When Should I (Not) Use pandas.apply() in My Code?
Introduction
pandas.apply() is a powerful tool that allows users to apply a function over the rows or columns of a DataFrame or Series. However, it has been known to be slower than other methods, leading to the question of when it should be used and avoided. This article examines the reasons behind apply()'s performance issues and provides practical guidelines on how to eliminate its use.
Why is apply() Slow?
apply() calculates the result for each row or column individually, which can be inefficient when vectorized operations are available. Additionally, apply() incurs overhead by handling alignment, handling complex arguments, and allocating memory.
When to Avoid apply()
Use vectorized alternatives whenever possible. Vectorized operations, such as those provided by NumPy or pandas' own vectorized functions, operate on entire arrays at once, resulting in significant performance gains.
Avoid apply() for string manipulations. Pandas provides optimized string functions that are vectorized and faster than string-based apply() calls.
Use list comprehensions for column explosions. Exploding columns of lists using apply() is inefficient. Prefer using list comprehensions or converting the column to a list and passing it to pd.DataFrame().
When to Use apply()
Functions not vectorized for DataFrames. There are functions that are vectorized for Series but not DataFrames. For example, pd.to_datetime() can be used with apply() to convert multiple columns to datetime.
Complex functions requiring row-wise processing. In certain cases, it may be necessary to apply a complex function that requires row-wise processing. However, this should be avoided if possible.
GroupBy.apply() Considerations
Use vectorized GroupBy operations. GroupBy operations have vectorized alternatives that can be more efficient.
Avoid apply() for chained transformations. Chaining multiple operations within GroupBy.apply() can result in unnecessary iterations. Use separate GroupBy calls if possible.
Other Caveats
apply() operates on the first row twice. It needs to determine if the function has side effects, which can impact performance.
Memory consumption. apply() consumes a substantial amount of memory, making it unsuitable for memory-bound applications.
Conclusion
pandas.apply() is an accessible function, but its performance limitations should be carefully considered. To avoid performance issues, it is essential to identify vectorized alternatives, explore efficient options for string manipulations, and use apply() judiciously when no other option is available. By understanding the reasons behind its inefficiency, developers can write efficient and maintainable pandas code.
The above is the detailed content of When Should I Use (and When Should I Avoid) pandas.apply()?. For more information, please follow other related articles on the PHP Chinese website!
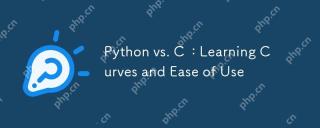
Python is easier to learn and use, while C is more powerful but complex. 1. Python syntax is concise and suitable for beginners. Dynamic typing and automatic memory management make it easy to use, but may cause runtime errors. 2.C provides low-level control and advanced features, suitable for high-performance applications, but has a high learning threshold and requires manual memory and type safety management.
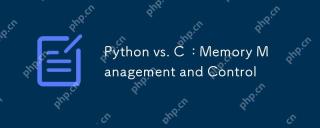
Python and C have significant differences in memory management and control. 1. Python uses automatic memory management, based on reference counting and garbage collection, simplifying the work of programmers. 2.C requires manual management of memory, providing more control but increasing complexity and error risk. Which language to choose should be based on project requirements and team technology stack.
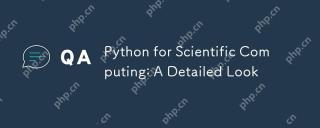
Python's applications in scientific computing include data analysis, machine learning, numerical simulation and visualization. 1.Numpy provides efficient multi-dimensional arrays and mathematical functions. 2. SciPy extends Numpy functionality and provides optimization and linear algebra tools. 3. Pandas is used for data processing and analysis. 4.Matplotlib is used to generate various graphs and visual results.
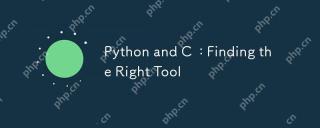
Whether to choose Python or C depends on project requirements: 1) Python is suitable for rapid development, data science, and scripting because of its concise syntax and rich libraries; 2) C is suitable for scenarios that require high performance and underlying control, such as system programming and game development, because of its compilation and manual memory management.
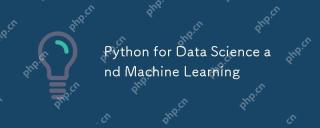
Python is widely used in data science and machine learning, mainly relying on its simplicity and a powerful library ecosystem. 1) Pandas is used for data processing and analysis, 2) Numpy provides efficient numerical calculations, and 3) Scikit-learn is used for machine learning model construction and optimization, these libraries make Python an ideal tool for data science and machine learning.
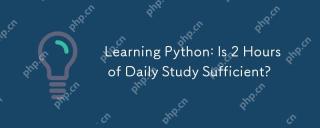
Is it enough to learn Python for two hours a day? It depends on your goals and learning methods. 1) Develop a clear learning plan, 2) Select appropriate learning resources and methods, 3) Practice and review and consolidate hands-on practice and review and consolidate, and you can gradually master the basic knowledge and advanced functions of Python during this period.
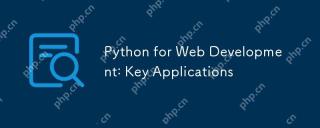
Key applications of Python in web development include the use of Django and Flask frameworks, API development, data analysis and visualization, machine learning and AI, and performance optimization. 1. Django and Flask framework: Django is suitable for rapid development of complex applications, and Flask is suitable for small or highly customized projects. 2. API development: Use Flask or DjangoRESTFramework to build RESTfulAPI. 3. Data analysis and visualization: Use Python to process data and display it through the web interface. 4. Machine Learning and AI: Python is used to build intelligent web applications. 5. Performance optimization: optimized through asynchronous programming, caching and code
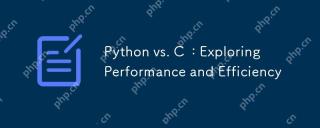
Python is better than C in development efficiency, but C is higher in execution performance. 1. Python's concise syntax and rich libraries improve development efficiency. 2.C's compilation-type characteristics and hardware control improve execution performance. When making a choice, you need to weigh the development speed and execution efficiency based on project needs.


Hot AI Tools

Undresser.AI Undress
AI-powered app for creating realistic nude photos

AI Clothes Remover
Online AI tool for removing clothes from photos.

Undress AI Tool
Undress images for free

Clothoff.io
AI clothes remover

AI Hentai Generator
Generate AI Hentai for free.

Hot Article

Hot Tools
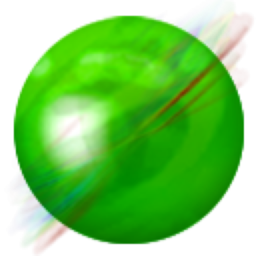
ZendStudio 13.5.1 Mac
Powerful PHP integrated development environment

Notepad++7.3.1
Easy-to-use and free code editor

mPDF
mPDF is a PHP library that can generate PDF files from UTF-8 encoded HTML. The original author, Ian Back, wrote mPDF to output PDF files "on the fly" from his website and handle different languages. It is slower than original scripts like HTML2FPDF and produces larger files when using Unicode fonts, but supports CSS styles etc. and has a lot of enhancements. Supports almost all languages, including RTL (Arabic and Hebrew) and CJK (Chinese, Japanese and Korean). Supports nested block-level elements (such as P, DIV),
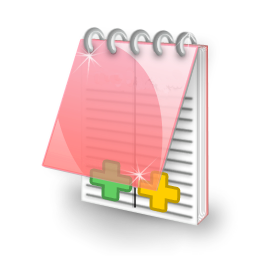
EditPlus Chinese cracked version
Small size, syntax highlighting, does not support code prompt function
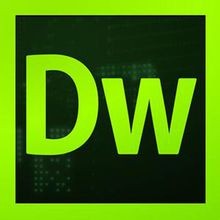
Dreamweaver CS6
Visual web development tools