Error Metrics for Regression Algorithms
When we create a regression algorithm and want to know how efficient this model was, we use error metrics to obtain values that represent the error of our machine learning model. The metrics in this article are important when we want to measure the error of prediction models for numerical values (real, integers).
In this article we will cover the main error metrics for regression algorithms, performing the calculations manually in Python and measuring the error of the machine learning model on a dollar quote dataset.
Metrics Addressed
- SE — Sum of error
- ME — Mean error
- MAE — Mean Absolute error
- MPE — Mean Percentage error
- MAPAE — Mean Absolute Percentage error
Both metrics are a little similar, where we have metrics for average and percentage of error and metrics for average and absolute percentage of error, differentiated so only that one group obtains the real value of the difference and the other obtains the absolute value of the difference. difference. It is important to remember that in both metrics, the lower the value, the better our forecast.
SE — Sum of error
The SE metric is the simplest among all in this article, where its formula is:
SE = εR — P
Therefore, it is the sum of the difference between the real value (target variable of the model) and the predicted value. This metric has some negative points, such as not treating values as absolute, which will consequently result in a false value.
ME — Mean of error
The ME metric is a "complement" of the SE, where we basically have the difference that we will obtain an average of the SE given the number of elements:
ME = ε(R-P)/N
Unlike SE, we just divide the SE result by the number of elements. This metric, like SE, depends on scale, that is, we must use the same set of data and can compare with different forecasting models.
MAE — Mean absolute error
The MAE metric is the ME but considering only absolute (non-negative) values. When we are calculating the difference between actual and predicted, we may have negative results and this negative difference is applied to previous metrics. In this metric, we have to transform the difference into positive values and then take the average based on the number of elements.
MPE — Mean Percentage error
The MPE metric is the average error as a percentage of the sum of each difference. Here we have to take the percentage of the difference, add it and then divide it by the number of elements to obtain the average. Therefore, the difference between the actual value and the predicted value is made, divided by the actual value, multiplied by 100, we add up all this percentage and divide by the number of elements. This metric is independent of scale (%).
MAPAE — Mean Absolute Percentage error
The MAPAE metric is very similar to the previous metric, but the difference between the predicted x actual is made absolutely, that is, you calculate it with positive values. Therefore, this metric is the absolute difference in the percentage of error. This metric is also scale independent.
Using metrics in practice
Given an explanation of each metric, we will calculate both manually in Python based on a prediction from a dollar exchange rate machine learning model. Currently, most of the regression metrics exist in ready-made functions in the Sklearn package, however here we will calculate them manually for teaching purposes only.
We will use the RandomForest and Decision Tree algorithms only to compare results between the two models.
Data Analysis
In our dataset, we have a column of SaldoMercado and saldoMercado_2 which are information that influence the Value column (our dollar quote). As we can see, the MercadoMercado balance has a closer relationship to the quote than theMerado_2 balance. It is also possible to observe that we do not have missing values (infinite or Nan values) and that the balanceMercado_2 column has many non-absolute values.
Model Preparation
We prepare our values for the machine learning model by defining the predictor variables and the variable we want to predict. We use train_test_split to randomly divide the data into 30% for testing and 70% for training.
Finally, we initialize both algorithms (RandomForest and DecisionTree), fit the data and measure the score of both with the test data. We obtained a score of 83% for TreeRegressor and 90% for ForestRegressor, which in theory indicates that ForestRegressor performed better.
Results and Analysis
Given the partially observed performance of ForestRegressor, we created a dataset with the necessary data to apply the metrics. We perform the prediction on the test data and create a DataFrame with the actual and predicted values, including columns for difference and percentage.
We can observe that in relation to the real total of the dollar rate vs the rate that our model predicted:
- We had a total difference of R$578.00
- This represents 0.36% difference between predicted x Actual (not considered absolute values)
- In terms of the average error (ME) we had a low value, an average of R$0.009058
- For absolute average, this value increases a little, since we have negative values in our dataset
I reinforce that here we perform the calculation manually for teaching purposes. However, it is recommended to use the metrics functions from the Sklearn package due to better performance and low chance of error in the calculation.
The complete code is available on my GitHub: github.com/AirtonLira/artigo_metricasregressao
Author: Airton Lira Junior
LinkedIn: linkedin.com/in/airton-lira-junior-6b81a661/
The above is the detailed content of Metrics for regression algorithms. For more information, please follow other related articles on the PHP Chinese website!
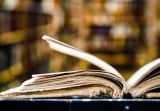
This tutorial demonstrates how to use Python to process the statistical concept of Zipf's law and demonstrates the efficiency of Python's reading and sorting large text files when processing the law. You may be wondering what the term Zipf distribution means. To understand this term, we first need to define Zipf's law. Don't worry, I'll try to simplify the instructions. Zipf's Law Zipf's law simply means: in a large natural language corpus, the most frequently occurring words appear about twice as frequently as the second frequent words, three times as the third frequent words, four times as the fourth frequent words, and so on. Let's look at an example. If you look at the Brown corpus in American English, you will notice that the most frequent word is "th
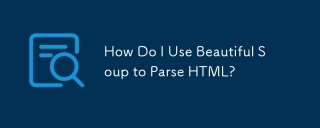
This article explains how to use Beautiful Soup, a Python library, to parse HTML. It details common methods like find(), find_all(), select(), and get_text() for data extraction, handling of diverse HTML structures and errors, and alternatives (Sel
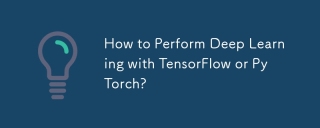
This article compares TensorFlow and PyTorch for deep learning. It details the steps involved: data preparation, model building, training, evaluation, and deployment. Key differences between the frameworks, particularly regarding computational grap
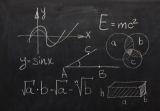
Python's statistics module provides powerful data statistical analysis capabilities to help us quickly understand the overall characteristics of data, such as biostatistics and business analysis. Instead of looking at data points one by one, just look at statistics such as mean or variance to discover trends and features in the original data that may be ignored, and compare large datasets more easily and effectively. This tutorial will explain how to calculate the mean and measure the degree of dispersion of the dataset. Unless otherwise stated, all functions in this module support the calculation of the mean() function instead of simply summing the average. Floating point numbers can also be used. import random import statistics from fracti
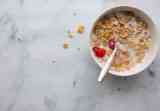
Serialization and deserialization of Python objects are key aspects of any non-trivial program. If you save something to a Python file, you do object serialization and deserialization if you read the configuration file, or if you respond to an HTTP request. In a sense, serialization and deserialization are the most boring things in the world. Who cares about all these formats and protocols? You want to persist or stream some Python objects and retrieve them in full at a later time. This is a great way to see the world on a conceptual level. However, on a practical level, the serialization scheme, format or protocol you choose may determine the speed, security, freedom of maintenance status, and other aspects of the program
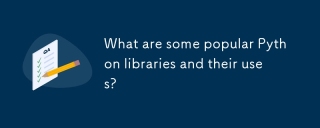
The article discusses popular Python libraries like NumPy, Pandas, Matplotlib, Scikit-learn, TensorFlow, Django, Flask, and Requests, detailing their uses in scientific computing, data analysis, visualization, machine learning, web development, and H
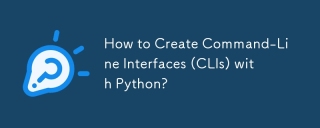
This article guides Python developers on building command-line interfaces (CLIs). It details using libraries like typer, click, and argparse, emphasizing input/output handling, and promoting user-friendly design patterns for improved CLI usability.
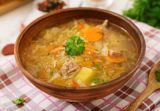
This tutorial builds upon the previous introduction to Beautiful Soup, focusing on DOM manipulation beyond simple tree navigation. We'll explore efficient search methods and techniques for modifying HTML structure. One common DOM search method is ex


Hot AI Tools

Undresser.AI Undress
AI-powered app for creating realistic nude photos

AI Clothes Remover
Online AI tool for removing clothes from photos.

Undress AI Tool
Undress images for free

Clothoff.io
AI clothes remover

AI Hentai Generator
Generate AI Hentai for free.

Hot Article

Hot Tools
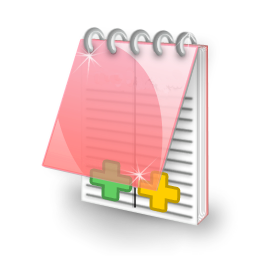
EditPlus Chinese cracked version
Small size, syntax highlighting, does not support code prompt function

SublimeText3 Chinese version
Chinese version, very easy to use

DVWA
Damn Vulnerable Web App (DVWA) is a PHP/MySQL web application that is very vulnerable. Its main goals are to be an aid for security professionals to test their skills and tools in a legal environment, to help web developers better understand the process of securing web applications, and to help teachers/students teach/learn in a classroom environment Web application security. The goal of DVWA is to practice some of the most common web vulnerabilities through a simple and straightforward interface, with varying degrees of difficulty. Please note that this software

PhpStorm Mac version
The latest (2018.2.1) professional PHP integrated development tool
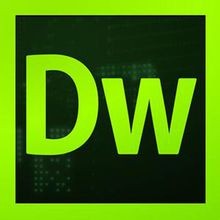
Dreamweaver CS6
Visual web development tools
