Integrating Stanford Parser into NLTK with Python
Can Stanford Parser be leveraged within NLTK?
Yes, it is possible to utilize Stanford Parser within the NLTK framework using Python. The following Python code snippet demonstrates how to achieve this:
import os from nltk.parse import stanford # Specify paths to Stanford Parser and models os.environ['STANFORD_PARSER'] = '/path/to/standford/jars' os.environ['STANFORD_MODELS'] = '/path/to/standford/jars' # Initialize the Stanford Parser parser = stanford.StanfordParser(model_path="/location/of/the/englishPCFG.ser.gz") # Parse a list of sample sentences sentences = parser.raw_parse_sents(("Hello, My name is Melroy.", "What is your name?")) print sentences # Visualize the dependency tree for line in sentences: for sentence in line: sentence.draw()
This example showcases the parsed dependency trees for the provided sentences:
[Tree('ROOT', [Tree('S', [Tree('INTJ', [Tree('UH', ['Hello'])]), Tree(',', [',']), Tree('NP', [Tree('PRP$', ['My']), Tree('NN', ['name'])]), Tree('VP', [Tree('VBZ', ['is']), Tree('ADJP', [Tree('JJ', ['Melroy'])])]), Tree('.', ['.'])])]), Tree('ROOT', [Tree('SBARQ', [Tree('WHNP', [Tree('WP', ['What'])]), Tree('SQ', [Tree('VBZ', ['is']), Tree('NP', [Tree('PRP$', ['your']), Tree('NN', ['name'])])]), Tree('.', ['?'])])])}
Key Notes:
- In this example, both the parser and model jars reside in the same directory.
- The Stanford Parser's filename is stanford-parser.jar.
- The Stanford model's filename is stanford-parser-x.x.x-models.jar.
- The englishPCFG.ser.gz file is located within the models.jar file and needs to be extracted for use.
- Java JRE 1.8 (Java Development Kit 8) is required.
Installation Instructions:
Using NLTK v3 Installer:
- Download and install NLTK v3.
- Use the NLTK downloader:
import nltk nltk.download()
Manual Installation:
- Download and install NLTK v3.
- Download the latest Stanford Parser version.
- Extract the stanford-parser-3.x.x-models.jar and stanford-parser.jar files.
- Place these files in a designated 'jars' folder and set the STANFORD_PARSER and STANFORD_MODELS environment variables to point to this folder.
- Extract the englishPCFG.ser.gz file from the models.jar file and note its location.
- Create a StanfordParser instance using the specified model path.
The above is the detailed content of How Can I Integrate Stanford Parser with NLTK in Python?. For more information, please follow other related articles on the PHP Chinese website!
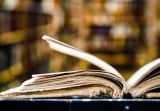
This tutorial demonstrates how to use Python to process the statistical concept of Zipf's law and demonstrates the efficiency of Python's reading and sorting large text files when processing the law. You may be wondering what the term Zipf distribution means. To understand this term, we first need to define Zipf's law. Don't worry, I'll try to simplify the instructions. Zipf's Law Zipf's law simply means: in a large natural language corpus, the most frequently occurring words appear about twice as frequently as the second frequent words, three times as the third frequent words, four times as the fourth frequent words, and so on. Let's look at an example. If you look at the Brown corpus in American English, you will notice that the most frequent word is "th
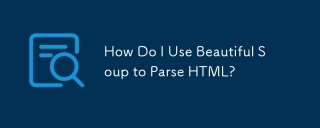
This article explains how to use Beautiful Soup, a Python library, to parse HTML. It details common methods like find(), find_all(), select(), and get_text() for data extraction, handling of diverse HTML structures and errors, and alternatives (Sel
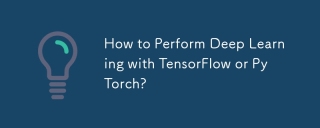
This article compares TensorFlow and PyTorch for deep learning. It details the steps involved: data preparation, model building, training, evaluation, and deployment. Key differences between the frameworks, particularly regarding computational grap
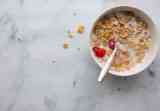
Serialization and deserialization of Python objects are key aspects of any non-trivial program. If you save something to a Python file, you do object serialization and deserialization if you read the configuration file, or if you respond to an HTTP request. In a sense, serialization and deserialization are the most boring things in the world. Who cares about all these formats and protocols? You want to persist or stream some Python objects and retrieve them in full at a later time. This is a great way to see the world on a conceptual level. However, on a practical level, the serialization scheme, format or protocol you choose may determine the speed, security, freedom of maintenance status, and other aspects of the program
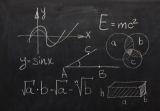
Python's statistics module provides powerful data statistical analysis capabilities to help us quickly understand the overall characteristics of data, such as biostatistics and business analysis. Instead of looking at data points one by one, just look at statistics such as mean or variance to discover trends and features in the original data that may be ignored, and compare large datasets more easily and effectively. This tutorial will explain how to calculate the mean and measure the degree of dispersion of the dataset. Unless otherwise stated, all functions in this module support the calculation of the mean() function instead of simply summing the average. Floating point numbers can also be used. import random import statistics from fracti
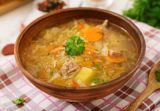
This tutorial builds upon the previous introduction to Beautiful Soup, focusing on DOM manipulation beyond simple tree navigation. We'll explore efficient search methods and techniques for modifying HTML structure. One common DOM search method is ex
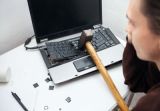
In this tutorial you'll learn how to handle error conditions in Python from a whole system point of view. Error handling is a critical aspect of design, and it crosses from the lowest levels (sometimes the hardware) all the way to the end users. If y
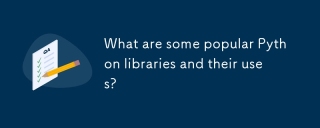
The article discusses popular Python libraries like NumPy, Pandas, Matplotlib, Scikit-learn, TensorFlow, Django, Flask, and Requests, detailing their uses in scientific computing, data analysis, visualization, machine learning, web development, and H


Hot AI Tools

Undresser.AI Undress
AI-powered app for creating realistic nude photos

AI Clothes Remover
Online AI tool for removing clothes from photos.

Undress AI Tool
Undress images for free

Clothoff.io
AI clothes remover

AI Hentai Generator
Generate AI Hentai for free.

Hot Article

Hot Tools

Atom editor mac version download
The most popular open source editor

mPDF
mPDF is a PHP library that can generate PDF files from UTF-8 encoded HTML. The original author, Ian Back, wrote mPDF to output PDF files "on the fly" from his website and handle different languages. It is slower than original scripts like HTML2FPDF and produces larger files when using Unicode fonts, but supports CSS styles etc. and has a lot of enhancements. Supports almost all languages, including RTL (Arabic and Hebrew) and CJK (Chinese, Japanese and Korean). Supports nested block-level elements (such as P, DIV),

SublimeText3 Linux new version
SublimeText3 Linux latest version
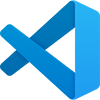
VSCode Windows 64-bit Download
A free and powerful IDE editor launched by Microsoft
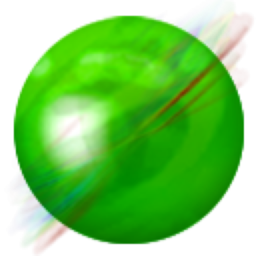
ZendStudio 13.5.1 Mac
Powerful PHP integrated development environment
