GitHub: https://github.com/chatsapi/ChatsAPI
Library: https://pypi.org/project/chatsapi/
Artificial Intelligence has transformed industries, but deploying it effectively remains a daunting challenge. Complex frameworks, slow response times, and steep learning curves create barriers for businesses and developers alike. Enter ChatsAPI — a groundbreaking, high-performance AI agent framework designed to deliver unmatched speed, flexibility, and simplicity.
In this article, we’ll uncover what makes ChatsAPI unique, why it’s a game-changer, and how it empowers developers to build intelligent systems with unparalleled ease and efficiency.
What Makes ChatsAPI Unique?
ChatsAPI is not just another AI framework; it’s a revolution in AI-driven interactions. Here’s why:
- Unmatched Performance ChatsAPI leverages SBERT embeddings, HNSWlib, and BM25 Hybrid Search to deliver the fastest query-matching system ever built.
Speed: With sub-millisecond response times, ChatsAPI is the world’s fastest AI agent framework. Its HNSWlib-powered search ensures lightning-fast retrieval of routes and knowledge, even with large datasets.
Efficiency: The hybrid approach of SBERT and BM25 combines semantic understanding with traditional ranking systems, ensuring both speed and accuracy.
Seamless Integration with LLMs
ChatsAPI supports state-of-the-art Large Language Models (LLMs) like OpenAI, Gemini, LlamaAPI, and Ollama. It simplifies the complexity of integrating LLMs into your applications, allowing you to focus on building better experiences.Dynamic Route Matching
ChatsAPI uses natural language understanding (NLU) to dynamically match user queries to predefined routes with unparalleled precision.
Register routes effortlessly with decorators like @trigger.
Use parameter extraction with @extract to simplify input handling, no matter how complex your use case.
- Simplicity in Design We believe that power and simplicity can coexist. With ChatsAPI, developers can build robust AI-driven systems in minutes. No more wrestling with complicated setups or configurations.
The Advantages of ChatsAPI
High-Performance Query Handling
Traditional AI systems struggle with either speed or accuracy — ChatsAPI delivers both. Whether it’s finding the best match in a vast knowledge base or handling high volumes of queries, ChatsAPI excels.
Flexible Framework
ChatsAPI adapts to any use case, whether you’re building:
- Customer support chatbots.
- Intelligent search systems.
- AI-powered assistants for e-commerce, healthcare, or education.
Built for Developers
Designed by developers, for developers, ChatsAPI offers:
- Quick Start: Set up your environment, define routes, and go live in just a few steps.
- Customization: Tailor the behavior with decorators and fine-tune performance for your specific needs.
- Easy LLM Integration: Switch between supported LLMs like OpenAI or Gemini with minimal effort.
How Does ChatsAPI Work?
At its core, ChatsAPI operates through a three-step process:
- Register Routes: Use the @trigger decorator to define routes and associate them with your functions.
- Search and Match: ChatsAPI uses SBERT embeddings and BM25 Hybrid Search to match user inputs with the right routes dynamically.
- Extract Parameters: With the @extract decorator, ChatsAPI automatically extracts and validates parameters, making it easier to handle complex inputs.
The result? A system that’s fast, accurate, and ridiculously easy to use.
Use Cases
Customer Support
Automate customer interactions with blazing-fast query resolution. ChatsAPI ensures users get relevant answers instantly, improving satisfaction and reducing operational costs.Knowledge Base Search
Empower users to search vast knowledge bases with semantic understanding. The hybrid SBERT-BM25 approach ensures accurate, context-aware results.Conversational AI
Build conversational AI agents that understand and adapt to user inputs in real-time. ChatsAPI integrates seamlessly with top LLMs to deliver natural, engaging conversations.
Why Should You Care?
Other frameworks promise flexibility or performance — but none can deliver both like ChatsAPI. We’ve created a framework that’s:
- Faster than anything else in the market.
- Simpler to set up and use.
- Smarter, with its unique hybrid search engine that blends semantic and keyword-based approaches.
ChatsAPI empowers developers to unlock the full potential of AI, without the headaches of complexity or slow performance.
How to Get Started
Getting started with ChatsAPI is easy:
- Install the framework:
pip install chatsapi
- Define your routes:
from chatsapi import ChatsAPI chat = ChatsAPI() @chat.trigger("Hello") async def greet(input_text): return "Hi there!"
- Extract some data from the message
from chatsapi import ChatsAPI chat = ChatsAPI() @chat.trigger("Need help with account settings.") @chat.extract([ ("account_number", "Account number (a nine digit number)", int, None), ("holder_name", "Account holder's name (a person name)", str, None) ]) async def account_help(chat_message: str, extracted: dict): return {"message": chat_message, "extracted": extracted} Run your message (with no LLM) @app.post("/chat") async def message(request: RequestModel, response: Response): reply = await chat.run(request.message) return {"message": reply}
- Conversations (with LLM) — Full Example
import os from dotenv import load_dotenv from fastapi import FastAPI, Request, Response from pydantic import BaseModel from chatsapi.chatsapi import ChatsAPI # Load environment variables from .env file load_dotenv() app = FastAPI() # instantiate FastAPI or your web framework chat = ChatsAPI( # instantiate ChatsAPI llm_type="gemini", llm_model="models/gemini-pro", llm_api_key=os.getenv("GOOGLE_API_KEY"), ) # chat trigger - 1 @chat.trigger("Want to cancel a credit card.") @chat.extract([("card_number", "Credit card number (a 12 digit number)", str, None)]) async def cancel_credit_card(chat_message: str, extracted: dict): return {"message": chat_message, "extracted": extracted} # chat trigger - 2 @chat.trigger("Need help with account settings.") @chat.extract([ ("account_number", "Account number (a nine digit number)", int, None), ("holder_name", "Account holder's name (a person name)", str, None) ]) async def account_help(chat_message: str, extracted: dict): return {"message": chat_message, "extracted": extracted} # request model class RequestModel(BaseModel): message: str # chat conversation @app.post("/chat") async def message(request: RequestModel, response: Response, http_request: Request): session_id = http_request.cookies.get("session_id") reply = await chat.conversation(request.message, session_id) return {"message": f"{reply}"} # set chat session @app.post("/set-session") def set_session(response: Response): session_id = chat.set_session() response.set_cookie(key="session_id", value=session_id) return {"message": "Session set"} # end chat session @app.post("/end-session") def end_session(response: Response, http_request: Request): session_id = http_request.cookies.get("session_id") chat.end_session(session_id) response.delete_cookie("session_id") return {"message": "Session ended"}
- Routes adhering LLM queries — Single Query
await chat.query(request.message)
Benchmarks
Traditional LLM (API)-based methods typically take around four seconds per request. In contrast, ChatsAPI processes requests in under one second, often within milliseconds, without making any LLM API calls.
Performing a chat routing task within 472ms (no cache)
Performing a chat routing task within 21ms (after cache)
Performing a chat routing data extraction task within 862ms (no cache)
Demonstrating its conversational abilities with WhatsApp Cloud API
ChatsAPI — Feature Hierarchy
ChatsAPI is more than just a framework; it’s a paradigm shift in how we build and interact with AI systems. By combining speed, accuracy, and ease of use, ChatsAPI sets a new benchmark for AI agent frameworks.
Join the revolution today and see why ChatsAPI is transforming the AI landscape.
Ready to dive in? Get started with ChatsAPI now and experience the future of AI development.
The above is the detailed content of ChatsAPI — The World's Fastest AI Agent Framework. For more information, please follow other related articles on the PHP Chinese website!
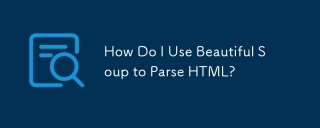
This article explains how to use Beautiful Soup, a Python library, to parse HTML. It details common methods like find(), find_all(), select(), and get_text() for data extraction, handling of diverse HTML structures and errors, and alternatives (Sel
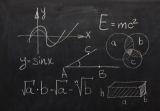
Python's statistics module provides powerful data statistical analysis capabilities to help us quickly understand the overall characteristics of data, such as biostatistics and business analysis. Instead of looking at data points one by one, just look at statistics such as mean or variance to discover trends and features in the original data that may be ignored, and compare large datasets more easily and effectively. This tutorial will explain how to calculate the mean and measure the degree of dispersion of the dataset. Unless otherwise stated, all functions in this module support the calculation of the mean() function instead of simply summing the average. Floating point numbers can also be used. import random import statistics from fracti
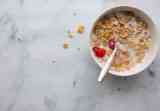
Serialization and deserialization of Python objects are key aspects of any non-trivial program. If you save something to a Python file, you do object serialization and deserialization if you read the configuration file, or if you respond to an HTTP request. In a sense, serialization and deserialization are the most boring things in the world. Who cares about all these formats and protocols? You want to persist or stream some Python objects and retrieve them in full at a later time. This is a great way to see the world on a conceptual level. However, on a practical level, the serialization scheme, format or protocol you choose may determine the speed, security, freedom of maintenance status, and other aspects of the program
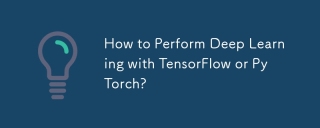
This article compares TensorFlow and PyTorch for deep learning. It details the steps involved: data preparation, model building, training, evaluation, and deployment. Key differences between the frameworks, particularly regarding computational grap
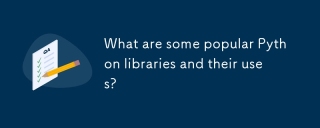
The article discusses popular Python libraries like NumPy, Pandas, Matplotlib, Scikit-learn, TensorFlow, Django, Flask, and Requests, detailing their uses in scientific computing, data analysis, visualization, machine learning, web development, and H
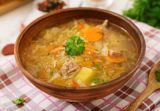
This tutorial builds upon the previous introduction to Beautiful Soup, focusing on DOM manipulation beyond simple tree navigation. We'll explore efficient search methods and techniques for modifying HTML structure. One common DOM search method is ex
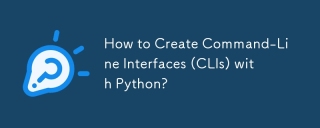
This article guides Python developers on building command-line interfaces (CLIs). It details using libraries like typer, click, and argparse, emphasizing input/output handling, and promoting user-friendly design patterns for improved CLI usability.
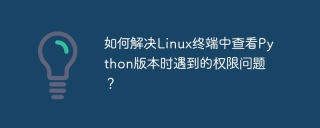
Solution to permission issues when viewing Python version in Linux terminal When you try to view Python version in Linux terminal, enter python...


Hot AI Tools

Undresser.AI Undress
AI-powered app for creating realistic nude photos

AI Clothes Remover
Online AI tool for removing clothes from photos.

Undress AI Tool
Undress images for free

Clothoff.io
AI clothes remover

AI Hentai Generator
Generate AI Hentai for free.

Hot Article

Hot Tools

Safe Exam Browser
Safe Exam Browser is a secure browser environment for taking online exams securely. This software turns any computer into a secure workstation. It controls access to any utility and prevents students from using unauthorized resources.

SecLists
SecLists is the ultimate security tester's companion. It is a collection of various types of lists that are frequently used during security assessments, all in one place. SecLists helps make security testing more efficient and productive by conveniently providing all the lists a security tester might need. List types include usernames, passwords, URLs, fuzzing payloads, sensitive data patterns, web shells, and more. The tester can simply pull this repository onto a new test machine and he will have access to every type of list he needs.
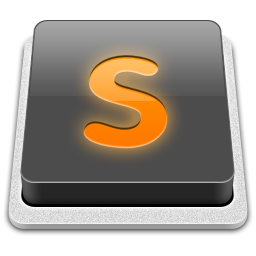
SublimeText3 Mac version
God-level code editing software (SublimeText3)
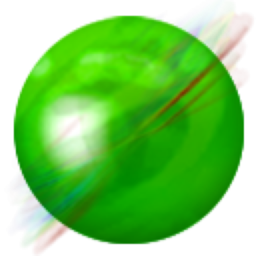
ZendStudio 13.5.1 Mac
Powerful PHP integrated development environment

SublimeText3 English version
Recommended: Win version, supports code prompts!
