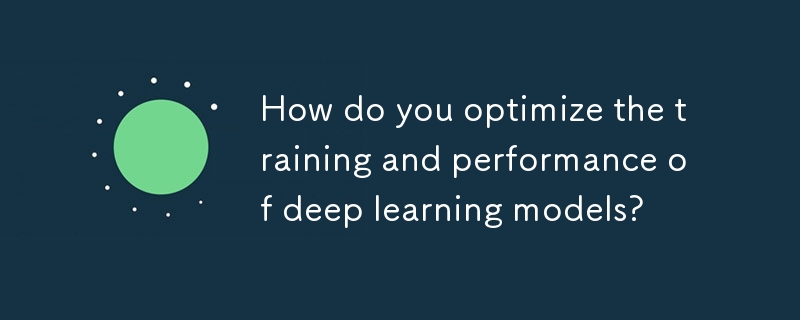
Understanding Keras Long Short-Term Memory (LSTM)
Before discussing the title question, let’s review the background.
Question:
- Selection criteria of loss function
- Advantages and disadvantages of weight update rules
- Training a good network Tips
- Principles of hyperparameter adjustment for deep learning models
Answer:
Loss function selection criteria:
- The choice of loss function depends on the nature of the training task and data.
- Commonly used loss functions include mean square error (MSE), cross entropy (CE), and KL divergence.
- For regression tasks, MSE is a common choice.
- For classification tasks, CE is widely used in binary and multi-classification problems.
- KL divergence measures the difference between two probability distributions.
Advantages and disadvantages of weight update rules:
- Gradient descent is the most commonly used weight update rule in deep learning.
- Advantages of gradient descent include ease of implementation and wide applicability.
- Disadvantages of gradient descent may include local optima and slow convergence.
- Other weight update rules include momentum, adaptive moment estimation (Adam), and RMSprop. These rules are designed to improve convergence speed and stability by employing different learning rate strategies.
Tips for training a good network:
-
Data preprocessing: Proper data preprocessing (e.g. normalization , standardization) can improve model performance and increase convergence speed.
-
Hyperparameter tuning: Hyperparameters (e.g. learning rate, batch size, network architecture) are tuned through techniques such as cross-validation or Bayesian optimization to optimize model performance.
-
Regularization: Regularization techniques such as L1, L2 regularization, and dropout help prevent overfitting and improve model generalization.
-
Data augmentation: Data augmentation techniques (such as image rotation, flipping, cropping) can generate more data samples, thereby improving the robustness and performance of the model.
Principles for hyperparameter adjustment of deep learning models:
-
Grid search: Grid search is the most effective way to adjust hyperparameters. Simple method that performs a comprehensive evaluation of a set of discrete values of hyperparameter values.
-
Random Search: Random search is more efficient than grid search because it randomly samples candidate values in the hyperparameter space for evaluation.
-
Bayesian Optimization: Bayesian optimization uses Bayes’ theorem to step-by-step guide the hyperparameter search process to maximize the objective function (such as model accuracy).
-
Reinforcement Learning: Reinforcement learning is an advanced hyperparameter tuning technique that uses a reward mechanism to optimize hyperparameter selection.
By understanding these principles and applying these techniques, you can optimize the training and performance of your deep learning models.
The above is the detailed content of How do you optimize the training and performance of deep learning models?. For more information, please follow other related articles on the PHP Chinese website!
Statement:The content of this article is voluntarily contributed by netizens, and the copyright belongs to the original author. This site does not assume corresponding legal responsibility. If you find any content suspected of plagiarism or infringement, please contact admin@php.cn