


Improving Red Color Detection with OpenCV using HSV Color Space
In OpenCV, the HSV color space offers an effective approach to detect specific colors, including red. However, due to the circular nature of the hue channel in HSV, red color can wrap around values near 180 degrees. This can pose challenges in detecting red objects accurately.
To address this issue, a more precise detection can be achieved by considering two ranges for the hue component: [0,10] and [170, 180]. By including both ranges, we ensure that the detection covers the entire red color spectrum.
The following Python code demonstrates this approach:
import cv2 # Read the input image image = cv2.imread("path_to_image") # Convert BGR to HSV color space hsv = cv2.cvtColor(image, cv2.COLOR_BGR2HSV) # Define HSV values for red color hue_min1 = 0 hue_max1 = 10 hue_min2 = 170 hue_max2 = 180 sat_min = 70 sat_max = 255 val_min = 50 val_max = 255 # Create masks for the two hue ranges mask1 = cv2.inRange(hsv, (hue_min1, sat_min, val_min), (hue_max1, sat_max, val_max)) mask2 = cv2.inRange(hsv, (hue_min2, sat_min, val_min), (hue_max2, sat_max, val_max)) # Combine the masks mask = mask1 | mask2 # Display the mask cv2.imshow("Mask", mask) cv2.waitKey(0) cv2.destroyAllWindows()
This code effectively detects the red rectangle in the image, as shown in the mask output.
Alternative Approach
An alternative method is to invert the BGR image and then convert it to HSV. This approach essentially searches for the complementary color, cyan (90 degrees on the hue channel), allowing you to detect red with a single range.
The following Python code demonstrates this technique:
import cv2 # Read the input image image = cv2.imread("path_to_image") # Invert the BGR image inverted_image = cv2.bitwise_not(image) # Convert inverted image to HSV color space hsv_inverted = cv2.cvtColor(inverted_image, cv2.COLOR_BGR2HSV) # Define HSV values for cyan color (inverted red) hue_min = 90 - 10 hue_max = 90 + 10 sat_min = 70 sat_max = 255 val_min = 50 val_max = 255 # Create a mask for the cyan color range mask = cv2.inRange(hsv_inverted, (hue_min, sat_min, val_min), (hue_max, sat_max, val_max)) # Display the mask cv2.imshow("Mask", mask) cv2.waitKey(0) cv2.destroyAllWindows()
Both approaches offer improved red color detection using OpenCV in HSV color space, providing more accurate results for image processing applications.
The above is the detailed content of How can I achieve more precise red color detection in OpenCV using HSV color space?. For more information, please follow other related articles on the PHP Chinese website!
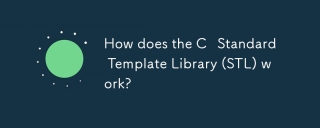
This article explains the C Standard Template Library (STL), focusing on its core components: containers, iterators, algorithms, and functors. It details how these interact to enable generic programming, improving code efficiency and readability t
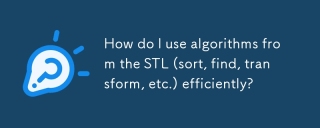
This article details efficient STL algorithm usage in C . It emphasizes data structure choice (vectors vs. lists), algorithm complexity analysis (e.g., std::sort vs. std::partial_sort), iterator usage, and parallel execution. Common pitfalls like
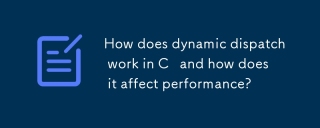
The article discusses dynamic dispatch in C , its performance costs, and optimization strategies. It highlights scenarios where dynamic dispatch impacts performance and compares it with static dispatch, emphasizing trade-offs between performance and
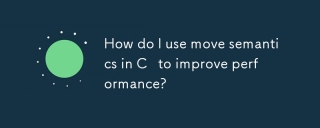
The article discusses using move semantics in C to enhance performance by avoiding unnecessary copying. It covers implementing move constructors and assignment operators, using std::move, and identifies key scenarios and pitfalls for effective appl
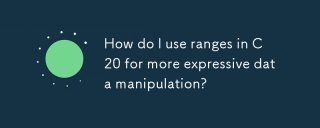
C 20 ranges enhance data manipulation with expressiveness, composability, and efficiency. They simplify complex transformations and integrate into existing codebases for better performance and maintainability.
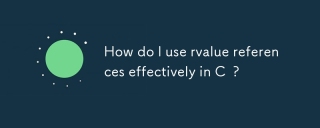
Article discusses effective use of rvalue references in C for move semantics, perfect forwarding, and resource management, highlighting best practices and performance improvements.(159 characters)
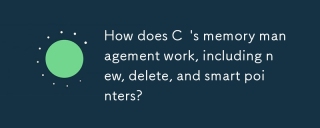
C memory management uses new, delete, and smart pointers. The article discusses manual vs. automated management and how smart pointers prevent memory leaks.
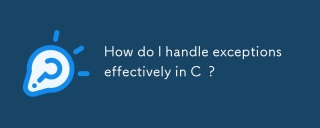
This article details effective exception handling in C , covering try, catch, and throw mechanics. It emphasizes best practices like RAII, avoiding unnecessary catch blocks, and logging exceptions for robust code. The article also addresses perf


Hot AI Tools

Undresser.AI Undress
AI-powered app for creating realistic nude photos

AI Clothes Remover
Online AI tool for removing clothes from photos.

Undress AI Tool
Undress images for free

Clothoff.io
AI clothes remover

AI Hentai Generator
Generate AI Hentai for free.

Hot Article

Hot Tools

SublimeText3 English version
Recommended: Win version, supports code prompts!

Safe Exam Browser
Safe Exam Browser is a secure browser environment for taking online exams securely. This software turns any computer into a secure workstation. It controls access to any utility and prevents students from using unauthorized resources.

Zend Studio 13.0.1
Powerful PHP integrated development environment

DVWA
Damn Vulnerable Web App (DVWA) is a PHP/MySQL web application that is very vulnerable. Its main goals are to be an aid for security professionals to test their skills and tools in a legal environment, to help web developers better understand the process of securing web applications, and to help teachers/students teach/learn in a classroom environment Web application security. The goal of DVWA is to practice some of the most common web vulnerabilities through a simple and straightforward interface, with varying degrees of difficulty. Please note that this software

mPDF
mPDF is a PHP library that can generate PDF files from UTF-8 encoded HTML. The original author, Ian Back, wrote mPDF to output PDF files "on the fly" from his website and handle different languages. It is slower than original scripts like HTML2FPDF and produces larger files when using Unicode fonts, but supports CSS styles etc. and has a lot of enhancements. Supports almost all languages, including RTL (Arabic and Hebrew) and CJK (Chinese, Japanese and Korean). Supports nested block-level elements (such as P, DIV),
