I introduce myself, I am Alfredo Riveros and I have been learning about programming for some years, I am currently studying Higher Technician in Software Development at the Higher School of Commerce - Río Tercero, and below I will describe a challenge that I came across.
As the title says, my goal was to migrate a SQLite database to MySQL, something that arose from an assignment in the database subject that I am studying.
The database I selected belongs to the game SQL Murder Mystery. This game, created to teach SQL skills in a playful way, is available at this link, where you can download the database provided by its developers.
I chose this database for its pedagogical orientation, given that although it is a game in itself, it constitutes a valuable resource for both teaching and learning concepts related to databases.
My first step in this challenge was to investigate if I could use DB Browser for SQLite to export the database in a format compatible with MySQL Workbench. Although I managed to generate a SQL script from DB Browser, importing it into Workbench presented me with numerous problems, especially syntax and data integrity, in addition to the complexity of managing such a large file.
I studied this file and tried to solve the syntax problems, and finally came to the conclusion that I should look for another approach.
My next step was to use the sqlite3 function to export a sql script through the terminal (linux).
This time the script improved a lot in syntax, but nevertheless the big problem is that one or another new problem always appeared.
With both approaches exhausted, I took a moment to reflect and evaluate other alternatives. I considered that Python could be an effective tool for this migration, given its support for both SQLite and MySQL, and began to design an algorithm to automate the process.
Then I looked for information on the topic, first verifying that it was a possible approach and collecting information to be able to design an algorithm that would allow me to meet my goal.
Now I will briefly describe the new approach with which I achieved success in my goal.
The first thing I did was document my research step by step, which led me to learn about something called object-relational mapping (ORM).
object-relational mapping (ORM) is a technique used in programming to convert data between incompatible type systems in object-oriented programming languages. In the context of databases, ORM allows you to interact with relational databases through objects instead of using SQL queries directly. This provides a more intuitive and efficient way of working with data.
In my case I used SQLAlchemy to carry out the development of the algorithm in python, and analyzing the results I found the following key points.
-
Classes like Tables:
- Each class you define (such as crime_scene_report, drivers_license, etc.) corresponds to a table in the database. The class attributes represent the columns of the table.
-
Instances as Rows:
- Each instance of a class represents a row in the corresponding table. When you query data from SQLite, you get instances of these classes, which makes working with data more intuitive and object-oriented.
-
Insertion and Update Operations:
- Using methods like merge() allows you to operate on these instances directly. You can insert new records or update existing ones without having to write SQL queries manually, which simplifies the code and makes it more readable.
-
Relationship Management:
- SQLAlchemy automatically handles relationships between tables (for example, through foreign keys) through attributes in classes, making it easier to navigate between related records.
An important thing to note during the process, after several trials and errors, is that understanding the approach and evaluating the written code is crucial, as it helps you identify places where problems can arise. After some reflection and pause, I came to the conclusion that the issue was probably related to the database structure. However, one question persisted in my mind: how is it possible for this database to work in SQLite despite having integrity problems and the various errors that appeared? The answer is simple: unlike MySQL, SQLite allows you to have tables without primary keys, which contributes to large differences in data management between both systems. This flexibility in SQLite can mask problems that, in a more restrictive environment like MySQL, would result in immediate errors.
Another difference is that MySQL has a more rigorous approach to data structure and types. For example, if you define a field as INTEGER, you will not be able to insert a value that is not a number.
The differences continue, the result of understanding them was to realize that for the approach to work there would have to be a change in the database, for this I decided to modify the tables and make sure they complied with MySQL standards , the first thing is that each one has its primary key, and make sure that both have the same data types.
I add... If you want to do the same, keep in mind that SQLite does not allow you to directly alter tables, another big difference with MySQL.
Finally having made the adaptations in the script, and in the algorithm written in python, I proceeded to execute it. The result: The game database was migrated to MySQL.
This challenge not only improved my technical skills, but also taught me the importance of understanding the differences between database management systems and how these can affect the integrity of the database.
I hope my experience migrating database from SQLite to MySQL was helpful and inspiring. Each challenge presents an opportunity to learn and grow in the world of programming.
Thanks for reading and until next time!
The above is the detailed content of Migrar de SQLite a MySQL.. For more information, please follow other related articles on the PHP Chinese website!
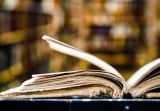
This tutorial demonstrates how to use Python to process the statistical concept of Zipf's law and demonstrates the efficiency of Python's reading and sorting large text files when processing the law. You may be wondering what the term Zipf distribution means. To understand this term, we first need to define Zipf's law. Don't worry, I'll try to simplify the instructions. Zipf's Law Zipf's law simply means: in a large natural language corpus, the most frequently occurring words appear about twice as frequently as the second frequent words, three times as the third frequent words, four times as the fourth frequent words, and so on. Let's look at an example. If you look at the Brown corpus in American English, you will notice that the most frequent word is "th
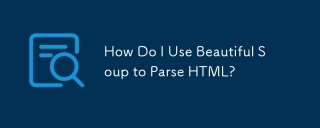
This article explains how to use Beautiful Soup, a Python library, to parse HTML. It details common methods like find(), find_all(), select(), and get_text() for data extraction, handling of diverse HTML structures and errors, and alternatives (Sel
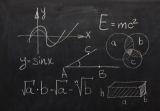
Python's statistics module provides powerful data statistical analysis capabilities to help us quickly understand the overall characteristics of data, such as biostatistics and business analysis. Instead of looking at data points one by one, just look at statistics such as mean or variance to discover trends and features in the original data that may be ignored, and compare large datasets more easily and effectively. This tutorial will explain how to calculate the mean and measure the degree of dispersion of the dataset. Unless otherwise stated, all functions in this module support the calculation of the mean() function instead of simply summing the average. Floating point numbers can also be used. import random import statistics from fracti
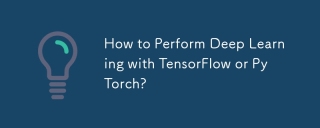
This article compares TensorFlow and PyTorch for deep learning. It details the steps involved: data preparation, model building, training, evaluation, and deployment. Key differences between the frameworks, particularly regarding computational grap
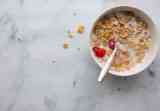
Serialization and deserialization of Python objects are key aspects of any non-trivial program. If you save something to a Python file, you do object serialization and deserialization if you read the configuration file, or if you respond to an HTTP request. In a sense, serialization and deserialization are the most boring things in the world. Who cares about all these formats and protocols? You want to persist or stream some Python objects and retrieve them in full at a later time. This is a great way to see the world on a conceptual level. However, on a practical level, the serialization scheme, format or protocol you choose may determine the speed, security, freedom of maintenance status, and other aspects of the program
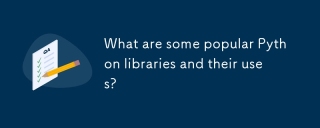
The article discusses popular Python libraries like NumPy, Pandas, Matplotlib, Scikit-learn, TensorFlow, Django, Flask, and Requests, detailing their uses in scientific computing, data analysis, visualization, machine learning, web development, and H
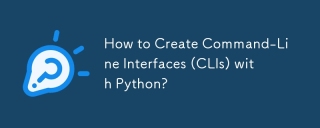
This article guides Python developers on building command-line interfaces (CLIs). It details using libraries like typer, click, and argparse, emphasizing input/output handling, and promoting user-friendly design patterns for improved CLI usability.
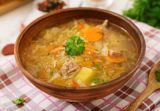
This tutorial builds upon the previous introduction to Beautiful Soup, focusing on DOM manipulation beyond simple tree navigation. We'll explore efficient search methods and techniques for modifying HTML structure. One common DOM search method is ex


Hot AI Tools

Undresser.AI Undress
AI-powered app for creating realistic nude photos

AI Clothes Remover
Online AI tool for removing clothes from photos.

Undress AI Tool
Undress images for free

Clothoff.io
AI clothes remover

AI Hentai Generator
Generate AI Hentai for free.

Hot Article

Hot Tools
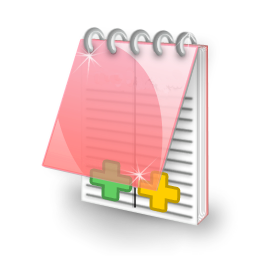
EditPlus Chinese cracked version
Small size, syntax highlighting, does not support code prompt function
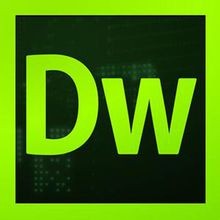
Dreamweaver CS6
Visual web development tools
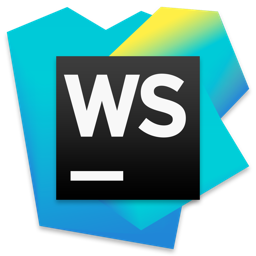
WebStorm Mac version
Useful JavaScript development tools
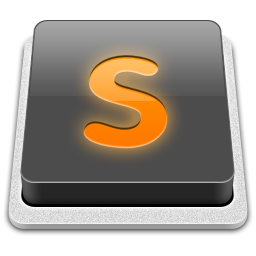
SublimeText3 Mac version
God-level code editing software (SublimeText3)

DVWA
Damn Vulnerable Web App (DVWA) is a PHP/MySQL web application that is very vulnerable. Its main goals are to be an aid for security professionals to test their skills and tools in a legal environment, to help web developers better understand the process of securing web applications, and to help teachers/students teach/learn in a classroom environment Web application security. The goal of DVWA is to practice some of the most common web vulnerabilities through a simple and straightforward interface, with varying degrees of difficulty. Please note that this software
