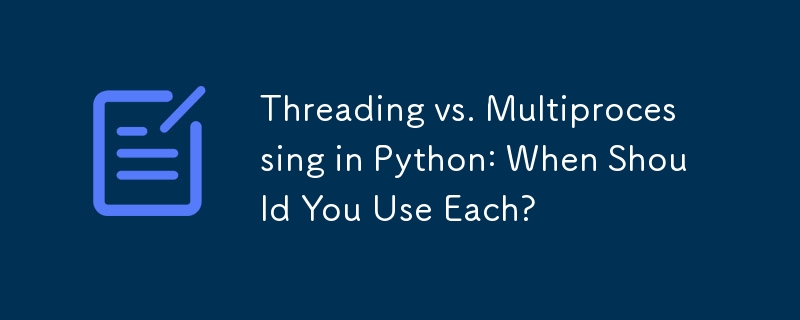
Parallel Processing in Python: Distinguishing Threading and Multiprocessing Modules
In Python, parallelizing operations is possible through both threading and multiprocessing to enhance code execution speed. However, these modules differ in their underlying mechanisms and applications.
Threading vs. Multiprocessing: A Comparison
-
Data Sharing: Threads innately share data within the same process, while processes operate independently.
-
Data Transfer: Sharing data in processes necessitates pickling, adding an overhead compared to thread communication.
-
GIL (Global Interpreter Lock): In CPython, the default Python implementation, threads are constrained by the GIL, limiting true parallelism. Processes are not subject to this restriction.
-
Resource Usage: Processes incur higher costs in creation and termination, particularly on Windows-based systems.
When to Utilize Threading vs. Multiprocessing
-
Thread Selection: Threads prove effective for concurrency tasks, such as handling network I/O or GUI events.
-
Multiprocess Selection: Use processes when CPU-bound operations are performed in pure Python to avoid GIL limitations. They also excel in scenarios where data sharing is limited or non-essential.
Job Management
Creating a queue of jobs and controlling their execution is achievable using a ThreadPoolExecutor for threads or a ProcessPoolExecutor for processes. These structures enable the submission of tasks, mapping functions to multiple inputs, and result retrieval.
Advanced Data Sharing
For non-self-contained jobs that require inter-job communication, messaging through queues is necessary. In cases where multiple jobs modify the same data structure, manual synchronization and shared-memory mechanisms are required.
Summary
- Threads facilitate data sharing by default.
- Processes isolate data, requiring pickling for data transfer.
- Processes are exempt from the GIL.
- Thread creation/destruction is more efficient than that of processes, especially in Windows environments.
- Threading module lacks certain features present in the multiprocessing module.
The above is the detailed content of Threading vs. Multiprocessing in Python: When Should You Use Each?. For more information, please follow other related articles on the PHP Chinese website!
Statement:The content of this article is voluntarily contributed by netizens, and the copyright belongs to the original author. This site does not assume corresponding legal responsibility. If you find any content suspected of plagiarism or infringement, please contact admin@php.cn