When running email marketing campaigns, one of the biggest challenges is ensuring that your messages reach the inbox rather than the spam folder.
Apache SpamAssassin is a widely used tool for many email clients and email filtering tools to classify messages as spam. In this post, we’ll explore how to leverage SpamAssassin to validate if your email will be marked as spam and why it's marked so.
The logic will be packaged as an API and deployed online, so that it can be integrated into your workflow.
Why Apache SpamAssassin?
Apache SpamAssassin is an open-source spam detection platform maintained by the Apache Software Foundation. It uses a multitude of rules, Bayesian filtering, and network tests to assign a spam “score” to a given email. Generally, an email scoring 5 or above is at high risk of being flagged as spam.
Since that SpamAssassin’s scoring is transparent and well-documented, you can also use it to identify exactly which aspects of your email are causing high spam scores and improve your writing.
Getting Started with SpamAssassin
SpamAssassin is designed to run on Linux systems. You'll need a Linux OS or create a Docker VM to install and run it.
On Debian or Ubuntu systems, install SpamAssassin with:
apt-get update && apt-get install -y spamassassin sa-update
The sa-update command ensures that SpamAssassin’s rules are up-to-date.
Once installed, you can pipe an email message into SpamAssassin’s command-line tool. The output includes an annotated version of the email with spam scores and explains which rules are triggered.
A typical usage might look like this:
spamassassin -t results.txt
results.txt will then contain the processed email with SpamAssassin’s headers and scores.
Use FastAPI to Wrap SpamAssassin as an API
Next, let’s create a simple API that accepts two email fields: subject and html_body. It will pass the fields to SpamAssassin and return the validation result.
Example FastAPI Code
from fastapi import FastAPI from datetime import datetime, timezone from email.utils import format_datetime from pydantic import BaseModel import subprocess import re def extract_analysis_details(text): rules_section = re.search(r"Content analysis details:.*?(pts rule name.*?description.*?)\n\n", text, re.DOTALL) if not rules_section: return [] rules_text = rules_section.group(1) pattern = r"^\s*([-\d.]+)\s+(\S+)\s+(.+)$" rules = [] for line in rules_text.splitlines()[1:]: match = re.match(pattern, line) if match: score, rule, description = match.groups() rules.append({ "rule": rule, "score": float(score), "description": description.strip() }) return rules app = FastAPI() class Email(BaseModel): subject: str html_body: str @app.post("/spam_check") def spam_check(email: Email): # assemble the full email message = f"""From: example@example.com To: recipient@example.com Subject: {email.subject} Date: {format_datetime(datetime.now(timezone.utc))} Content-Type: text/html; charset="UTF-8" {email.html_body}""" # Run SpamAssassin and capture the output directly output = subprocess.run(["spamassassin", "-t"], input=message.encode('utf-8'), capture_output=True) output_str = output.stdout.decode('utf-8', errors='replace') details = extract_analysis_details(output_str) return {"result": details}
The response will contain the analysis details of SpamAssassin’s results.
Let's take this input as an example:
subject: Test Email html_body: <p>This is an <b>HTML</b> test email.</p>
The response would be like this:
[ { "rule": "MISSING_MID", "score": 0.1, "description": "Missing Message-Id: header" }, { "rule": "NO_RECEIVED", "score": -0.0, "description": "Informational: message has no Received headers" }, { "rule": "NO_RELAYS", "score": -0.0, "description": "Informational: message was not relayed via SMTP" }, { "rule": "HTML_MESSAGE", "score": 0.0, "description": "BODY: HTML included in message" }, { "rule": "MIME_HTML_ONLY", "score": 0.1, "description": "BODY: Message only has text/html MIME parts" }, { "rule": "MIME_HEADER_CTYPE_ONLY", "score": 0.1, "description": "'Content-Type' found without required MIME headers" } ]
Deploying the API Online
Running SpamAssassin requires a Linux environment with the software installed. Traditionally, you might need an EC2 instance or a DigitalOcean droplet to deploy, which can be costly and tedious, especially if your usage is low-volume.
As for serverless platforms, they often do not provide a straightforward way to run system packages like SpamAssassin.
Now with Leapcell, you can deploy any system packages like SpamAssassin, meanwhile keep the service serverless - you only pay for invocations, which is usually cheaper.
Deploying the API on Leapcell is very easy. You don't have to worry about how to set up a Linux environment or how to build a Dockerfile. Just select the Python image for deploying, and fill in the "Build Command" field properly.
Once deployed, you’ll have an endpoint you can call on-demand. Whenever your API is invoked, it will run SpamAssassin, score the email, and return the response.
The above is the detailed content of Build an API to Keep Your Marketing Emails Out of Spam. For more information, please follow other related articles on the PHP Chinese website!
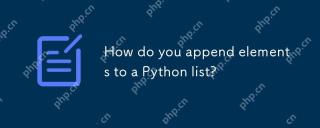
ToappendelementstoaPythonlist,usetheappend()methodforsingleelements,extend()formultipleelements,andinsert()forspecificpositions.1)Useappend()foraddingoneelementattheend.2)Useextend()toaddmultipleelementsefficiently.3)Useinsert()toaddanelementataspeci
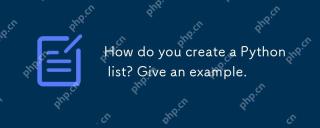
TocreateaPythonlist,usesquarebrackets[]andseparateitemswithcommas.1)Listsaredynamicandcanholdmixeddatatypes.2)Useappend(),remove(),andslicingformanipulation.3)Listcomprehensionsareefficientforcreatinglists.4)Becautiouswithlistreferences;usecopy()orsl
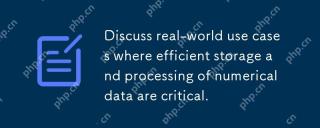
In the fields of finance, scientific research, medical care and AI, it is crucial to efficiently store and process numerical data. 1) In finance, using memory mapped files and NumPy libraries can significantly improve data processing speed. 2) In the field of scientific research, HDF5 files are optimized for data storage and retrieval. 3) In medical care, database optimization technologies such as indexing and partitioning improve data query performance. 4) In AI, data sharding and distributed training accelerate model training. System performance and scalability can be significantly improved by choosing the right tools and technologies and weighing trade-offs between storage and processing speeds.
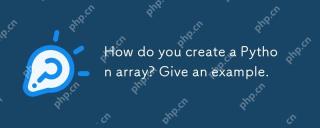
Pythonarraysarecreatedusingthearraymodule,notbuilt-inlikelists.1)Importthearraymodule.2)Specifythetypecode,e.g.,'i'forintegers.3)Initializewithvalues.Arraysofferbettermemoryefficiencyforhomogeneousdatabutlessflexibilitythanlists.
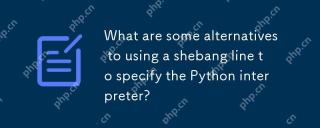
In addition to the shebang line, there are many ways to specify a Python interpreter: 1. Use python commands directly from the command line; 2. Use batch files or shell scripts; 3. Use build tools such as Make or CMake; 4. Use task runners such as Invoke. Each method has its advantages and disadvantages, and it is important to choose the method that suits the needs of the project.
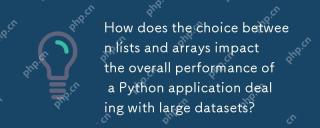
ForhandlinglargedatasetsinPython,useNumPyarraysforbetterperformance.1)NumPyarraysarememory-efficientandfasterfornumericaloperations.2)Avoidunnecessarytypeconversions.3)Leveragevectorizationforreducedtimecomplexity.4)Managememoryusagewithefficientdata
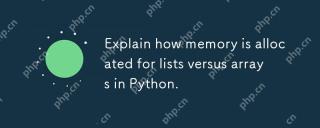
InPython,listsusedynamicmemoryallocationwithover-allocation,whileNumPyarraysallocatefixedmemory.1)Listsallocatemorememorythanneededinitially,resizingwhennecessary.2)NumPyarraysallocateexactmemoryforelements,offeringpredictableusagebutlessflexibility.
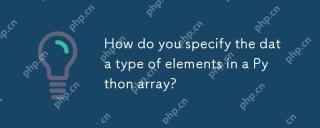
InPython, YouCansSpectHedatatYPeyFeLeMeReModelerErnSpAnT.1) UsenPyNeRnRump.1) UsenPyNeRp.DLOATP.PLOATM64, Formor PrecisconTrolatatypes.


Hot AI Tools

Undresser.AI Undress
AI-powered app for creating realistic nude photos

AI Clothes Remover
Online AI tool for removing clothes from photos.

Undress AI Tool
Undress images for free

Clothoff.io
AI clothes remover

Video Face Swap
Swap faces in any video effortlessly with our completely free AI face swap tool!

Hot Article

Hot Tools

DVWA
Damn Vulnerable Web App (DVWA) is a PHP/MySQL web application that is very vulnerable. Its main goals are to be an aid for security professionals to test their skills and tools in a legal environment, to help web developers better understand the process of securing web applications, and to help teachers/students teach/learn in a classroom environment Web application security. The goal of DVWA is to practice some of the most common web vulnerabilities through a simple and straightforward interface, with varying degrees of difficulty. Please note that this software

SAP NetWeaver Server Adapter for Eclipse
Integrate Eclipse with SAP NetWeaver application server.
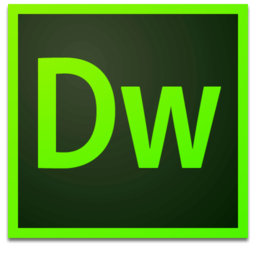
Dreamweaver Mac version
Visual web development tools

Atom editor mac version download
The most popular open source editor
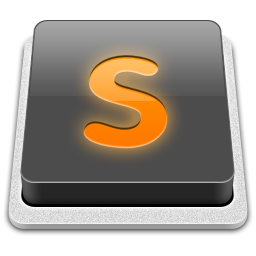
SublimeText3 Mac version
God-level code editing software (SublimeText3)
