Hello everyone, my name is sea_turt1e.
This article will share the process and results of building a machine learning model to predict player chemistry in the National Basketball League (NBA), a sport I love very much.
Overview
- Predict player chemistry using graph neural networks (GNN).
- The area under the curve (AUC) is used as the evaluation metric.
- The AUC at convergence is approximately 0.73.
- The training data covers the 1996-97 season to the 2021-22 season, and the data from the 2022-23 season is used for testing.
Note: About NBA
For readers unfamiliar with the NBA, parts of this article may be difficult to understand. "Chemical reaction" can be understood from a more intuitive perspective. Additionally, while this article focuses on the NBA, the method could also be applied to other sports and even interpersonal chemistry prediction.
Chemical reaction prediction results
Let’s look at the prediction results first. I'll go into more detail about the dataset and technical details later.
Explanation of sides and fractions
In chemical reaction prediction, red edges indicate good chemical reactions, black edges indicate moderate chemical reactions, and blue edges indicate poor chemical reactions.
The fraction on the side represents the chemical reaction score, ranging from 0 to 1.
Chemistry predictions for star players
Here are the chemistry predictions for star players. The graph only contains pairs of players who never played for the same team.
Looking at the predictions of star players who have never played together, the results may not always be intuitive.
For example, LeBron James and Stephen Curry showed excellent coordination in the Olympics, indicating good chemistry. On the other hand, Nikola Jokic is surprisingly predicted to have poor chemistry with other players.
Chemistry predictions for major trades in 2022-23 season
To bring the predictions closer to reality, I tested the chemistry between players in actual trades for the 2022-23 season.
Since data from the 2022-23 season is not included in the training data, predictions that match realistic impressions can indicate the effectiveness of the model.
There are several important trades happening in the 2022-23 season.
Here are the predictions for key players including Kevin Durant, Kyrie Irving and Rui Hachimura.
The chemistry predictions for their new team are as follows:
- Lakers: Rui Hachimura – LeBron James (red edge: good chemistry)
- Suns: Kevin Durant – Chris Paul (Black Side: Medium chemistry)
- Mavericks: Kyrie Irving – Luka Doncic (blue side: poor chemistry)
These results appear to be pretty accurate considering the dynamics of the 2022-23 season. (Though things changed for the Suns and Mavericks the following season.)
Technical details
Next, I will explain the technical aspects, including the GNN framework and dataset preparation.
What is GNN?
GNN (Graph Neural Network) is a network designed to process graph-structured data.
In this model, "chemical reactions between players" are represented as graph edges, and the learning process is as follows:
- Direct side: The pair of players with higher number of assists.
- Negative Side: A pair of players with a lower number of assists.
For negative edges, the model gives priority to “teammates with low assists” and weakens the influence of “players from different teams”.
What is AUC?
AUC (area under the curve) refers to the area under the ROC curve and is used as a metric to evaluate model performance.
The closer the AUC is to 1, the higher the accuracy. In this study, the AUC of the model was approximately 0.73—a middling to above average result.
Learning Curve and AUC Progress
The following is the learning curve and AUC progress during the training process:
Dataset
The main innovation lies in the construction of the data set.
To quantify chemistry, I assume "high assists" means good chemistry. Based on this assumption, the data set is structured as follows:
- Positive side: Players with high number of assists.
- Negative side: Players with low assists.
Additionally, teammates with low assist counts are explicitly considered to have poor chemistry.
Code details
All code is available on GitHub.
Following the instructions in the README, you should be able to replicate the training process and plot the graphs described here.
https://www.php.cn/link/867079fcaff2dfddeb29ca1f27853ef7
Future Outlook
There is still room for improvement and I plan to achieve the following goals:
-
Expand the definition of chemical reaction
- Incorporate factors beyond assists to more accurately capture player relationships.
-
Improve accuracy
- Improving AUC through better training methods and expanded data sets.
-
Integrated natural language processing
- Analyze player interviews and social media posts to add new perspectives.
-
Write an article in English
- Publish content in English to reach a wider international audience.
-
Developing GUI for graph visualization
- Create a web application that allows users to interactively explore player chemistry.
Conclusion
In this article, I describe my attempts to predict NBA player chemistry.
While the model is still under development, I hope to achieve more exciting results with further improvements.
Welcome to leave your thoughts and suggestions in the comment area!
If you need further improvements, please let me know!
The above is the detailed content of Predicting NBA Player Chemistry Using Graph Neural Networks. For more information, please follow other related articles on the PHP Chinese website!
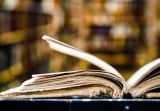
This tutorial demonstrates how to use Python to process the statistical concept of Zipf's law and demonstrates the efficiency of Python's reading and sorting large text files when processing the law. You may be wondering what the term Zipf distribution means. To understand this term, we first need to define Zipf's law. Don't worry, I'll try to simplify the instructions. Zipf's Law Zipf's law simply means: in a large natural language corpus, the most frequently occurring words appear about twice as frequently as the second frequent words, three times as the third frequent words, four times as the fourth frequent words, and so on. Let's look at an example. If you look at the Brown corpus in American English, you will notice that the most frequent word is "th
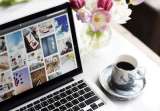
Dealing with noisy images is a common problem, especially with mobile phone or low-resolution camera photos. This tutorial explores image filtering techniques in Python using OpenCV to tackle this issue. Image Filtering: A Powerful Tool Image filter
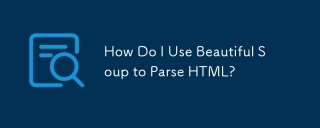
This article explains how to use Beautiful Soup, a Python library, to parse HTML. It details common methods like find(), find_all(), select(), and get_text() for data extraction, handling of diverse HTML structures and errors, and alternatives (Sel
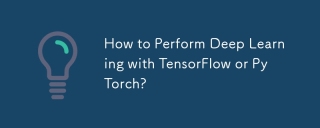
This article compares TensorFlow and PyTorch for deep learning. It details the steps involved: data preparation, model building, training, evaluation, and deployment. Key differences between the frameworks, particularly regarding computational grap
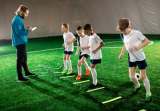
Python, a favorite for data science and processing, offers a rich ecosystem for high-performance computing. However, parallel programming in Python presents unique challenges. This tutorial explores these challenges, focusing on the Global Interprete
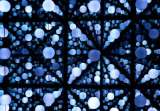
This tutorial demonstrates creating a custom pipeline data structure in Python 3, leveraging classes and operator overloading for enhanced functionality. The pipeline's flexibility lies in its ability to apply a series of functions to a data set, ge
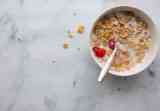
Serialization and deserialization of Python objects are key aspects of any non-trivial program. If you save something to a Python file, you do object serialization and deserialization if you read the configuration file, or if you respond to an HTTP request. In a sense, serialization and deserialization are the most boring things in the world. Who cares about all these formats and protocols? You want to persist or stream some Python objects and retrieve them in full at a later time. This is a great way to see the world on a conceptual level. However, on a practical level, the serialization scheme, format or protocol you choose may determine the speed, security, freedom of maintenance status, and other aspects of the program
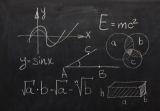
Python's statistics module provides powerful data statistical analysis capabilities to help us quickly understand the overall characteristics of data, such as biostatistics and business analysis. Instead of looking at data points one by one, just look at statistics such as mean or variance to discover trends and features in the original data that may be ignored, and compare large datasets more easily and effectively. This tutorial will explain how to calculate the mean and measure the degree of dispersion of the dataset. Unless otherwise stated, all functions in this module support the calculation of the mean() function instead of simply summing the average. Floating point numbers can also be used. import random import statistics from fracti


Hot AI Tools

Undresser.AI Undress
AI-powered app for creating realistic nude photos

AI Clothes Remover
Online AI tool for removing clothes from photos.

Undress AI Tool
Undress images for free

Clothoff.io
AI clothes remover

AI Hentai Generator
Generate AI Hentai for free.

Hot Article

Hot Tools

mPDF
mPDF is a PHP library that can generate PDF files from UTF-8 encoded HTML. The original author, Ian Back, wrote mPDF to output PDF files "on the fly" from his website and handle different languages. It is slower than original scripts like HTML2FPDF and produces larger files when using Unicode fonts, but supports CSS styles etc. and has a lot of enhancements. Supports almost all languages, including RTL (Arabic and Hebrew) and CJK (Chinese, Japanese and Korean). Supports nested block-level elements (such as P, DIV),

SublimeText3 Linux new version
SublimeText3 Linux latest version

Notepad++7.3.1
Easy-to-use and free code editor

PhpStorm Mac version
The latest (2018.2.1) professional PHP integrated development tool
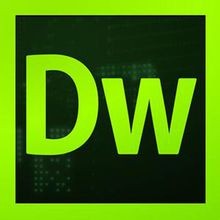
Dreamweaver CS6
Visual web development tools
