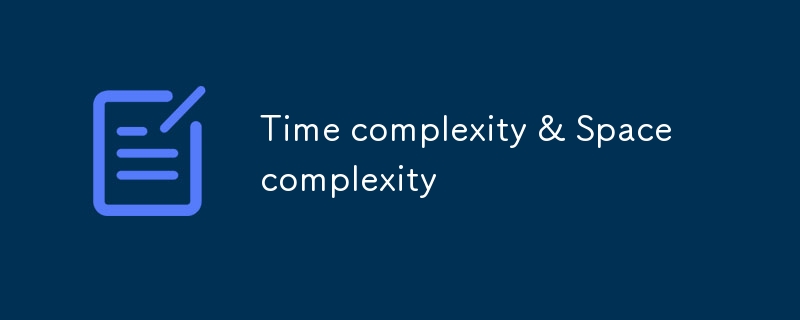
In general, time complexity and space complexity are ways to measure the efficiency of an algorithm based on how its resource usage scales with the size of its input. Let’s go over the basics and some common examples.
Time Complexity
Time complexity describes the amount of time an algorithm takes to complete based on the size of the input (often denoted as n).
-
Constant Time – O(1):
- The algorithm's execution time doesn’t change with the input size.
- Example: Accessing an element in an array by index, as in arr[5].
-
Logarithmic Time – O(log n):
- The algorithm's execution time grows logarithmically as input size increases, meaning it divides the problem in half with each step.
- Example: Binary search on a sorted array.
-
Linear Time – O(n):
- The algorithm's execution time grows linearly with input size.
- Example: Traversing an array of n elements once.
-
Linearithmic Time – O(n log n):
- Common in efficient sorting algorithms where each element is handled logarithmically, usually due to recursive division and linear merging or processing.
- Example: Merge sort, quicksort.
-
Quadratic Time – O(n²):
- Execution time grows proportionally to the square of the input size.
- Example: Nested loops, such as comparing each element in an array to every other element.
-
Cubic Time – O(n³):
- Execution time grows with the cube of the input size. Rare but can occur in algorithms with three nested loops.
- Example: Solving certain matrix operations using brute-force algorithms.
-
Exponential Time – O(2^n):
- Execution time doubles with each additional element in the input, typically from recursive algorithms that solve subproblems in all possible combinations.
- Example: The naive solution for the Fibonacci sequence, where each call leads to two more calls.
-
Factorial Time – O(n!):
- Execution time grows factorially with input size. Often from algorithms that involve generating all possible permutations or combinations.
- Example: Solving the traveling salesman problem with brute force.
Space Complexity
Space complexity measures the amount of memory an algorithm uses relative to the input size.
-
Constant Space – O(1):
- The algorithm uses a fixed amount of memory regardless of input size.
- Example: Storing a few variables, like integers or counters.
-
Logarithmic Space – O(log n):
- Memory usage grows logarithmically, often seen with recursive algorithms that halve the problem each step.
- Example: Recursive binary search (space complexity due to call stack).
-
Linear Space – O(n):
- Memory usage grows linearly with input size, common when creating an additional array or data structure to store input.
- Example: Creating a copy of an array of size n.
-
Quadratic Space – O(n²):
- Memory usage grows with the square of input size, such as when storing a 2D matrix of size n x n.
- Example: Storing an adjacency matrix for a graph with n nodes.
-
Exponential Space – O(2^n):
- Memory usage grows exponentially with the input size, often in recursive solutions that store data for each possible subset of the input.
- Example: Memoization in recursive algorithms with many overlapping subproblems.
Practical Examples
Analyzing Complexity
When analyzing code for time and space complexity:
-
Identify the loops: Nested loops usually increase complexity (e.g., one loop gives ( O(n) ); two nested loops give ( O(n^2) )).
-
Look for recursion: Recursive calls can lead to exponential time and space complexity, depending on the branching factor and depth of recursion.
-
Consider data structures: Using extra data structures like arrays, lists, or hash maps can affect space complexity.
General Tips
-
Time Complexity is about counting operations as a function of input size.
-
Space Complexity is about counting the amount of extra memory required.
By assessing these factors, you can estimate how efficiently an algorithm performs and how much memory it consumes based on input size.
The above is the detailed content of Time complexity & Space complexity. For more information, please follow other related articles on the PHP Chinese website!
Statement:The content of this article is voluntarily contributed by netizens, and the copyright belongs to the original author. This site does not assume corresponding legal responsibility. If you find any content suspected of plagiarism or infringement, please contact admin@php.cn