


Recently, I was working on a multi-threaded implementation of a function to calculate the Poisson distribution (amath_pdist). The goal was to divide the workload across multiple threads to improve performance, especially for large arrays. However, instead of achieving the expected speedup, I noticed a significant slowdown as the size of the array increased.
After some investigation, I discovered the culprit: false sharing. In this post, I’ll explain what false sharing is, show the original code causing the problem, and share the fixes that led to a substantial performance improvement.
The Problem: False Sharing in Multi-threaded Code
False sharing happens when multiple threads work on different parts of a shared array, but their data resides in the same cache line. Cache lines are the smallest unit of data transferred between memory and the CPU cache (typically 64 bytes). If one thread writes to part of a cache line, it invalidates the line for other threads—even if they’re working on logically independent data. This unnecessary invalidation leads to significant performance degradation due to repeated reloading of cache lines.
Here’s a simplified version of my original code:
void *calculate_pdist_segment(void *data) { struct pdist_segment *segment = (struct pdist_segment *)data; size_t interval_a = segment->interval_a, interval_b = segment->interval_b; double lambda = segment->lambda; int *d = segment->data; for (size_t i = interval_a; i pdist[i] = pow(lambda, d[i]) * exp(-lambda) / tgamma(d[i] + 1); } return NULL; } double *amath_pdist(int *data, double lambda, size_t n_elements, size_t n_threads) { double *pdist = malloc(sizeof(double) * n_elements); pthread_t threads[n_threads]; struct pdist_segment segments[n_threads]; size_t step = n_elements / n_threads; for (size_t i = 0; i <hr> <h3> Where the Problem Occurs </h3> <p>In the above code:</p>
- The array pdist is shared among all threads.
- Each thread writes to a specific range of indices (interval_a to interval_b).
- At segment boundaries, adjacent indices may reside in the same cache line. For example, if pdist[249999] and pdist[250000] share a cache line, Thread 1 (working on pdist[249999]) and Thread 2 (working on pdist[250000]) invalidate each other’s cache lines.
This issue scaled poorly with larger arrays. While the boundary issue might seem small, the sheer number of iterations magnified the cost of cache invalidations, leading to seconds of unnecessary overhead.
The Solution: Align Memory to Cache Line Boundaries
To fix the problem, I used posix_memalign to ensure that the pdist array was aligned to 64-byte boundaries. This guarantees that threads operate on completely independent cache lines, eliminating false sharing.
Here’s the updated code:
double *amath_pdist(int *data, double lambda, size_t n_elements, size_t n_threads) { double *pdist; if (posix_memalign((void **)&pdist, 64, sizeof(double) * n_elements) != 0) { perror("Failed to allocate aligned memory"); return NULL; } pthread_t threads[n_threads]; struct pdist_segment segments[n_threads]; size_t step = n_elements / n_threads; for (size_t i = 0; i <hr> <h3> Why Does This Work? </h3> <ol> <li> <p><strong>Aligned Memory</strong>:</p> <ul> <li>Using posix_memalign, the array starts on a cache line boundary.</li> <li>Each thread’s assigned range aligns neatly with cache lines, preventing overlap.</li> </ul> </li> <li> <p><strong>No Cache Line Sharing</strong>:</p> <ul> <li>Threads operate on distinct cache lines, eliminating invalidations caused by false sharing.</li> </ul> </li> <li> <p><strong>Improved Cache Efficiency</strong>:</p> <ul> <li>Sequential memory access patterns align well with CPU prefetchers, further boosting performance.</li> </ul> </li> </ol> <hr> <h3> Results and Takeaways </h3> <p>After applying the fix, the runtime of the amath_pdist function dropped significantly. For a dataset I was testing, the wall clock time dropped from <strong>10.92 seconds to 0.06 seconds</strong>.</p> <h4> Key Lessons: </h4> <ol> <li> <strong>False sharing</strong> is a subtle yet critical issue in multi-threaded applications. Even small overlaps at segment boundaries can degrade performance.</li> <li> <strong>Memory alignment</strong> using posix_memalign is a simple and effective way to solve false sharing. Aligning memory to cache line boundaries ensures threads operate independently.</li> <li>Always analyze your code for cache-related issues when working with large arrays or parallel processing. Tools like perf or valgrind can help pinpoint bottlenecks.</li> </ol> <p>Thank you for reading!</p> <p>For anyone curious about the code, you can find it here</p>
The above is the detailed content of Understanding and Solving False Sharing in Multi-threaded Applications with an actual issue I had. For more information, please follow other related articles on the PHP Chinese website!
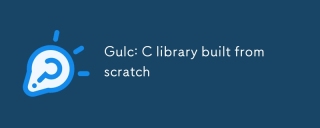
Gulc is a high-performance C library prioritizing minimal overhead, aggressive inlining, and compiler optimization. Ideal for performance-critical applications like high-frequency trading and embedded systems, its design emphasizes simplicity, modul
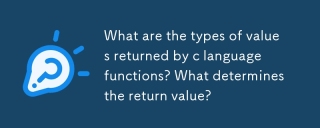
This article details C function return types, encompassing basic (int, float, char, etc.), derived (arrays, pointers, structs), and void types. The compiler determines the return type via the function declaration and the return statement, enforcing
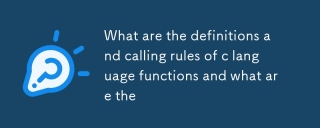
This article explains C function declaration vs. definition, argument passing (by value and by pointer), return values, and common pitfalls like memory leaks and type mismatches. It emphasizes the importance of declarations for modularity and provi
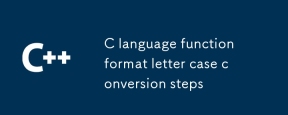
This article details C functions for string case conversion. It explains using toupper() and tolower() from ctype.h, iterating through strings, and handling null terminators. Common pitfalls like forgetting ctype.h and modifying string literals are
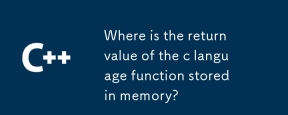
This article examines C function return value storage. Small return values are typically stored in registers for speed; larger values may use pointers to memory (stack or heap), impacting lifetime and requiring manual memory management. Directly acc
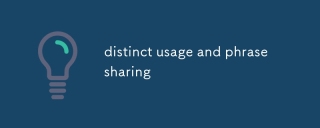
This article analyzes the multifaceted uses of the adjective "distinct," exploring its grammatical functions, common phrases (e.g., "distinct from," "distinctly different"), and nuanced application in formal vs. informal
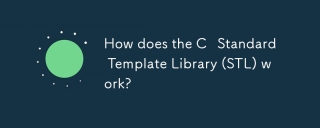
This article explains the C Standard Template Library (STL), focusing on its core components: containers, iterators, algorithms, and functors. It details how these interact to enable generic programming, improving code efficiency and readability t
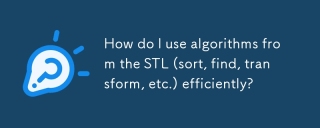
This article details efficient STL algorithm usage in C . It emphasizes data structure choice (vectors vs. lists), algorithm complexity analysis (e.g., std::sort vs. std::partial_sort), iterator usage, and parallel execution. Common pitfalls like


Hot AI Tools

Undresser.AI Undress
AI-powered app for creating realistic nude photos

AI Clothes Remover
Online AI tool for removing clothes from photos.

Undress AI Tool
Undress images for free

Clothoff.io
AI clothes remover

AI Hentai Generator
Generate AI Hentai for free.

Hot Article

Hot Tools

Zend Studio 13.0.1
Powerful PHP integrated development environment

Atom editor mac version download
The most popular open source editor
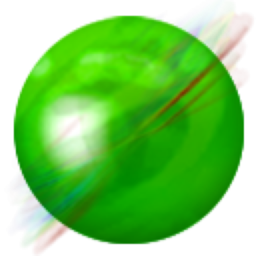
ZendStudio 13.5.1 Mac
Powerful PHP integrated development environment
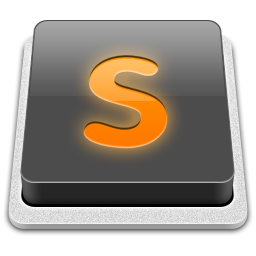
SublimeText3 Mac version
God-level code editing software (SublimeText3)
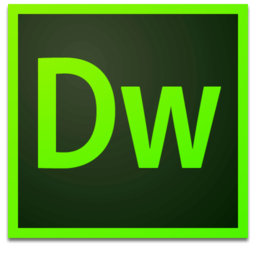
Dreamweaver Mac version
Visual web development tools
