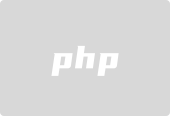
Here's a comparison between the IndexIVFFlat and IndexIVFPQ indices, along with some alternatives for their use:
Comparison: IndexIVFFlat vs. IndexIVFPQ
Characteristic |
Characteristic |
IndexIVFFlat |
IndexIVFPQ |
Storage Type |
Stores vectors in their original form. |
Utilizes product quantization (PQ) to compress vectors. |
Precision |
High precision, as it performs exact searches within cells. |
May sacrifice some precision for compression, but still provides good results. |
Search Speed |
Slower on large datasets due to exhaustive search. |
Faster, especially on large sets, thanks to reduced search space. |
Memory Usage |
Consumes more memory as it stores all vectors without compression. |
Consumes significantly less memory due to compression (up to 97% less). |
Configuration |
Simpler, only requires defining the number of cells (nlist). |
Requires defining both the number of cells (nlist) and code size (code_size). |
Training |
Needs to be trained to create cells before adding data. |
Also requires training, but the process is more complex due to quantization. |
IndexIVFFlat |
IndexIVFPQ |
Storage Type |
Stores vectors in their original form. |
Utilizes product quantization (PQ) to compress vectors. |
Precision |
High precision, as it performs exact searches within cells. |
May sacrifice some precision for compression, but still provides good results. |
Search Speed |
Slower on large datasets due to exhaustive search. |
Faster, especially on large sets, thanks to reduced search space. |
Memory Usage |
Consumes more memory as it stores all vectors without compression. |
Consumes significantly less memory due to compression (up to 97% less). |
Configuration |
Simpler, only requires defining the number of cells (nlist). |
Requires defining both the number of cells (nlist) and code size (code_size). |
Training |
Needs to be trained to create cells before adding data. |
Also requires training, but the process is more complex due to quantization. |
Pros and Cons
Pros of IndexIVFFlat
-
Precision: Provides exact results when searching within each cell.
-
Simplicity: Easy to understand and configure.
Cons of IndexIVFFlat
-
Speed: Can be very slow with large volumes of data.
-
Memory Usage: Does not optimize memory usage, which can be problematic with large datasets.
Pros of IndexIVFPQ
-
Speed: Much faster in searches due to reduced search space.
-
Memory Efficiency: Significantly reduces memory usage, allowing for handling larger datasets.
Cons of IndexIVFPQ
-
Precision: There may be a slight loss in precision due to compression.
-
Complexity: Configuration and training are more complex than in IndexIVFFlat.
Alternatives
-
IndexFlatL2
- Performs an exhaustive search without compression. Ideal for small datasets where maximum precision is required.
-
IndexPQ
- Uses only product quantization without clustering. Useful when a balance between speed and precision is needed, but clustering is not required.
-
IndexIVFScalarQuantizer
- Combines the inverted index with scalar quantization, offering a different approach to reduce memory usage and improve speed.
-
IndexIVFPQR
- A variant that combines IVF and PQ with code-based re-ranking, offering a balance between speed and improved precision.
-
Composite Indexes
- Use index_factory to create composite indices that combine multiple techniques (e.g., OPQ IVF PQ) to further optimize performance.
These alternatives allow adapting the solution to different needs in terms of precision, speed, and memory usage according to the specific case being addressed.
Citations:
[1] https://github.com/facebookresearch/faiss/wiki/Faiss-indexes/9df19586b3a75e4cb1c2fb915f2c695755a599b8
[2] https://ai.plainenglish.io/speeding-up-similarity-search-in-recommender-systems-with-faiss-advanced-concepts-part-ii-95e796a7db74?gi=ce57aff1a0c4
[3] https://www.pinecone.io/learn/series/faiss/faiss-tutorial/
[4] https://faiss.ai/cpp_api/struct/structfaiss_1_1IndexIVFFlat.html
[5] https://unfoldai.com/effortless-large-scale-image-retrieval-with-faiss-a-hands-on-tutorial/
[6] https://www.pinecone.io/learn/series/faiss/product-quantization/
[7] https://www.pinecone.io/learn/series/faiss/composite-indexes/
[8] https://github.com/facebookresearch/faiss/issues/1113
En Español, Soy Español, pero por respeto a la comunidad, pongo primero la traduccion al inglés.
Aquí tienes una comparación entre los índices IndexIVFFlat e IndexIVFPQ, junto con algunas alternativas para su uso:
Comparación: IndexIVFFlat vs. IndexIVFPQ
Característica |
Característica |
IndexIVFFlat |
IndexIVFPQ |
Tipo de Almacenamiento |
Almacena vectores en su forma original. |
Utiliza cuantización de producto (PQ) para comprimir vectores. |
Precisión |
Alta precisión, ya que realiza búsquedas exactas dentro de las celdas. |
Puede sacrificar algo de precisión por la compresión, pero aún proporciona buenos resultados. |
Velocidad de Búsqueda |
Más lento en grandes conjuntos de datos debido a la búsqueda exhaustiva. |
Más rápido, especialmente en grandes conjuntos, gracias a la reducción del espacio de búsqueda. |
Uso de Memoria |
Consume más memoria porque almacena todos los vectores sin compresión. |
Consume significativamente menos memoria debido a la compresión (hasta 97% menos). |
Configuración |
Más simple, solo requiere definir el número de celdas (nlist). |
Requiere definir tanto el número de celdas (nlist) como el tamaño del código (code_size). |
Entrenamiento |
Necesita ser entrenado para crear las celdas antes de añadir datos. |
También necesita entrenamiento, pero el proceso es más complejo debido a la cuantización. |
IndexIVFFlat |
IndexIVFPQ |
Tipo de Almacenamiento |
Almacena vectores en su forma original. |
Utiliza cuantización de producto (PQ) para comprimir vectores. |
Precisión |
Alta precisión, ya que realiza búsquedas exactas dentro de las celdas. |
Puede sacrificar algo de precisión por la compresión, pero aún proporciona buenos resultados. |
Velocidad de Búsqueda |
Más lento en grandes conjuntos de datos debido a la búsqueda exhaustiva. |
Más rápido, especialmente en grandes conjuntos, gracias a la reducción del espacio de búsqueda. |
Uso de Memoria |
Consume más memoria porque almacena todos los vectores sin compresión. |
Consume significativamente menos memoria debido a la compresión (hasta 97% menos). |
Configuración |
Más simple, solo requiere definir el número de celdas (nlist). |
Requiere definir tanto el número de celdas (nlist) como el tamaño del código (code_size). |
Entrenamiento |
Necesita ser entrenado para crear las celdas antes de añadir datos. |
También necesita entrenamiento, pero el proceso es más complejo debido a la cuantización. |
Pros and Cons
Pros of IndexIVFFlat
-
Precision: Provides exact results when searching each cell.
-
Simplicity: Easy to understand and configure.
Cons of IndexIVFFlat
-
Speed: Can be very slow with large volumes of data.
-
Memory Usage: Does not optimize memory usage, which can be a problem with large data sets.
Pros of IndexIVFPQ
-
Speed: Much faster in searches due to the reduction of the search space.
-
Memory Efficiency: Significantly reduces memory usage, allowing larger data sets to be handled.
Cons of IndexIVFPQ
-
Accuracy: There may be a slight loss in accuracy due to compression.
-
Complexity: The configuration and training are more complex than in IndexIVFFlat.
Alternatives
-
IndexFlatL2
- Performs an exhaustive search without compression. Ideal for small data sets where maximum precision is required.
-
IndexPQ
- Use only product quantization without grouping. It is useful when a balance between speed and precision is needed, but grouping is not required.
-
IndexIVFScalarQuantizer
- It combines inverted index with scalar quantization, offering a different approach to reduce memory usage and improve speed.
-
IndexIVFPQR
- A variant that combines IVF and PQ with code-based re-ranking, offering a balance between speed and improved accuracy.
-
Composite Indexes
- Use index_factory to create composite indexes that combine multiple techniques (e.g. OPQ IVF PQ) to further optimize performance.
These alternatives allow you to adapt the solution to different needs in terms of precision, speed and memory usage depending on the specific case you are addressing.
Citations:
[1] https://www.pinecone.io/learn/series/faiss/faiss-tutorial/
[2] https://www.pinecone.io/learn/series/faiss/product-quantization/
[3] https://www.pinecone.io/learn/series/faiss/composite-indexes/
[4] https://github.com/facebookresearch/faiss/wiki/Faiss-indexes/9df19586b3a75e4cb1c2fb915f2c695755a599b8
[5] https://faiss.ai/cpp_api/struct/structfaiss_1_1IndexIVFFlat.html
[6] https://pub.towardsai.net/unlocking-the-power-of-efficient-vector-search-in-rag-applications-c2e3a0c551d5?gi=71a82e3ea10e
[7] https://www.pingcap.com/article/mastering-faiss-vector-database-a-beginners-handbook/
[8] https://wangzwhu.github.io/home/file/acmmm-t-part3-ann.pdf
[9] https://github.com/alonsoir/ubiquitous-carnival/blob/main/contextual-data-faiss-IndexIVFPQ.py
[10] https://github.com/alonsoir/ubiquitous-carnival/blob/main/contextual-data-faiss-indexivfflat.py
The above is the detailed content of IndexIVFFlat y IndexIVFPQ. For more information, please follow other related articles on the PHP Chinese website!
Statement:The content of this article is voluntarily contributed by netizens, and the copyright belongs to the original author. This site does not assume corresponding legal responsibility. If you find any content suspected of plagiarism or infringement, please contact admin@php.cn