


NumPy Benefits over Python Lists for Large-Scale Matrix Operations
In complex data analysis tasks involving large matrices, NumPy outperforms traditional Python lists due to its significant advantages. For instance, consider the task of creating a 100x100x100 cube array (approximately 1 million cells) and performing regressions on each x with y and z.
NumPy's arrays offer significant space efficiency compared to Python lists. In this case, a list of lists (as utilized in Python) would occupy approximately 20MB, while a NumPy array with single-precision floats would require only 4MB. This space saving becomes even more apparent with extremely large arrays, such as a 1000x1000x1000 cube array (1 billion cells). With NumPy, this array would fit into approximately 4GB on a 64-bit architecture, while Python would require around 12GB, making 32-bit architecture insufficient.
Apart from space efficiency, NumPy also provides faster data access for both reading and writing. This is because NumPy arrays use contiguous memory blocks, allowing the processor to cache the data and access it swiftly. In comparison, Python lists are a collection of objects with individual memory pointers, making access less efficient.
In summary, NumPy's compactness, speed, and scalability make it the preferred choice for handling large matrices and performing complex computations. Its advantages become more pronounced as the dataset grows, and for datasets like 1 billion cells, NumPy offers a clear performance and memory advantage.
The above is the detailed content of Why is NumPy Superior to Python Lists for Large-Scale Matrix Operations?. For more information, please follow other related articles on the PHP Chinese website!
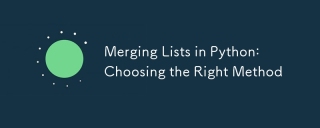
TomergelistsinPython,youcanusethe operator,extendmethod,listcomprehension,oritertools.chain,eachwithspecificadvantages:1)The operatorissimplebutlessefficientforlargelists;2)extendismemory-efficientbutmodifiestheoriginallist;3)listcomprehensionoffersf
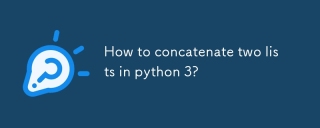
In Python 3, two lists can be connected through a variety of methods: 1) Use operator, which is suitable for small lists, but is inefficient for large lists; 2) Use extend method, which is suitable for large lists, with high memory efficiency, but will modify the original list; 3) Use * operator, which is suitable for merging multiple lists, without modifying the original list; 4) Use itertools.chain, which is suitable for large data sets, with high memory efficiency.
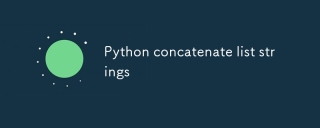
Using the join() method is the most efficient way to connect strings from lists in Python. 1) Use the join() method to be efficient and easy to read. 2) The cycle uses operators inefficiently for large lists. 3) The combination of list comprehension and join() is suitable for scenarios that require conversion. 4) The reduce() method is suitable for other types of reductions, but is inefficient for string concatenation. The complete sentence ends.
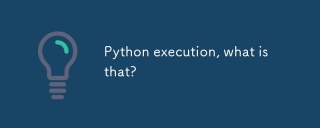
PythonexecutionistheprocessoftransformingPythoncodeintoexecutableinstructions.1)Theinterpreterreadsthecode,convertingitintobytecode,whichthePythonVirtualMachine(PVM)executes.2)TheGlobalInterpreterLock(GIL)managesthreadexecution,potentiallylimitingmul
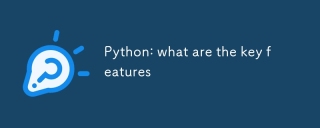
Key features of Python include: 1. The syntax is concise and easy to understand, suitable for beginners; 2. Dynamic type system, improving development speed; 3. Rich standard library, supporting multiple tasks; 4. Strong community and ecosystem, providing extensive support; 5. Interpretation, suitable for scripting and rapid prototyping; 6. Multi-paradigm support, suitable for various programming styles.
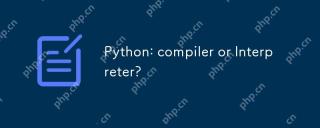
Python is an interpreted language, but it also includes the compilation process. 1) Python code is first compiled into bytecode. 2) Bytecode is interpreted and executed by Python virtual machine. 3) This hybrid mechanism makes Python both flexible and efficient, but not as fast as a fully compiled language.
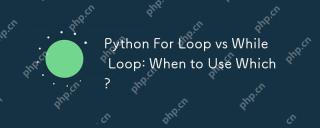
Useaforloopwheniteratingoverasequenceorforaspecificnumberoftimes;useawhileloopwhencontinuinguntilaconditionismet.Forloopsareidealforknownsequences,whilewhileloopssuitsituationswithundeterminediterations.
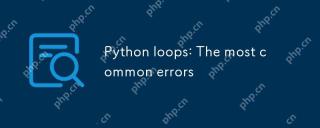
Pythonloopscanleadtoerrorslikeinfiniteloops,modifyinglistsduringiteration,off-by-oneerrors,zero-indexingissues,andnestedloopinefficiencies.Toavoidthese:1)Use'i


Hot AI Tools

Undresser.AI Undress
AI-powered app for creating realistic nude photos

AI Clothes Remover
Online AI tool for removing clothes from photos.

Undress AI Tool
Undress images for free

Clothoff.io
AI clothes remover

Video Face Swap
Swap faces in any video effortlessly with our completely free AI face swap tool!

Hot Article

Hot Tools

PhpStorm Mac version
The latest (2018.2.1) professional PHP integrated development tool
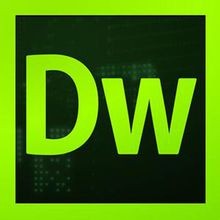
Dreamweaver CS6
Visual web development tools
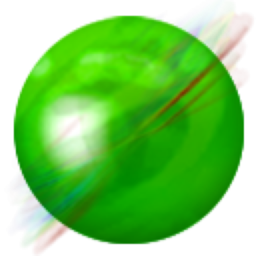
ZendStudio 13.5.1 Mac
Powerful PHP integrated development environment
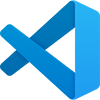
VSCode Windows 64-bit Download
A free and powerful IDE editor launched by Microsoft
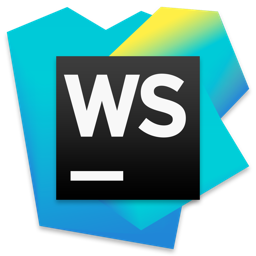
WebStorm Mac version
Useful JavaScript development tools
