.io
AI clothes remover

AI Hentai Generator
Generate AI Hentai for free.

Hot Article

Hot Tools
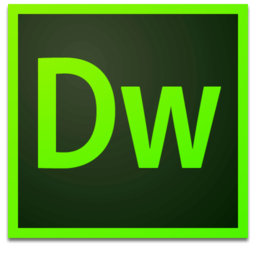
Dreamweaver Mac version
Visual web development tools

SublimeText3 Chinese version
Chinese version, very easy to use

SAP NetWeaver Server Adapter for Eclipse
Integrate Eclipse with SAP NetWeaver application server.

Safe Exam Browser
Safe Exam Browser is a secure browser environment for taking online exams securely. This software turns any computer into a secure workstation. It controls access to any utility and prevents students from using unauthorized resources.
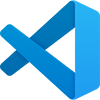
VSCode Windows 64-bit Download
A free and powerful IDE editor launched by Microsoft
