


Advantages of NumPy Arrays over Python Lists for Large Matrices
When working with exceedingly large matrices, transitioning from Python lists to NumPy arrays offers significant advantages.
Compactness and Speed:
NumPy arrays excel in both compactness and speed compared to Python lists. Python lists, particularly those containing sublists (as in a cube array), occupy considerable memory due to the additional overhead of storing pointers to each sublist. Conversely, NumPy arrays store uniform data types, minimizing memory usage and providing faster access and manipulation.
Memory Efficiency and Scalability:
As the size of your datasets increases, the memory efficiency of NumPy arrays becomes increasingly apparent. For instance, a 100x100x100 matrix using single-precision floats would occupy approximately 4 MB using NumPy, whereas a Python list representation would require a minimum of 20 MB. With a billion-cell data cube (1000 series), NumPy would require about 4 GB of memory, while Python lists would demand 12 GB or more.
Underlying Architecture:
The difference between NumPy arrays and Python lists stems from their underlying architecture. Python lists rely on indirect addressing, with each element containing a pointer to the actual data. NumPy arrays, however, store data directly, minimizing overhead and optimizing performance.
Practical Applications:
In your specific case, with a 1 million-cell data cube, NumPy offers tangible benefits in compactness and performance. However, as your dataset grows to a billion cells, the memory efficiency advantage of NumPy becomes indispensable. Not only would it reduce memory requirements by a factor of three, but it would also enable the processing of such a large dataset on machines with limited RAM.
The above is the detailed content of Why Choose NumPy Arrays over Python Lists for Large Matrix Operations?. For more information, please follow other related articles on the PHP Chinese website!
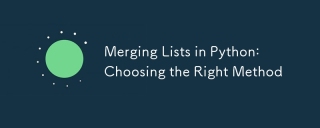
TomergelistsinPython,youcanusethe operator,extendmethod,listcomprehension,oritertools.chain,eachwithspecificadvantages:1)The operatorissimplebutlessefficientforlargelists;2)extendismemory-efficientbutmodifiestheoriginallist;3)listcomprehensionoffersf
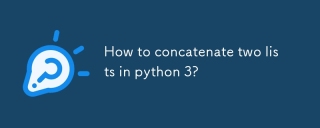
In Python 3, two lists can be connected through a variety of methods: 1) Use operator, which is suitable for small lists, but is inefficient for large lists; 2) Use extend method, which is suitable for large lists, with high memory efficiency, but will modify the original list; 3) Use * operator, which is suitable for merging multiple lists, without modifying the original list; 4) Use itertools.chain, which is suitable for large data sets, with high memory efficiency.
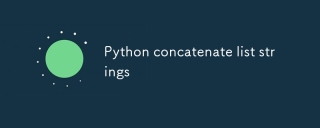
Using the join() method is the most efficient way to connect strings from lists in Python. 1) Use the join() method to be efficient and easy to read. 2) The cycle uses operators inefficiently for large lists. 3) The combination of list comprehension and join() is suitable for scenarios that require conversion. 4) The reduce() method is suitable for other types of reductions, but is inefficient for string concatenation. The complete sentence ends.
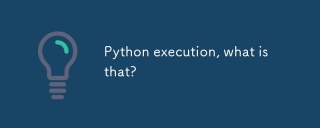
PythonexecutionistheprocessoftransformingPythoncodeintoexecutableinstructions.1)Theinterpreterreadsthecode,convertingitintobytecode,whichthePythonVirtualMachine(PVM)executes.2)TheGlobalInterpreterLock(GIL)managesthreadexecution,potentiallylimitingmul
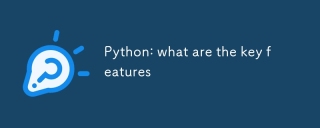
Key features of Python include: 1. The syntax is concise and easy to understand, suitable for beginners; 2. Dynamic type system, improving development speed; 3. Rich standard library, supporting multiple tasks; 4. Strong community and ecosystem, providing extensive support; 5. Interpretation, suitable for scripting and rapid prototyping; 6. Multi-paradigm support, suitable for various programming styles.
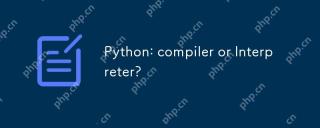
Python is an interpreted language, but it also includes the compilation process. 1) Python code is first compiled into bytecode. 2) Bytecode is interpreted and executed by Python virtual machine. 3) This hybrid mechanism makes Python both flexible and efficient, but not as fast as a fully compiled language.
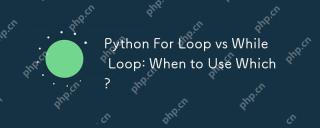
Useaforloopwheniteratingoverasequenceorforaspecificnumberoftimes;useawhileloopwhencontinuinguntilaconditionismet.Forloopsareidealforknownsequences,whilewhileloopssuitsituationswithundeterminediterations.
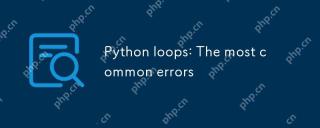
Pythonloopscanleadtoerrorslikeinfiniteloops,modifyinglistsduringiteration,off-by-oneerrors,zero-indexingissues,andnestedloopinefficiencies.Toavoidthese:1)Use'i


Hot AI Tools

Undresser.AI Undress
AI-powered app for creating realistic nude photos

AI Clothes Remover
Online AI tool for removing clothes from photos.

Undress AI Tool
Undress images for free

Clothoff.io
AI clothes remover

Video Face Swap
Swap faces in any video effortlessly with our completely free AI face swap tool!

Hot Article

Hot Tools
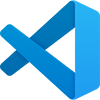
VSCode Windows 64-bit Download
A free and powerful IDE editor launched by Microsoft

Notepad++7.3.1
Easy-to-use and free code editor

SAP NetWeaver Server Adapter for Eclipse
Integrate Eclipse with SAP NetWeaver application server.
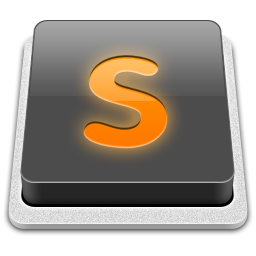
SublimeText3 Mac version
God-level code editing software (SublimeText3)
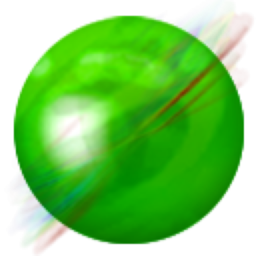
ZendStudio 13.5.1 Mac
Powerful PHP integrated development environment
