The Six Triple Eight relied on discipline and coordination to execute their mission. We’ll mirror this by creating and submitting a fine-tuning job, allowing the LLM to learn from our curated dataset.
Fine-Tuning with OpenAI
When you create a fine-tuning job via client.fine_tuning.job.create(), you submit your configuration and dataset to OpenAI for training. Below are the key parameters and their purposes.
1. Parameters Overview
model
- Description: The pre-trained GPT model you wish to fine-tune.
- Examples: "gpt-3.5-turbo", "davinci", "gpt-4-mini" (hypothetical).
training_file
- Description: The file ID of an uploaded JSONL file containing your training data.
- Note: Obtain this ID by uploading your dataset with the Files API and storing the file_id.
hyperparameters
- Description: A dictionary specifying the fine-tuning hyperparameters.
-
Key Fields:
- batch_size: Number of examples per batch (auto by default).
- learning_rate_multiplier: Scale factor for the learning rate (auto by default).
- n_epochs: Number of epochs (passes through the entire dataset).
suffix
- Description: A custom string (up to 18 characters) appended to the fine-tuned model name.
seed
- Description: Integer for reproducibility.
- Usage: Ensures the same randomization and consistent training results across runs.
validation_file
- Description: The file ID of a JSONL file containing your validation set.
- Optional: But recommended for tracking overfitting and ensuring a well-generalized model.
integrations
- Description: A list of integrations (e.g., Weights & Biases) you want enabled for the job.
- Fields: Typically includes type and integration-specific configurations.
client.fine_tuning.job.create( model="gpt-3.5-turbo", training_file="train_id", hyperparameters={ "n_epochs": 1 }, validation_file="val_id" )
Managing Fine-Tuning Jobs
Retrieves up to 10 fine-tuning jobs.
client.fine_tuning.jobs.list(limit=10)
Retrieve a Specific Job
client.fine_tuning.retrieve("job_id")
List Events for a Job
client.fine_tuning.list_events( fine_tuning_job_id="xxxx", limit=5 )
Summary
Model Selection: Choose a suitable GPT model to fine-tune.
Data Preparation: Upload JSONL files and note their IDs.
Hyperparameters: Tune batch size, learning rate, and epochs for optimal performance.
Monitoring: Use validation files, job retrieval, and event logging to ensure your model trains effectively.
Reproducibility: Set a seed if consistent results are important for your workflow.
By following these steps, you’ll have a clear path to submitting and managing your fine-tuning jobs in OpenAI, ensuring your model is trained precisely on your custom data.
The above is the detailed content of Submitting a Fine-Tuning Job: Organising the Workforce. For more information, please follow other related articles on the PHP Chinese website!
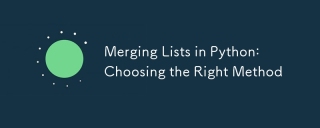
TomergelistsinPython,youcanusethe operator,extendmethod,listcomprehension,oritertools.chain,eachwithspecificadvantages:1)The operatorissimplebutlessefficientforlargelists;2)extendismemory-efficientbutmodifiestheoriginallist;3)listcomprehensionoffersf
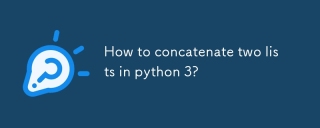
In Python 3, two lists can be connected through a variety of methods: 1) Use operator, which is suitable for small lists, but is inefficient for large lists; 2) Use extend method, which is suitable for large lists, with high memory efficiency, but will modify the original list; 3) Use * operator, which is suitable for merging multiple lists, without modifying the original list; 4) Use itertools.chain, which is suitable for large data sets, with high memory efficiency.
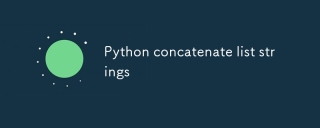
Using the join() method is the most efficient way to connect strings from lists in Python. 1) Use the join() method to be efficient and easy to read. 2) The cycle uses operators inefficiently for large lists. 3) The combination of list comprehension and join() is suitable for scenarios that require conversion. 4) The reduce() method is suitable for other types of reductions, but is inefficient for string concatenation. The complete sentence ends.
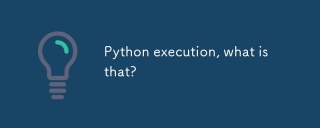
PythonexecutionistheprocessoftransformingPythoncodeintoexecutableinstructions.1)Theinterpreterreadsthecode,convertingitintobytecode,whichthePythonVirtualMachine(PVM)executes.2)TheGlobalInterpreterLock(GIL)managesthreadexecution,potentiallylimitingmul
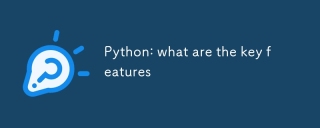
Key features of Python include: 1. The syntax is concise and easy to understand, suitable for beginners; 2. Dynamic type system, improving development speed; 3. Rich standard library, supporting multiple tasks; 4. Strong community and ecosystem, providing extensive support; 5. Interpretation, suitable for scripting and rapid prototyping; 6. Multi-paradigm support, suitable for various programming styles.
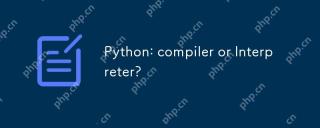
Python is an interpreted language, but it also includes the compilation process. 1) Python code is first compiled into bytecode. 2) Bytecode is interpreted and executed by Python virtual machine. 3) This hybrid mechanism makes Python both flexible and efficient, but not as fast as a fully compiled language.
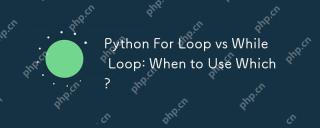
Useaforloopwheniteratingoverasequenceorforaspecificnumberoftimes;useawhileloopwhencontinuinguntilaconditionismet.Forloopsareidealforknownsequences,whilewhileloopssuitsituationswithundeterminediterations.
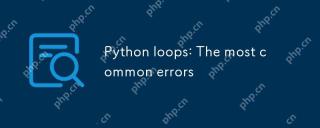
Pythonloopscanleadtoerrorslikeinfiniteloops,modifyinglistsduringiteration,off-by-oneerrors,zero-indexingissues,andnestedloopinefficiencies.Toavoidthese:1)Use'i


Hot AI Tools

Undresser.AI Undress
AI-powered app for creating realistic nude photos

AI Clothes Remover
Online AI tool for removing clothes from photos.

Undress AI Tool
Undress images for free

Clothoff.io
AI clothes remover

Video Face Swap
Swap faces in any video effortlessly with our completely free AI face swap tool!

Hot Article

Hot Tools
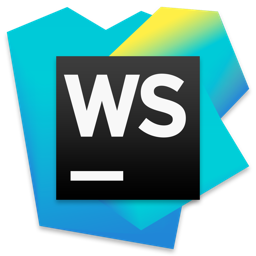
WebStorm Mac version
Useful JavaScript development tools

SublimeText3 Linux new version
SublimeText3 Linux latest version
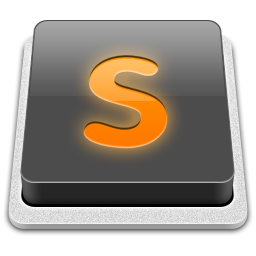
SublimeText3 Mac version
God-level code editing software (SublimeText3)

Atom editor mac version download
The most popular open source editor
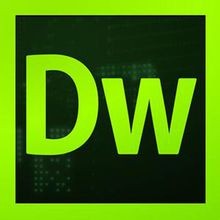
Dreamweaver CS6
Visual web development tools
