


How Can I Efficiently Create an Empty DataFrame for Time-Series Data Before Filling It?
Conundrum: Creating an Empty Data Structure
You aspire to construct a DataFrame and subsequently fill it with time-series data. Initially, you envision an empty DataFrame furnished with specific columns and timestamps, all adorned with zeros or NaN values.
Current Approach: An Inelegant Solution
Your current code initializes a DataFrame with all-zero columns and timestamp rows before iterating through the data to calculate new values. While this approach serves its purpose, it feels cumbersome and suggests the existence of a more efficient solution.
Preferred Solution: Accumulating Data in a List
To optimize this process, it's prudent to steer clear of row-wise growth in the DataFrame. Instead, accumulate data into a list and then initialize a DataFrame once data collection is complete. Lists are more lightweight, consume less memory, and facilitate automatic dtypes inference and index assignment.
data = [] for row in some_function_that_yields_data(): data.append(row) df = pd.DataFrame(data)
Advantages of Accumulation in a List
- Enhanced Computational Efficiency: Appending to a list and creating a DataFrame in one go is significantly faster than iterative appends to an empty DataFrame.
- Memory Optimization: Lists occupy less memory and are easier to append and manipulate.
- Automatic Dtypes Inference: DataFrame constructor automatically infers dtypes based on the data added.
- Synchronized Index Creation: A RangeIndex is automatically generated for the resulting DataFrame.
Deprecated Methods to Avoid
Certain practices, prevalent among novice users, should be avoided due to their inefficiency and nuances:
- Iterative Append or Concat: This approach suffers from quadratic complexity and data type concerns.
- appending via loc: Appending using loc incurs the same memory reallocation issues as iterative append.
- Empty DataFrame of NaNs: Creating a DataFrame with NaN values results in object columns, which complicates data operations. It's better to allocate memory once in advance to avoid memory fragmentation.
The above is the detailed content of How Can I Efficiently Create an Empty DataFrame for Time-Series Data Before Filling It?. For more information, please follow other related articles on the PHP Chinese website!
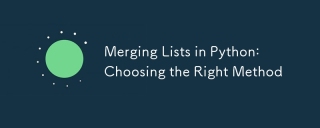
TomergelistsinPython,youcanusethe operator,extendmethod,listcomprehension,oritertools.chain,eachwithspecificadvantages:1)The operatorissimplebutlessefficientforlargelists;2)extendismemory-efficientbutmodifiestheoriginallist;3)listcomprehensionoffersf
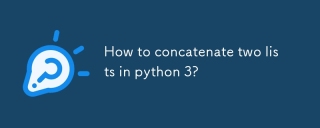
In Python 3, two lists can be connected through a variety of methods: 1) Use operator, which is suitable for small lists, but is inefficient for large lists; 2) Use extend method, which is suitable for large lists, with high memory efficiency, but will modify the original list; 3) Use * operator, which is suitable for merging multiple lists, without modifying the original list; 4) Use itertools.chain, which is suitable for large data sets, with high memory efficiency.
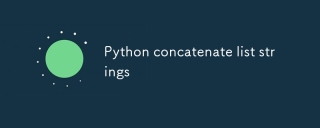
Using the join() method is the most efficient way to connect strings from lists in Python. 1) Use the join() method to be efficient and easy to read. 2) The cycle uses operators inefficiently for large lists. 3) The combination of list comprehension and join() is suitable for scenarios that require conversion. 4) The reduce() method is suitable for other types of reductions, but is inefficient for string concatenation. The complete sentence ends.
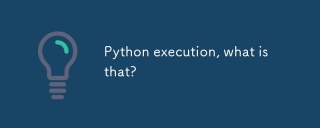
PythonexecutionistheprocessoftransformingPythoncodeintoexecutableinstructions.1)Theinterpreterreadsthecode,convertingitintobytecode,whichthePythonVirtualMachine(PVM)executes.2)TheGlobalInterpreterLock(GIL)managesthreadexecution,potentiallylimitingmul
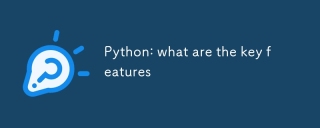
Key features of Python include: 1. The syntax is concise and easy to understand, suitable for beginners; 2. Dynamic type system, improving development speed; 3. Rich standard library, supporting multiple tasks; 4. Strong community and ecosystem, providing extensive support; 5. Interpretation, suitable for scripting and rapid prototyping; 6. Multi-paradigm support, suitable for various programming styles.
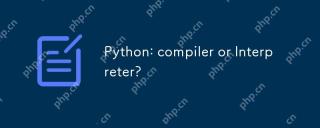
Python is an interpreted language, but it also includes the compilation process. 1) Python code is first compiled into bytecode. 2) Bytecode is interpreted and executed by Python virtual machine. 3) This hybrid mechanism makes Python both flexible and efficient, but not as fast as a fully compiled language.
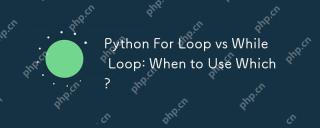
Useaforloopwheniteratingoverasequenceorforaspecificnumberoftimes;useawhileloopwhencontinuinguntilaconditionismet.Forloopsareidealforknownsequences,whilewhileloopssuitsituationswithundeterminediterations.
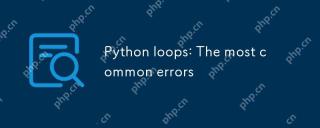
Pythonloopscanleadtoerrorslikeinfiniteloops,modifyinglistsduringiteration,off-by-oneerrors,zero-indexingissues,andnestedloopinefficiencies.Toavoidthese:1)Use'i


Hot AI Tools

Undresser.AI Undress
AI-powered app for creating realistic nude photos

AI Clothes Remover
Online AI tool for removing clothes from photos.

Undress AI Tool
Undress images for free

Clothoff.io
AI clothes remover

Video Face Swap
Swap faces in any video effortlessly with our completely free AI face swap tool!

Hot Article

Hot Tools

PhpStorm Mac version
The latest (2018.2.1) professional PHP integrated development tool
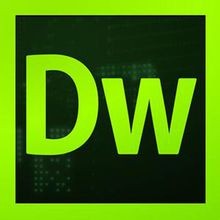
Dreamweaver CS6
Visual web development tools
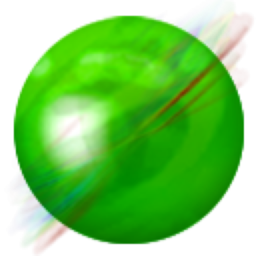
ZendStudio 13.5.1 Mac
Powerful PHP integrated development environment
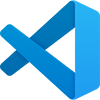
VSCode Windows 64-bit Download
A free and powerful IDE editor launched by Microsoft
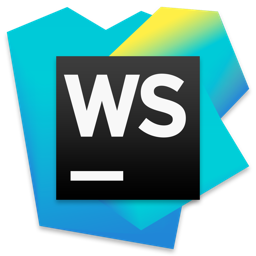
WebStorm Mac version
Useful JavaScript development tools
