


Python is known for its simplicity and readability, but when it comes to object-oriented programming (OOP), there are some less-discussed mechanisms that are crucial for writing robust code. One such mechanism is name mangling. This article will guide you through what name mangling is, why Python uses it, and how it can help prevent name collisions in complex class hierarchies.
What Is Name Mangling
Python allows methods in classes to be overridden by subclasses. However, this can sometimes lead to name conflicts when a subclass unintentionally overrides attributes or methods from the parent class. Name mangling is a mechanism Python uses to avoid these conflicts, specifically for attributes that are meant to be private.
Name mangling in Python is a feature where the interpreter changes the names of private class properties in order to minimize the risk of them being accessed and overridden by mistake. This provides a level of privacy in class attributes, though it is not strictly enforced. However, it is not a strict enforcement.
Technical Definition
In Python, any identifier with two leading underscores (__) and no more than one trailing underscore will undergo name mangling. The interpreter transforms the name by prefixing it with the class name.
Why Does Python Use Name Mangling
In order to prevent naming conflicts, particularly in the situation when the subclasses may have their own variables that may override the variables in the parent class, Python implements name mangling. Name mangling addresses this problem.
from datetime import datetime, timedelta from time import time, sleep class Machine: def __init__(self, id): self.id = id self._started = time() def uptime(self): return time() - self._started class PetrolMachine(Machine): def __init__(self, id): super().__init__(id) self._started = datetime.now() def cost(self): duration = datetime.now() - self._started return duration/timedelta(seconds=60) *0.02 worked = PetrolMachine('12345') sleep(0.123) print(f"uptime : {worked.uptime():.2f}")
In this example, the Machine class stores an ID and records the start time using Python’s time() function. When you request uptime, it calculates the difference between the current time and the start time, which is stored as a floating-point number. The subclass PetrolMachine, however, stores the start time using datetime.now(). When we try to calculate the uptime, the program throws an error because it expects start_time to be a floating-point number, but it's now a datetime object. This naming conflict can happen when subclass attributes unintentionally override parent class attributes. Name mangling helps avoid this issue.
How Does Name Mangling Works
So how does name mangling help resolve this issue? When a class attribute is prefixed with two underscores, Python internally changes the name to include the class name as a prefix. Here's how you can modify the Machine class to avoid name conflicts using name mangling:
We can resolve the error by applying name mangling to the __started attribute in the Machine class, as shown below:
from datetime import datetime, timedelta from time import time, sleep class Machine: def __init__(self, id): self.id = id self.__started = time() def uptime(self): return time() - self.__started class PetrolMachine(Machine): def __init__(self, id): super().__init__(id) self._started = datetime.now() def cost(self): duration = datetime.now() - self._started return duration/timedelta(seconds=60) *0.02 worked = PetrolMachine('12345') sleep(0.123) print(f"uptime : {worked.uptime():.2f}")
A simple way of expressing name mangling is shown below. I have a class ClassA, which have one private_variable, which is name mangled.
class MyClass: def __init__(self): self.__private_var = "I am private" def get_private_var(self): return self.__private_var my_object = MyClass() print(my_object.get_private_var()) # This works print(my_object.__private_var)
The second print() will raise an AttributeError because the variable __private_var has been name-mangled. Internally, Python has changed the name to _MyClass__private_var, making it harder to access from outside the class.
Accessing The Mangled Name
While Python's name mangling is designed to prevent accidental access, it doesn’t enforce strict privacy. You can still access the mangled attribute by using the full mangled name, though this is discouraged. Here's how it works: my_object._MyClass__private_var
print(my_object._MyClass__private_var)
When Does Python Mangle Names
I will explain it through a simple example
class MyClass: def __init__(self): self._protected_var = "I'm protected" self.__private_var__ = "I'm not mangled"
In Python, a leading single underscore (e.g., _protected_var) indicates that the attribute is "protected" and should not be accessed directly from outside the class. However, Python does not enforce this. In contrast, names with two leading underscores (e.g., __private_var) are mangled to prevent accidental overriding. Importantly, names with double underscores on both sides (e.g., __special__) are not mangled and are reserved for special use cases such as magic methods.
優點和局限性
好處
- 有助於防止意外覆蓋子類別中的類別屬性
- 提供輕量級的隱私機制,但不會受到太多限制。
限制
- 它並不是真正的私有——其他開發人員仍然可以存取損壞的名稱,如果他們知道如何的話。
- 如果過度使用,會使偵錯和讀取程式碼變得更加複雜。
_ 儘管有這些限制,名稱修飾仍然是 Python OOP 工具包中的一個有用工具。雖然這不是嚴格的隱私保護,但它有助於防止命名衝突和意外的屬性覆蓋。了解名稱修飾將使您能夠編寫更健全、可維護的程式碼,尤其是在處理複雜的類別層次結構時。在您的專案中嘗試一下,並在下面的評論中分享您的經驗或問題! _
The above is the detailed content of Exploring Name Mangling in Python: What It Is and How It Works. For more information, please follow other related articles on the PHP Chinese website!
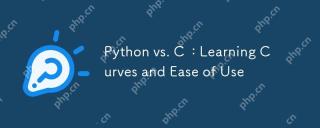
Python is easier to learn and use, while C is more powerful but complex. 1. Python syntax is concise and suitable for beginners. Dynamic typing and automatic memory management make it easy to use, but may cause runtime errors. 2.C provides low-level control and advanced features, suitable for high-performance applications, but has a high learning threshold and requires manual memory and type safety management.
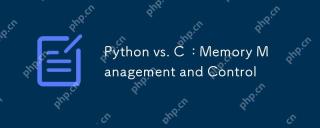
Python and C have significant differences in memory management and control. 1. Python uses automatic memory management, based on reference counting and garbage collection, simplifying the work of programmers. 2.C requires manual management of memory, providing more control but increasing complexity and error risk. Which language to choose should be based on project requirements and team technology stack.
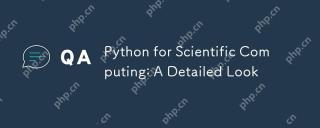
Python's applications in scientific computing include data analysis, machine learning, numerical simulation and visualization. 1.Numpy provides efficient multi-dimensional arrays and mathematical functions. 2. SciPy extends Numpy functionality and provides optimization and linear algebra tools. 3. Pandas is used for data processing and analysis. 4.Matplotlib is used to generate various graphs and visual results.
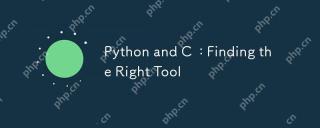
Whether to choose Python or C depends on project requirements: 1) Python is suitable for rapid development, data science, and scripting because of its concise syntax and rich libraries; 2) C is suitable for scenarios that require high performance and underlying control, such as system programming and game development, because of its compilation and manual memory management.
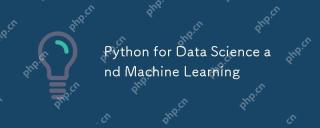
Python is widely used in data science and machine learning, mainly relying on its simplicity and a powerful library ecosystem. 1) Pandas is used for data processing and analysis, 2) Numpy provides efficient numerical calculations, and 3) Scikit-learn is used for machine learning model construction and optimization, these libraries make Python an ideal tool for data science and machine learning.
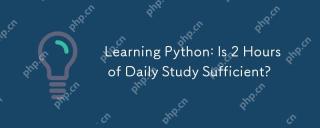
Is it enough to learn Python for two hours a day? It depends on your goals and learning methods. 1) Develop a clear learning plan, 2) Select appropriate learning resources and methods, 3) Practice and review and consolidate hands-on practice and review and consolidate, and you can gradually master the basic knowledge and advanced functions of Python during this period.
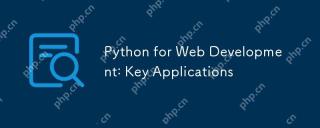
Key applications of Python in web development include the use of Django and Flask frameworks, API development, data analysis and visualization, machine learning and AI, and performance optimization. 1. Django and Flask framework: Django is suitable for rapid development of complex applications, and Flask is suitable for small or highly customized projects. 2. API development: Use Flask or DjangoRESTFramework to build RESTfulAPI. 3. Data analysis and visualization: Use Python to process data and display it through the web interface. 4. Machine Learning and AI: Python is used to build intelligent web applications. 5. Performance optimization: optimized through asynchronous programming, caching and code
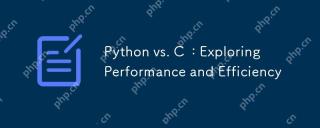
Python is better than C in development efficiency, but C is higher in execution performance. 1. Python's concise syntax and rich libraries improve development efficiency. 2.C's compilation-type characteristics and hardware control improve execution performance. When making a choice, you need to weigh the development speed and execution efficiency based on project needs.


Hot AI Tools

Undresser.AI Undress
AI-powered app for creating realistic nude photos

AI Clothes Remover
Online AI tool for removing clothes from photos.

Undress AI Tool
Undress images for free

Clothoff.io
AI clothes remover

Video Face Swap
Swap faces in any video effortlessly with our completely free AI face swap tool!

Hot Article

Hot Tools

SublimeText3 Linux new version
SublimeText3 Linux latest version

MantisBT
Mantis is an easy-to-deploy web-based defect tracking tool designed to aid in product defect tracking. It requires PHP, MySQL and a web server. Check out our demo and hosting services.

SublimeText3 Chinese version
Chinese version, very easy to use
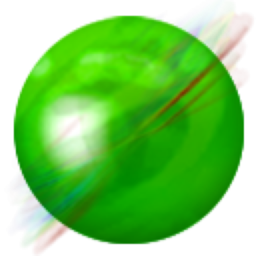
ZendStudio 13.5.1 Mac
Powerful PHP integrated development environment

Atom editor mac version download
The most popular open source editor